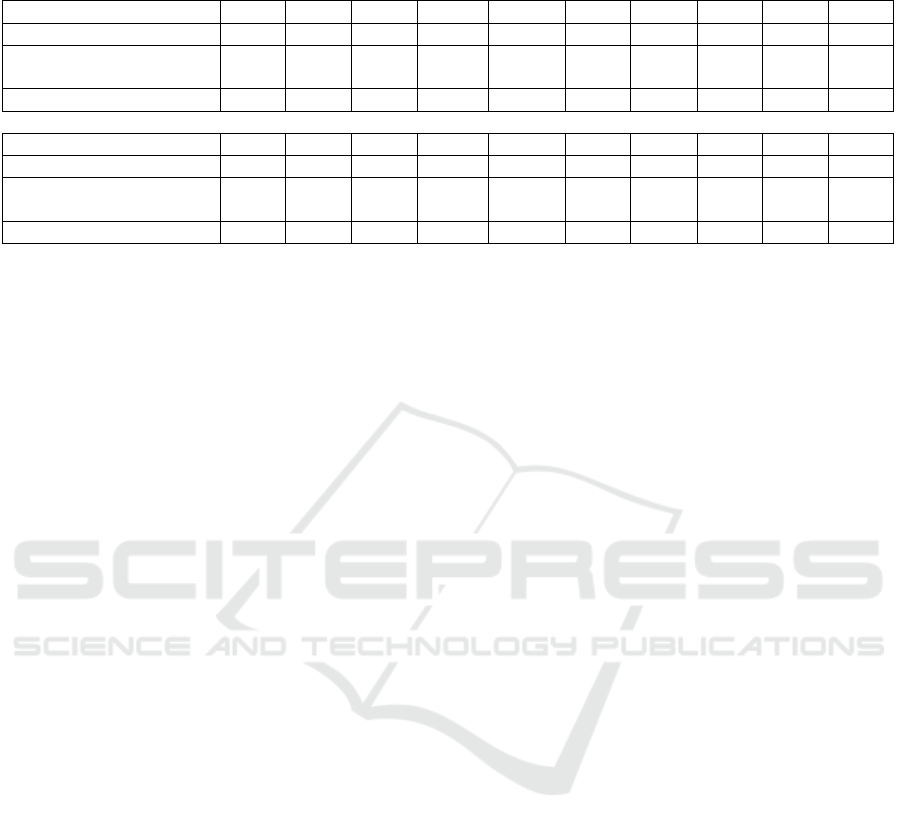
Table 11: Results for the individual classes in the 07 test set. Shown are the results for the baseline model and Image
Quality
WAvg
/ Resolution
Avgbase
. Additionally the difference between the two methods are presented for a given class.
Model aero bike bird boat bottle bus car cat chair cow
Baseline (Dai et al., 2016) 80.53 84.59 79.89 71.52 67.54 87.22 87.59 87.98 65.15 87.11
Image Quality
WAvg
/
Resolution
Avgbase
80.57 85.45 81.02 72.51 68.69 88.00 87.38 89.13 67.27 86.57
Difference +0.04 +0.86 +1.13 +0.99 +1.15 +0.78 -0.21 +1.15 +2.12 -0.54
Model table dog horse mbike person plant sheep sofa train tv
Baseline (Dai et al., 2016) 73.66 88.61 87.83 83.21 79.87 54.60 84.07 80.03 83.60 77.17
Image Quality
WAvg
/
Resolution
Avgbase
72.21 88.75 87.04 84.15 80.17 53.97 83.56 80.11 86.62 78.64
Difference -1.45 +0.14 -0.79 +0.95 +0.30 -0.63 -0.51 +0.08 +3.02 +1.47
REFERENCES
Bosse, S., Maniry, D., M
¨
uller, K., Wiegand, T., and Samek,
W. (2016). Deep neural networks for no-reference
and full-reference image quality assessment. CoRR,
abs/1612.01697.
COCO, M. (2017). Ms coco detections leaderboard.
Dai, J., Li, Y., He, K., and Sun, J. (2016). R-FCN: object de-
tection via region-based fully convolutional networks.
CoRR, abs/1605.06409.
Everingham, M., Van Gool, L., Williams, C. K. I., Winn,
J., and Zisserman, A. (2010). The pascal visual ob-
ject classes (voc) challenge. International Journal of
Computer Vision, 88(2):303–338.
