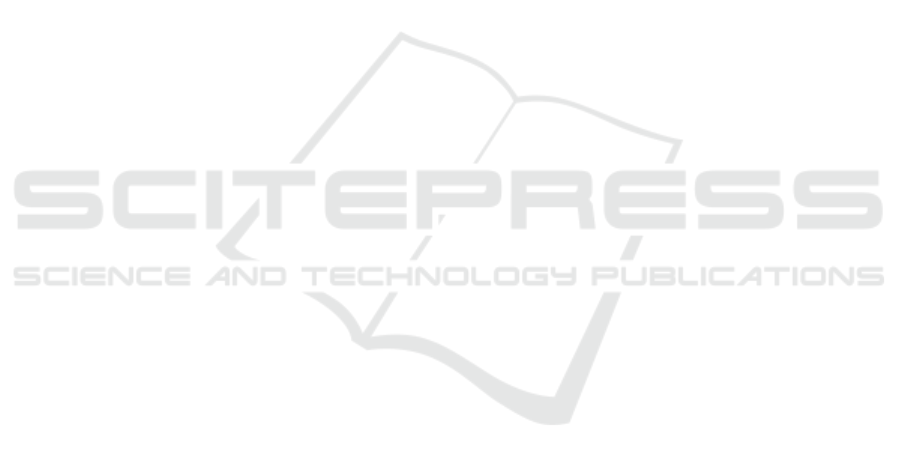
This method is an offline approach for eliciting pri-
vacy heuristics from regrettable online self-disclosure
experiences. Basically, the input of the method are
the experiences that users have reported themselves
(to the development team of IAS for instance), or the
outcome of an empirical research (e.g. questionnaires
or face to face interviews). This approach is effective
for building a baseline of heuristics prior to the exe-
cution of the system. However, eliciting new entries
of the PHDB requires the execution of this process
which can be expensive and inefficient in terms of the
resources and time needed to conduct interviews and
process the outcome of them. One way to overcome
this issue is to consider deleted posts with private in-
formation as potential sources of heuristics (D
´
ıaz Fer-
reyra et al., 2017b). This is, to use such posts as the
input of a machine learning engine for the automatic
derivation of privacy heuristics at runtime. This way,
the PHDB can be updated with new heuristics with-
out having to execute offline iterations of the PHeDer
method.
7 OUTLOOK AND CONCLUSION
Adaptive awareness technologies seem to be promis-
ing approaches for empowering the users of SNSs in
making wiser and more informed decisions, as to pro-
tect them from the risks of over-sharing private infor-
mation. We believe that this is not a minor issue that
should be taken seriously into consideration by Inter-
net service providers, multilateral organizations and
policy makers. We have used the example of HWLs in
cigarettes packages as a motivating scenario for work-
ing towards a more privacy aware social environment
in SNSs. Certainly, this topic will be part of a in-depth
and intense debate in the future. Therefore we hope
this paper will offer a more clarifying view on this is-
sue and serve as an instrument for the development of
more effective solutions.
ACKNOWLEDGEMENTS
This work was supported by the Deutsche
Forschungsgemeinschaft (DFG) under grant No.
GRK 2167, Research Training Group ”User-Centred
Social Media”.
REFERENCES
Calikli, G., Law, M., Bandara, A. K., Russo, A., Dick-
ens, L., Price, B. A., Stuart, A., Levine, M., and Nu-
seibeh, B. (2016). Privacy Dynamics: Learning Pri-
vacy Norms for Social Software. In Proceedings of the
11th International Symposium on Software Engineer-
ing for Adaptive and Self-Managing Systems, pages
47–56. ACM.
D
´
ıaz Ferreyra, N. E., Meis, R., and Heisel, M. (2017a). On-
line Self-disclosure: From Users’ Regrets to Instruc-
tional Awareness. In Proceedings of the IFIP Interna-
tional Cross-Domain Conference (CD-MAKE). Ac-
capted for publication.
D
´
ıaz Ferreyra, N. E., Meis, R., and Heisel, M. (2017b). To-
wards an ILP Approach for Learning Privacy Heuris-
tics From Users’ Regrets. In Proceedings of the 4th
European Network Intelligence Conference (ENIC).
Accapted for publication.
D
´
ıaz Ferreyra, N. E., Sch
¨
awel, J., Heisel, M., and Meske, C.
(2016). Addressing Self-disclosure in Social Media:
An Instructional Awareness Approach. In Proceed-
ings of the 2nd ACS/IEEE International Workshop on
Online Social Networks Technologies (OSNT). AC-
S/IEEE.
Fang, L. and LeFevre, K. (2010). Privacy wizards for social
networking sites. In Proceedings of the 19th Inter-
national Conference on World Wide Web, WWW ’10,
pages 351–360, New York, NY, USA. ACM.
Ghazinour, K., Matwin, S., and Sokolova, M. (2013).
YourPrivacyProtector: A Recommender System for
Privacy Settings in Social Networks. International
Journal of Security, Privacy and Trust Management
(IJSPTM), 2(4).
Hiilamo, H., Crosbie, E., and Glantz, S. A. (2014). The
evolution of health warning labels on cigarette packs:
the role of precedents, and tobacco industry strategies
to block diffusion. Tobacco control, 23(1):e2–e2.
Keller, D. (2017). The right tools: Europe’s intermediary li-
ability laws and the 2016 general data protection regu-
lation. Technical report, Stanford Law School Center
for Internet and Society.
Mitrovic, A. and Ohlsson, S. (2006). Constraint-based
knowledge representation for individualized instruc-
tion. Computer Science and Information Systems
(ComSIS) Journal, 13(1).
Regulation, G. D. P. (2016). Regulation (EU) 2016/679
of the European Parliament and of the Council of 27
April 2016 on the protection of natural persons with
regard to the processing of personal data and on the
free movement of such data, and repealing Directive
95/46/EC (General Data Protection Regulation). Offi-
cial Journal of the European Union (OJ), 59:1–88.
Stark, L. (2016). The Emotional Context of Information
Privacy. The Information Society, 32(1):14–27.
Wang, Y., Leon, P. G., Scott, K., Chen, X., Acquisti, A., and
Cranor, L. F. (2013). Privacy Nudges for Social Me-
dia: An Exploratory Facebook Study. In Proceedings
of the 22nd International Conference on World Wide
Web, pages 763–770. ACM.
Ziegeldorf, J. H., Henze, M., Hummen, R., and Wehrle,
K. (2015). Comparison-based privacy: nudging pri-
vacy in social media (position paper). In International
Workshop on Data Privacy Management, pages 226–
234. Springer.