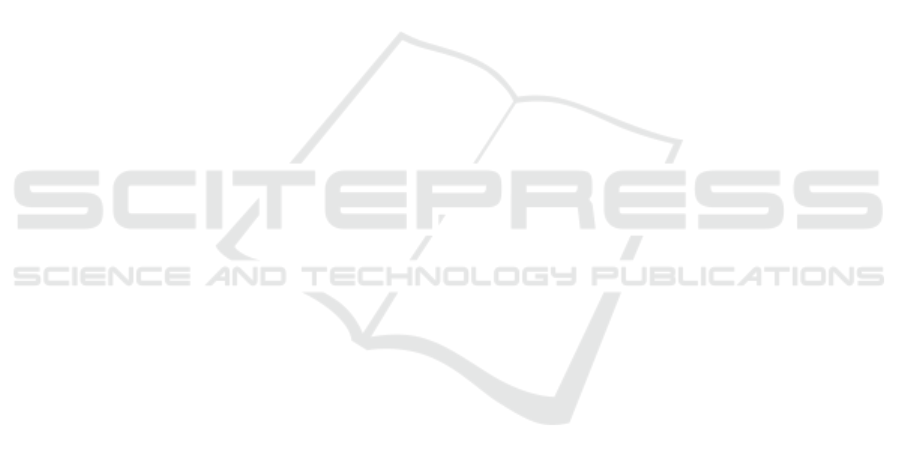
1993). In the case where all these experiments result
in high accuracy phone duration prediction, the de-
veloped system can be applied and tested in an ASR
system and employed in a real-world application for
phone segmentation. We believe that this first imple-
mentation of FL based approaches for phone segmen-
tation will be an important step for a wider deploy-
ment and development of FL approaches in the re-
search area of ASR.
ACKNOWLEDGEMENT
This work is partially supported by the Marie Curie
Initial Training Network (ITN) ESSENCE, grant
agreement no. 607062.
REFERENCES
Bezdek, J. C., Ehrlich, R., and Full, W. (1984). Fcm: The
fuzzy c-means clustering algorithm. Computers &
Geosciences, 10(2):191–203.
Chiu, S. (1996). Method and software for extracting fuzzy
classification rules by subtractive clustering. In Fuzzy
Information Processing Society, 1996. NAFIPS., 1996
Biennial Conference of the North American, pages
461–465. IEEE.
Garg, A. and Sharma, P. (2016). Survey on acoustic mod-
eling and feature extraction for speech recognition.
In Computing for Sustainable Global Development
(INDIACom), 2016 3rd International Conference on,
pages 2291–2295. IEEE.
Garofolo, J. S., Lamel, L. F., Fisher, W. M., Fiscus, J. G.,
and Pallett, D. S. (1993). Darpa timit acoustic-
phonetic continous speech corpus cd-rom. nist speech
disc 1-1.1. NASA STI/Recon Technical Report N, 93.
Giripunje, S. and Bawane, N. (2007). Anfis based emo-
tions recognision in speech. In Knowledge-based In-
telligent Information and Engineering Systems, pages
77–84. Springer.
Goubanova, O. and King, S. (2008). Bayesian networks
for phone duration prediction. Speech communication,
50(4):301–311.
Grimm, M. and Kroschel, K. (2005). Rule-based emotion
classification using acoustic features. In in Proc. Int.
Conf. on Telemedicine and Multimedia Communica-
tion. Citeseer.
Harma, A. and Pham, K. (2009). Conversation detection in
ambient telephony. In Acoustics, Speech and Signal
Processing, 2009. ICASSP 2009. IEEE International
Conference on, pages 4641–4644. IEEE.
Igras, M. and Zi
´
ołko, B. (2016). Detection of sentence
boundaries in polish based on acoustic cues. Archives
of Acoustics, 41(2):233–243.
Igras, M., Ziolko, B., and Ziolko, M. (2014). Is
phoneme length and phoneme energy useful in auto-
matic speaker recognition? In Pacific Voice Confer-
ence (PVC), 2014 XXII Annual, pages 1–5. IEEE.
Jacobi, I., Pols, L. C., Stroop, J., et al. (2005). Polder dutch:
Aspects of the/ei/-lowering in standard dutch. In In-
terspeech, number 6, pages 2877–2880. ISCA.
Jang, J.-S. (1993). Anfis: adaptive-network-based fuzzy in-
ference system. IEEE transactions on systems, man,
and cybernetics, 23(3):665–685.
Jang, J.-S. R. et al. (1991). Fuzzy modeling using general-
ized neural networks and kalman filter algorithm. In
AAAI, volume 91, pages 762–767.
Jurafsky, D. and Martin, J. H. (2014). Speech and language
processing. Pearson.
Kasparaitis, P. and Beniu
ˇ
s
˙
e, M. (2016). Automatic parame-
ters estimation of the d. klatt phoneme duration model.
Informatica, 27(3):573–586.
Lee, C. M. and Narayanan, S. (2003). Emotion recognition
using a data-driven fuzzy inference system. In Eighth
European Conference on Speech Communication and
Technology.
Mendel, J. M. (2001). Uncertain rule-based fuzzy logic sys-
tems: introduction and new directions. Prentice Hall
PTR Upper Saddle River.
Mor
´
e, J. J. (1978). The levenberg-marquardt algorithm: im-
plementation and theory. In Numerical analysis, pages
105–116. Springer.
Pols, L. C. (1983). Three-mode principal component anal-
ysis of confusion matrices, based on the identification
of dutch consonants, under various conditions of noise
and reverberation. Speech Communication, 2(4):275–
293.
Pols, L. C., Tromp, H. R., and Plomp, R. (1973). Fre-
quency analysis of dutch vowels from 50 male speak-
ers. The journal of the Acoustical Society of America,
53(4):1093–1101.
Pols, L. C., Wang, X., and ten Bosch, L. F. (1996). Mod-
elling of phone duration (using the timit database) and
its potential benefit for asr. Speech Communication,
19(2):161–176.
Rabiner, L. R. (1989). A tutorial on hidden markov models
and selected applications in speech recognition. Pro-
ceedings of the IEEE, 77(2):257–286.
Schuller, B. W., Zhang, X., and Rigoll, G. (2008). Prosodic
and spectral features within segment-based acoustic
modeling. In INTERSPEECH, pages 2370–2373.
Son, R. J. v., Binnenpoorte, D., Heuvel, H. v. d., Pols, L. C.,
et al. (2001). The ifa corpus: a phonemically seg-
mented dutch” open source” speech database.
Ten Bosch, L., Baayen, R. H., and Ernestus, M. (2006). On
speech variation and word type differentiation by ar-
ticulatory feature representations. In INTERSPEECH.
Yu, D. and Deng, L. (2014). Automatic speech recognition:
A deep learning approach. Springer.
Zadeh, L. A. (1965). Fuzzy sets. Information and control,
8(3):338–353.
Zi, B. (2009). Speech recognition of highly inflective lan-
guages.
Zi
´
ołko, B. (2015). Fuzzy precision and recall measures for
audio signals segmentation. Fuzzy Sets and Systems,
279:101–111.