Girshick, R. (2015). Fast R-CNN. In Proceedings of the
International Conference on Computer Vision (ICCV).
Girshick, R., Donahue, J., Darrell, T., and Malik, J. (2014).
Rich feature hierarchies for accurate object detec-
tion and semantic segmentation. In Proceedings of
the IEEE conference on computer vision and pattern
recognition, pages 580–587.
He, K., Zhang, X., Ren, S., and Sun, J. (2015). Deep
residual learning for image recognition. CoRR,
abs/1512.03385.
Huang, J., Rathod, V., Sun, C., Zhu, M., Korattikara, A.,
Fathi, A., Fischer, I., Wojna, Z., Song, Y., Guadar-
rama, S., and Murphy, K. (2016). Speed/accuracy
trade-offs for modern convolutional object detectors.
CoRR, abs/1611.10012.
Jia, Y., Shelhamer, E., Donahue, J., Karayev, S., Long, J.,
Girshick, R., Guadarrama, S., and Darrell, T. (2014).
Caffe: Convolutional architecture for fast feature em-
bedding. arXiv preprint arXiv:1408.5093.
Krizhevsky, A., Sutskever, I., and Hinton, G. E. (2012).
Imagenet classification with deep convolutional neu-
ral networks. In Pereira, F., Burges, C. J. C., Bottou,
L., and Weinberger, K. Q., editors, Advances in Neu-
ral Information Processing Systems 25, pages 1097–
1105. Curran Associates, Inc.
Larson, E. and Chandler, D. M. (2009). Consumer subjec-
tive image quality database.
Li, Y., Qi, H., Dai, J., Ji, X., and Wei, Y. (2016). Fully
convolutional instance-aware semantic segmentation.
CoRR, abs/1611.07709.
Lin, T.-Y., Maire, M., Belongie, S., Hays, J., Perona, P.,
Ramanan, D., Doll
´
ar, P., and Zitnick, C. L. (2014).
Microsoft COCO: Common Objects in Context, pages
740–755. Springer International Publishing, Cham.
Ponomarenko, N., Ieremeiev, O., Lukin, V., Egiazarian, K.,
Jin, L., Astola, J., Vozel, B., Chehdi, K., Carli, M.,
Battisti, F., and Kuo, C. C. J. (2013). Color image
database tid2013: Peculiarities and preliminary re-
sults. In European Workshop on Visual Information
Processing (EUVIP), pages 106–111.
Redmon, J. and Farhadi, A. (2016). YOLO9000: better,
faster, stronger. CoRR, abs/1612.08242.
Ren, S., He, K., Girshick, R., and Sun, J. (2015). Faster R-
CNN: Towards real-time object detection with region
proposal networks. In Neural Information Processing
Systems (NIPS).
Russakovsky, O., Deng, J., Su, H., Krause, J., Satheesh,
S., Ma, S., Huang, Z., Karpathy, A., Khosla, A.,
Bernstein, M., Berg, A. C., and Fei-Fei, L. (2015).
ImageNet Large Scale Visual Recognition Challenge.
International Journal of Computer Vision (IJCV),
115(3):211–252.
Schroff, F. (2009). Semantic Image Segmentation and Web-
supervised Visual Learning. University of Oxford.
Sheikh, H. R., Sabir, M. F., and Bovik, A. C. Live image
quality assessment database release 2.
Sheikh, H. R., Sabir, M. F., and Bovik, A. C. (2006). A sta-
tistical evaluation of recent full reference image qual-
ity assessment algorithms. IEEE Transactions on Im-
age Processing, 15(11):3440–3451.
Shrivastava, A., Gupta, A., and Girshick, R. B. (2016).
Training region-based object detectors with online
hard example mining. CoRR, abs/1604.03540.
Simonyan, K. and Zisserman, A. (2015). Very deep convo-
lutional networks for large-scale image recognition. In
ICLR.
Szegedy, C., Ioffe, S., and Vanhoucke, V. (2016). Inception-
v4, inception-resnet and the impact of residual con-
nections on learning. CoRR, abs/1602.07261.
Tokui, S., Oono, K., Hido, S., and Clayton, J. (2015).
Chainer: a next-generation open source framework for