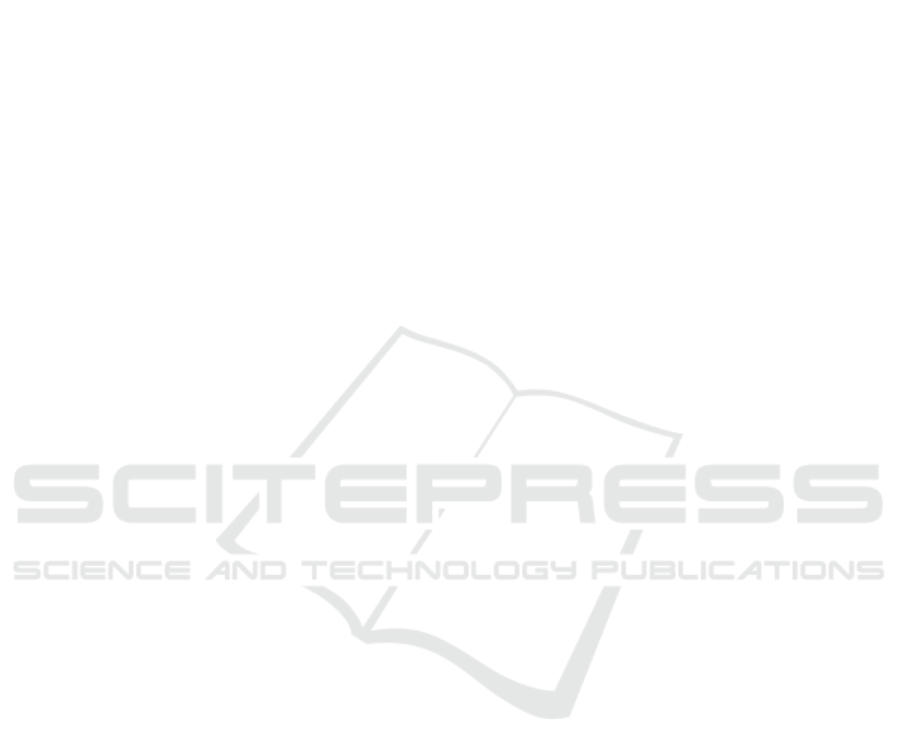
Real-Time Barcode Detection and Classification using Deep Learning
Daniel Kold Hansen, Kamal Nasrollahi, Christoffer B. Rasmusen and Thomas B. Moeslund
Aalborg University, Rendsburggade 14, 9000 Aalborg, Denmark
Keywords:
Deep Learning, Barcode detection, Barcode Rotation.
Abstract:
Barcodes, in their different forms, can be found on almost any packages available in the market. Detecting
and then decoding of barcodes have therefore great applications. We describe how to adapt the state-of-the-
art deep learning-based detector of You Only Look Once (YOLO) for the purpose of detecting barcodes in a
fast and reliable way. The detector is capable of detecting both 1D and QR barcodes. The detector achieves
state-of-the-art results on the benchmark dataset of Muenster BarcodeDB with a detection rate of 0.991. The
developed system can also find the rotation of both the 1D and QR barcodes, which gives the opportunity of
rotating the detection accordingly which is shown to benefit the decoding process in a positive way. Both the
detection and the rotation prediction shows real-time performance.
1 INTRODUCTION
Barcodes are an integrated part of the world today and
are used in many different contexts ranging from the
local supermarket to the use in advertising. Barcodes
can be split into two different main categories, 1D and
2D barcodes. The best known 1D barcode types are
probably the EAN an UPC type which is mainly used
for labelling consumer products at the local supermar-
ket. A very known and popular 2D barcode is the QR
barcode. The QR barcode is for example used in mar-
keting where it acts as a link between the printed and
digital media, by redirecting people to additional in-
formation, competitions, social media sites, etc. To
decode barcodes, several solutions exist ranging from
laser scanners to camera based devices. Traditional
solutions such as the laser scanner do not provide the
opportunity of decoding 2D barcodes, to do that cam-
era based scanners are needed. A popular camera
based scanner is the smartphone which allows the user
to scan virtually any type of barcode. The smartphone
does, however, requires a certain amount of guidance
from the user, and are usually only capable of decod-
ing one barcode at the time. To optimise this process,
it could be desirable to locate barcodes in an image
and thereby be able to decode multiple barcodes at
the time and require less guidance from a user.
2 RELATED WORK
There have been proposed a lot of different solu-
tions to various problems regarding locating barcodes
throughout the years. One of the first papers trying to
locate barcodes is Mu
˜
niz et al.(Muniz et al., 1999),
where an application to process Spanish medicine
prescription automatically is developed. This is a
very early example of locating barcodes, but as the
technology has expanded through the years, more and
more opportunities have arisen.
The introduction of mobile phones with cameras
has inspired several papers with algorithms trying to
find barcodes using the camera of a mobile phone.
Ohbuchi et al.(Ohbuchi et al., 2004) from 2004 im-
plements a mobile application able to locate both QR
and EAN-codes by corner detection and spiral search,
and rectifies the barcode in the end as well. In 2008
Wachenfeld et al.(Wachenfeld et al., 2008) propose a
method for recognition of 1D barcodes where decod-
ing is used as a tool for finding the barcode. Both
Ohbuchi and Wachenfeld rely very much on the user
pointing the camera at the barcode and thereby using
the phone very much like a laser scanner.
In more recent papers there is more focus on mak-
ing algorithms for barcode detection that rely as little
as possible on the user centring and aligning the cam-
era with the barcode. There are several approaches to
the problem, some are relying on simple morphology
operation like(Katona and Ny
´
ul, 2013) and the im-
proved version(Katona and Ny
´
ul, 2012) by Katona et
Hansen D., Nasrollahi K., B. Rasmusen C. and Moeslund T.
Real-Time Barcode Detection and Classification using Deep Learning.
DOI: 10.5220/0006508203210327
In Proceedings of the 9th International Joint Conference on Computational Intelligence (IJCCI 2017), pages 321-327
ISBN: 978-989-758-274-5
Copyright
c
2017 by SCITEPRESS – Science and Technology Publications, Lda. All rights reserved