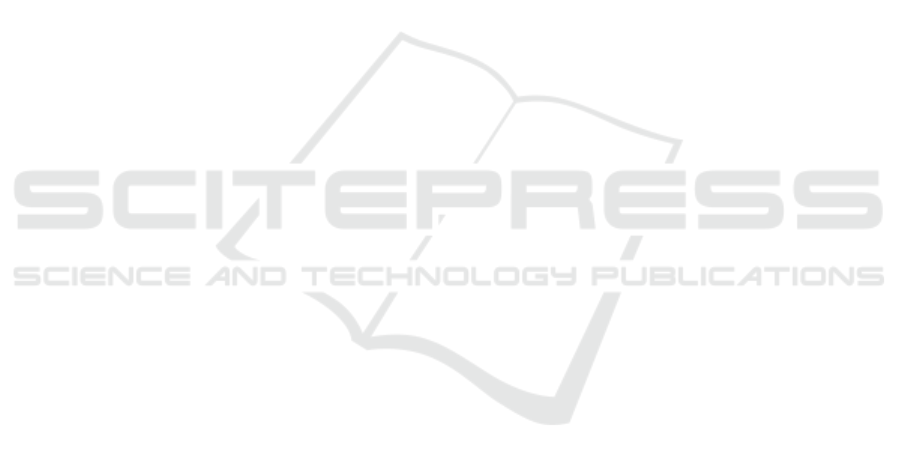
solutions, optimal under the compliance with the tar-
get illumination level, and the consumption of elec-
tric power. However, for designing an accurate in-
terior illumination and able to not be glare nor inad-
equate, it is needed to optimize multiple constraints,
often conflicting, which make hard the use of classical
computational methods. Thus, a multi-objective ge-
netic algorithm has been developed for interior light-
ing design, with the main aim to (1) optimize the level
of luminous intensity, and (2) maximizing the energy
saving. Moreover, a clustering of solutions approach
has been also developed, in order to reduce the search
space and the Pareto front, helping, then, the deci-
sion maker in the choice and selection of the more
appropriate illumination. In combination to NSGA-
II, the proposed computational tool is based also on
a 3D graphic software, that is Blender, for providing
a rendering engine for direct illumination and repro-
duce the architectural space to be lighted. Two dif-
ferent case studies have been considered in order to
evaluate the accuracy, and efficiency of the illumina-
tion produced, based on different complex shapes of
the architectural interiors (irregular and non-convex),
which make harder the design of an uniform illumina-
tion distribution. Finally, from the analysis of the sev-
eral experiments performed, the presented algorithm
has showed to be a suitable and effective tool for in-
terior lighting design in a variety of different environ-
ments.
REFERENCES
Bechikh, S., Kessentini, M., Said, L. B., and Gh
´
edira, K.
(2015). Preference incorporation in evolutionary mul-
tiobjective optimization: A survey of the state-of-the-
art. Advances in Computers, 98:141–207.
Bertoldi, P., Hirl, B., and Labanca, N. (2012). Energy effi-
ciency status report. Technical report, European Com-
mission – Institute for Energy and Transport.
Caldas, L. (2016). Painting with light: An interactive evo-
lutionary system for daylighting design. Building and
Environment. doi: 10.1016/j.buildenv.2016.07.023.
Chiu, S. L. (1994). Fuzzy model identification based on
cluster estimation. Journal of Intelligent & fuzzy sys-
tems, 2:267–278.
Cuttle, C. (2015). Lighting design: a perception-based ap-
proach. Routledge, New York.
Daenzer, S., Montgomery, K., Dillmann, R., and Un-
terhinninghofen, R. (2007). Real-time smoke and
bleeding simulation in virtual surgery. In Westwood,
J. D., Haluck, R. S., Hoffman, H. M., Mogel, G. T.,
Phillips, R., Robb, R. A., and Vosburgh, K. G., ed-
itors, Medicine Meets Virtual Reality, pages 94–99.
IOS Press, Amsterdam.
Deb, K., Agrawal, S., Pratap, A., and Meyarivan, T. (2000).
A fast elitist non-dominated sorting genetic algorithm
for multi-objective optimization: NSGA-II. In In-
ternational Conference on Parallel Problem Solving
From Nature, pages 849–858.
Fortin, F.-A., De Rainville, F.-M., Gardner, M.-A., Parizeau,
M., and Gagn
´
e, C. (2012). DEAP: Evolutionary algo-
rithms made easy. Journal of Machine Learning Re-
search, 13:2171–2175.
Gordon, G. (2014). Interior lighting for designers. John
Wiley, New York.
Jaimes, A. L. and Coello, C. A. C. (2013). Interactive ap-
proaches applied to multiobjective evolutionary algo-
rithms. In Doumpos, M. and Grigoroudis, E., editors,
Multicriteria decision aid and artificial intelligence:
Theory and applications, pages 191–207. John Wiley,
New York.
Livingston, J. (2015). Designing with light: the art, science,
and practice of architectural lighting design. John Wi-
ley, New York.
Palmer, S. (1999). Vision Science – Photons to Phe-
nomenology. MIT Press, Cambridge (MA).
Plebe, A. and Grasso, G. (2016). Simulating fire outbreaks
in industrial plants. In Blender Conference, Amster-
dam.
Sansoni, P., Farini, A., and Mercatelli, L., editors (2015).
Sustainable Indoor Lighting. Springer-Verlag, Berlin.
Wunderlich, C.-H. (2003). Light and economy: an essay
about the economy of prehistoric and ancient lamps.
In Chranovski, L., editor, Lychnological news, pages
251–264. LychnoServices, Hauterive (Suisse).
Zio, E. and Bazzo, R. (2010). Multiobjective optimization
of the inspection intervals of a nuclear safety system:
A clustering-based framework for reducing the pareto
front. Annals of Nuclear Energy, 37:798–812.
Zio, E. and Bazzo, R. (2012). A comparison of methods
for selecting preferred solutions in multiobjective de-
cision making. In Kahraman, C., editor, Computa-
tional Intelligence Systems in Industrial Engineering
With Recent Theory and Applications, pages 23–43.
Atlantis Press, Paris.