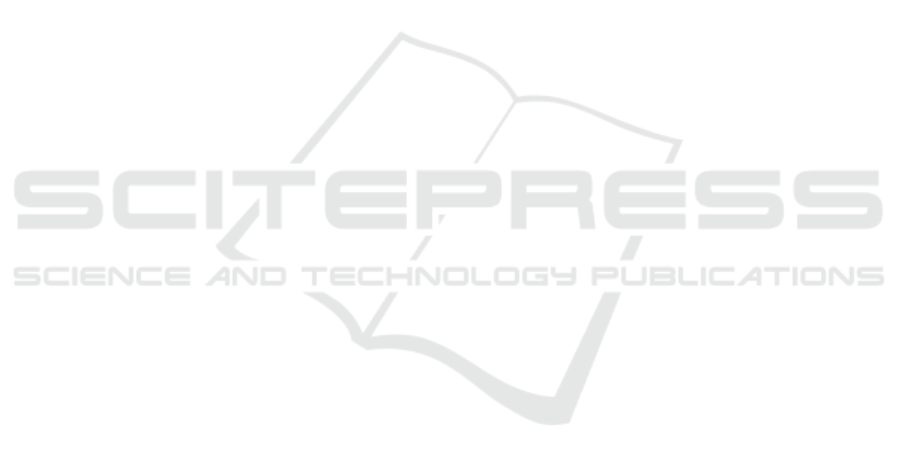
proach, Kab-NS reduced MSE results by 50%, 25%,
18%, 8%, 10% and 10% under 20dB, 10dB, 5dB,
3dB, 2dB and 0 dB, respectively for noise-free train-
ing scenarios (left side of the Fig. 7).
After noise-free training procedures were com-
pleted, training was repeated by using noisy time se-
ries (t = 1 to t = 700), and the remaining 300 points
from the same noisy sets were used in testing. As be-
fore, the standard deviation of the Gaussian input FSs
was adjusted to the level used in the corresponding
noise level each time. When the noisy training and
noisy testing (right side of the Fig.7) cases are scru-
tinised for Kab-NS technique, a similar tendency of
improvement (25%,18%,14%,11%,10% and 5%) can
be recognised compared to the standard composition
method.
3.4.2 Experiment 2: The Non-corresponding
Standard Deviations of Gaussian Input
Fuzzy Sets
These experiments were then modified to examine the
behaviour of all approaches under unknown noise lev-
els. In these versions of the experiment, the same
procedures from Experiment 1 (‘noise-free training,
noisy testing’ and ‘noisy training, noisy testing’) were
repeated. However, it was assumed that the noise lev-
els in time series are unknown and hence the standard
deviation of Gaussian input sets was not adjusted un-
der each different noise level. Rather the noise in the
input sets was fixed to two different levels.
Experiment 2a: The standard deviation was fixed
to be 5dB noise (0.161) and all procedures from Ex-
periment 1 were implemented without adjusting input
FSs. In comparison with the standard approach, Kab-
NS reduced MSE results by 46%, 35%, 18%, 5%,
5% and 0% under 20dB, 10dB, 5dB, 3dB, 2dB and 0
dB, respectively for noise-free training scenarios. As
it shows similar performance improvement (except
0 dB case) in noisy training (43%,29%,14%,8%,3%
and -11%). All the noise-free and noisy training pro-
cedures results can be seen in Fig.8.
Experiment 2b: This time the standard devia-
tion was fixed to be 0dB noise (0.286) and again
all operations were repeated without adjusting stan-
dard deviations of input FSs. In comparison with
the standard approach, Kab-NS reduced MSE re-
sults by 26%, 25%, 20%, 17%, 14% and 10% un-
der 20dB, 10dB, 5dB, 3dB, 2dB and 0 dB, respec-
tively for noise-free training scenarios. As it shows
similar performance improvement in noisy training
(25%,29%,25%,18%,17% and 5%). All the noise-
free and noisy training procedures results can be seen
in Fig.8. The experimental result can be seen in Fig.
9.
3.5 Discussion
When the Experiment 1 (Fig. 7) is analysed, it can
be seen that the novel similarity measure (Kab-NS)
outperforms both the standard and centroid (cen-NS)
techniques under each level of noises regardless of
noise-free or noisy training conditions. Comparing
the MSE results from jac-NS and Kab-NS, we can
see that the results for both techniques are the same
under almost all conditions.
As mentioned before, in Experiment 2a the stan-
dard deviations were fixed at the 5 dB noise level. In
this experiment (Fig. 8), Kab-NS has better MSE re-
sults than the standard and cen-NS techniques nine
out of twelve cases and the rest of cases have the
same MSE results except the extreme noisy training
case. In comparison to jac-NS, while the Kab-NS has
either higher or the same MSE results in less noisy
conditions and as the noise level is increased Kab-NS
shows the better results.
When the standard deviations were fixed as 0 dB
noise, in the Experiment 2b, in all the noise-free
and noisy training instances (Fig. 9), Kab-NS out-
performed the standard technique and cen-NS under
all 12 scenarios (both noise-free and noisy training).
However it should be mentioned that the jac-NS has
the lowest MSE results among all four variants in 10
cases out of 12.
To recapitulate, when all the 36 cases are exam-
ined, Kab-NS outperforms standard and cen-NS tech-
niques in 34 cases out of 36. Both jac-NS and Kab-
NS have moderately similar average MSE results. It
is worthwhile noting that the goal of this work is not
specifically to achieve the best performance in appli-
cations which use different approaches for generating
firing levels but to study and compare the various ap-
proaches to try to discover the most reliable approach
to be used under different conditions.
This is particularly relevant in situations in which
the noise level cannot easily be known in advance,
which is often the case in the real-world. Situations
might include when the FLS must be designed and
fixed in advance of implementation in the real world,
or in situations where the noise level itself is varying
in an unpredictable manner.
It should be noted that we have implemented
the experiments with different discretisation lev-
els/approaches and we found that the results are sen-
sitive to way the discretisations is applied.