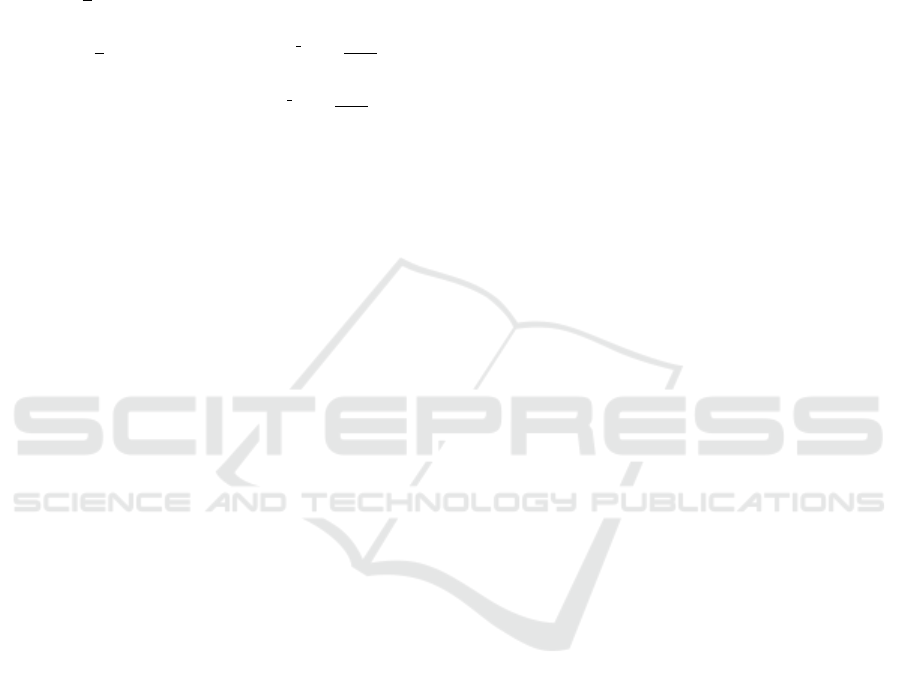
Figure 5 shows the fuzzy p-value at the signifi-
cance level δ = 0.05.
We defuzzify this fuzzy p-value by the signed dis-
tance method using the equation (36) and we obtain
the following distance:
d( ˜p,
˜
0) =
1
2
Z
1
0
2 ×(P
θ
L
(T ≥
˜
t
R
α
) + 2 ×P
θ
R
(T ≥
˜
t
L
α
))dα
=
1
2
Z
1
0
(2 ×
Z
∞
0.9+8.1×α
(2π)
−
1
2
exp(
−u
2
2
)du +
2 ×
Z
∞
17.1−8.1×α
(2π)
−
1
2
exp(
−u
2
2
)du)dα
= 0.0123989.
The defuzzified p-value (0.0123989) is smaller
than the significance level (0.05), then the decision
will be to reject the null hypothesis at the level δ =
0.05.
6 CONCLUSION
In this work, we presented a hypothesis testing proce-
dure when both data and hypotheses are fuzzy. We
introduced as well a fuzzy p-value with its α-cuts.
We discussed after the defuzzification of this fuzzy p-
value by the so-called ”signed distance method”. We
finally proposed numerical examples of one-sided and
two-sided tests, in addition to a small comparison be-
tween different null and alternative hypotheses with
the same hypothetical sample at the same significance
level. To conclude, despite the fact that the defuzzi-
fication step reduces the amount of information con-
tained in a fuzzy p-value, we thought that in many
cases defuzziying these p-values with the signed dis-
tance can be of a high relevance. In addition, since
testing hypotheses on linguistic variables is in most
cases complicated and not feasible in classical statis-
tics, proposing such an approach to deal with fuzzi-
ness and obtaining a p-value deserves merit in deci-
sion making. Indeed, we can see the defuzzied p-
value as an ”informal indicator” of rejecting or not a
given null hypothesis. For further researches, we will
be interested in testing the method with many other
statistical distributions.
REFERENCES
Berkachy, R. and Donz
´
e, L. (2016). Individual and global
assessments with signed distance defuzzification, and
characteristics of the output distributions based on an
empirical analysis. In Proceedings of the 8th Inter-
national Joint Conference on Computational Intelli-
gence - Volume 1: FCTA,, pages 75 – 82.
Berkachy, R. and Donz
´
e, L. (2017). Defuzzification of
a fuzzy hypothesis decision by the signed distance
method. In Proceedings of the 61st World Statistics
Congress, Marrakech, Morocco.
Filzmoser, P. and Viertl, R. (2004). Testing hypotheses with
fuzzy data: The fuzzy p-value. Metrika, Springer-
Verlag, 59:21–29.
Grzegorzewski, P. (2000). Testing statistical hypotheses
with vague data. Fuzzy Sets and Systems, 112(3):501
– 510.
Grzegorzewski, P. (2001). Fuzzy tests - defuzzification and
randomization. Fuzzy Sets and Systems, 118(3):437 –
446.
Lin, L. and Lee, H. (2009). Fuzzy assessment method on
sampling survey analysis. Expert Systems with Appli-
cations, 36(3):5955 – 5961.
Lin, L. and Lee, H. (2010). Fuzzy assessment for sampling
survey defuzzification by signed distance method. Ex-
pert Systems with Applications, 37(12):7852 – 7857.
Neyman, J. and Pearson, E. S. (1933). The testing of sta-
tistical hypotheses in relation to probabilities a priori.
Mathematical Proceedings of the Cambridge Philo-
sophical Society, 29(04):492–510.
Parchami, A., Taheri, S. M., and Mashinchi, M. (2010).
Fuzzy p-value in testing fuzzy hypotheses with crisp
data. Stat Pap, 51(1):209–226.
Torabi, H. and Mirhosseini, S. M. The most powerful tests
for fuzzy hypotheses testing with vague data.
Viertl, R. (2011). Statistical methods for fuzzy data. John
Wiley and Sons, Ltd.
Yao, J. and Wu, K. (2000). Ranking fuzzy numbers
based on decomposition principle and signed distance.
Fuzzy sets and Systems, 116(2):275 – 288.
Zadeh, L. (1965). Fuzzy sets. Information and Control,
8(3):338 – 353.
Zadeh, L. (1996). Fuzzy Sets, Fuzzy Logic, and Fuzzy Sys-
tems: Selected Papers of Lotfi A. Zadeh, G. J. Klir and
B. Yuan, Eds., Advances in Fuzzy Systems – Applica-
tions and Theory, volume 6. World Scientific.
Zimmermann, H. (2010). Fuzzy set theory. Wiley Interdis-
ciplinary Reviews: Computational Statistics, 2(3):317
– 332.