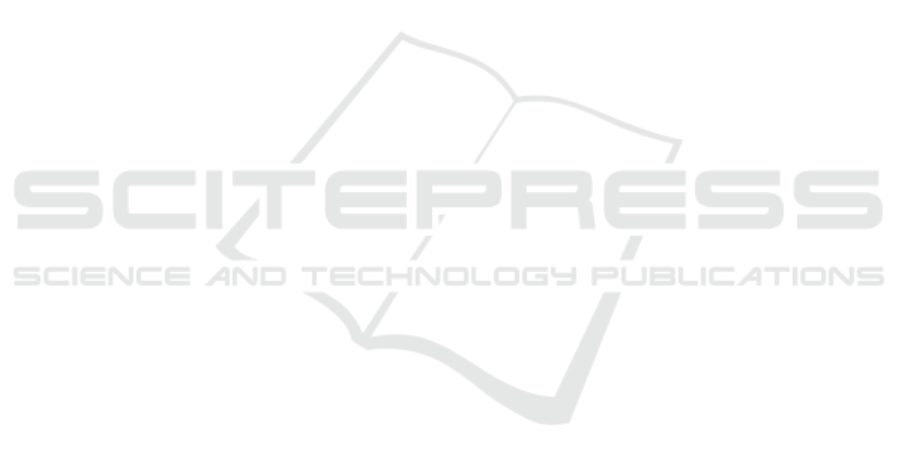
capacity is likely to emerge first in a narrowly defined
application domain.
REFERENCES
Aslam, M., Talib, R., and Majeed, H. (2014). A review on
the role of domain driven data mining. International
Journal of Computer Science and Mobile Computing,
3(5):708–712.
Bernstein, A., Provost, F., and Hill, S. (2005). Toward
intelligent assistance for a data mining process: An
ontology-based approach for cost-sensitive classifica-
tion. IEEE Transactions on Knowledge and Data En-
gineering, 17(4):503–518.
Brachman, R. J. and Anand, T. (1996). The process of
knowledge discovery in databases. In Advances in
Knowledge Discovery and Data Mining, pages 37–57.
American Association for Artificial Intelligence.
B
¨
uchner, A. G., Mulvenna, M. D., Anand, S. S., and
Hughes, J. G. (1999). An internet-enabled knowledge
discovery process. In Proceedings of the 9th Interna-
tional Database Conference, pages 13–27.
Cao, L. (2010). Domain-driven data mining: Challenges
and prospects. IEEE Transactions on Knowledge and
Data Engineering, 22(6):755–769.
Dou, D., Wang, H., and Liu, H. (2015). Semantic data min-
ing: A survey of ontology-based approaches. In Pro-
ceedings of the 9th IEEE International Conference on
Semantic Computing, pages 244–251.
Dumontier, M., Baker, C. J. O., Baran, J., Callahan, A.,
Chepelev, L., Cruz-Toledo, J., Del Rio, N. R., Duck,
G., Furlong, L. I., Keath, N., Klassen, D., McCusker,
J. P., Queralt-Rosinach, N., Samwald, M., Villanueva-
Rosales, N., Wilkinson, M. D., and Hoehndorf, R.
(2014). The Semanticscience Integrated Ontology
(SIO) for biomedical research and knowledge discov-
ery. Journal of Biomedical Semantics, 5(1):14.
Esteves, D., Mendes, P. N., Moussallem, D., Duarte, J. C.,
Zaveri, A., and Lehmann, J. (2016). MEX interfaces:
Automating machine learning metadata generation. In
Proceedings of the 12th International Conference on
Semantic Systems, pages 17–24.
Fawcett, T. (2016). Mining the quantified self: Personal
knowledge discovery as a challenge for data science.
Big Data, 3(4):249–266.
Fayyad, U., Piatetsky-Shapiro, G., and Smyth, P. (1996).
The KDD process for extracting useful knowledge
from volumes of data. Communications of the ACM,
39(11):27–34.
Federal Big Data Commission (2012). Demystifying big
data: A practical guide to transforming the business
of government. Technical report, TechAmerica Foun-
dation.
Keet, C. M., Ławrynowicz, A., d’Amato, C., Kalousis, A.,
Nguyen, P., Palma, R., Stevens, R., and Hilario, M.
(2015). The Data Mining OPtimization Ontology.
Web Semantics: Science, Services and Agents on the
World Wide Web, 32:43–53.
Kietz, J.-U., Serban, F., Fischer, S., and Bernstein, A.
(2014). “Semantics inside!” but let’s not tell the data
miners: Intelligent support for data mining. In The
Semantic Web: Trends and Challenges. 11th Interna-
tional Conference, ESWC 2014, Proceedings, pages
706–720.
Kuznetsov, S. O. and Poelmans, J. (2013). Knowledge rep-
resentation and processing with formal concept anal-
ysis. Wiley Interdisciplinary Reviews: Data Mining
and Knowledge Discovery, 3(3):200–215.
Lemke, C., Budka, M., and Gabrys, B. (2015). Metalearn-
ing: a survey of trends and technologies. Artificial
Intelligence Review, 44(1):117–130.
Moyle, S. and Jorge, A. (2001). RAMSYS - a methodology
for supporting rapid remote collaborative data mining
projects. In ECML/PKKD01 Workshop on Integrating
Aspects of Data Mining, Decision Support and Meta-
Learning, pages 20–31.
Nguyen, P., Hilario, M., and Kalousis, A. (2014). Using
meta-mining to support data mining workflow plan-
ning and optimization. Journal of Artificial Intelli-
gence Research, 51:605–644.
Panov, P., Soldatova, L., and D
ˇ
zeroski, S. (2014). Ontology
of core data mining entities. Data Mining and Knowl-
edge Discovery, 28(5–6):1222–1265.
Poelmans, J., Ignatov, D. I., Kuznetsov, S. O., and Dedene,
G. (2013a). Formal concept analysis in knowledge
processing: A survey on applications. Expert Systems
with Applications, 40(16):6538–6560.
Poelmans, J., Kuznetsov, S. O., Ignatov, D. I., and Dedene,
G. (2013b). Formal concept analysis in knowledge
processing: A survey on models and techniques. Ex-
pert Systems with Applications, 4(16):6601–6623.
Ristoski, P. and Paulheim, H. (2016). Semantic Web in data
mining and knowledge discovery: A comprehensive
survey. Web Semantics: Science, Services and Agents
on the World Wide Web, 36:1–22.
Serban, F., Vanschoren, J., Kietz, J.-U., and Bernstein, A.
(2013). A survey of intelligent assistants for data anal-
ysis. ACM Computing Surveys, 45(3):article 31.
Tuovinen, L. (2014). From machine learning to learning
with machines: Remodeling the knowledge discovery
process. PhD thesis, University of Oulu, Finland.
Tuovinen, L. (2016). A conceptual model of actors and in-
teractions for the knowledge discovery process. In
Proceedings of the 8th International Joint Confer-
ence on Knowledge Discovery, Knowledge Engineer-
ing and Knowledge Management – Volume 1: KDIR,
pages 240–248.
ˇ
Z
´
akov
´
a, M., K
ˇ
remen, P.,
ˇ
Zelezn
´
y, F., and Lavra
ˇ
c, N.
(2011). Automating knowledge discovery workflow
composition through ontology-based planning. IEEE
Transactions on Automation Science and Engineer-
ing, 8(2):253–264.
Wen, D., Zhang, X., Liu, X., and Lei, J. (2017). Eval-
uating the consistency of current mainstream wear-
able devices in health monitoring: A comparison un-
der free-living conditions. Journal of Medical Internet
Research, 19(3):e68.
Wirth, R. and Hipp, J. (2000). CRISP-DM: Towards a stan-
dard process model for data mining. In Proceedings of
the 4th International Conference on the Practical Ap-
plications of Knowledge Discovery and Data Mining,
pages 29–39.