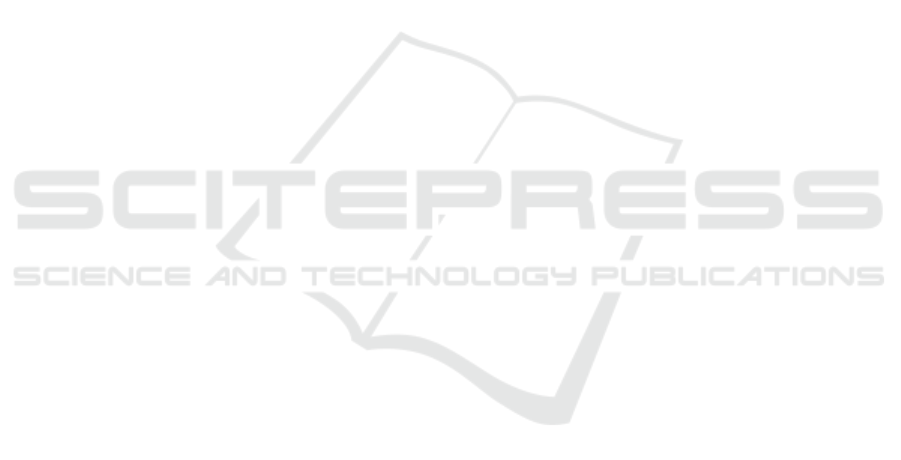
was achieved by double-level isoline map-based IG
features and SVM with sigmoid kernel. The best F-
score (96.7%) was achieved by double-level isoline
map-based chi-squared features and SVM with RBF
kernel.
4 CONCLUSIONS
In this paper a new automated heart disease diagnos-
tics approach based on supervised machine learning
with LV myocardium feature representation utilizing
isoline map statistics was presented. Experiments
provided in this paper demonstrate both intuitiveness
of presented feature model and its usability for the
considered task.
The scope of constructed feature model applica-
tion is not limited to contrast-enhanced CT images
analysis and medical imaging purposes. The pro-
posed approach can be also utilized in other texture
analysis or fixed intensity range blobs detection tasks.
For more applicability and fully automated anal-
ysis, automated LV myocardium segmentation algo-
rithm for contrast-enhanced CT images is being de-
veloped. Further evaluation of the proposed auto-
mated diagnostics algorithm on larger datasets is war-
ranted.
ACKNOWLEDGEMENTS
The work was supported by the Grant of President
of Russian Federation for young scientists No. MK-
1896.2017.9 (contract No. 14.W01.17.1896-MK).
REFERENCES
Afshin, M., Ben Ayed, I., Punithakumar, K., Law, M., Is-
lam, A., Goela, A., Ross, I., Peters, T., and Li, S.
(2011). Assessment of regional myocardial function
via statistical features in MR images. Medical Im-
age Computing and Computer-Assisted Intervention–
MICCAI 2011, pages 107–114.
Antunes, S., Esposito, A., Palmisanov, A., Colantoni, C.,
de Cobelli, F., and Del Maschio, A. (2016). Char-
acterization of normal and scarred myocardium based
on texture analysis of cardiac computed tomography
images. In Engineering in Medicine and Biology So-
ciety (EMBC), 2016 IEEE 38th Annual International
Conference of the, pages 4161–4164. IEEE.
Boser, B. E., Guyon, I. M., and Vapnik, V. N. (1992). A
training algorithm for optimal margin classifiers. In
Proceedings of the fifth annual workshop on Compu-
tational learning theory, pages 144–152. ACM.
Breiman, L. (2001). Random forests. Machine learning,
45(1):5–32.
Breiman, L., Friedman, J., Olshen, R., and Stone, C. (1984).
Classification and Regression Trees. Wadsworth &
brooks/cole advanced books & software. Pacific Cal-
ifornia.
Cerqueira, M. D., Weissman, N. J., Dilsizian, V., Jacobs,
A. K., Kaul, S., Laskey, W. K., Pennell, D. J., Rum-
berger, J. A., Ryan, T., Verani, M. S., et al. (2002).
Standardized myocardial segmentation and nomencla-
ture for tomographic imaging of the heart. Circula-
tion, 105(4):539–542.
Greenwood, P. E. and Nikulin, M. S. (1996). A guide to
chi-squared testing, volume 280. John Wiley & Sons.
Hall, M. A. (1999). Correlation-based feature selection for
machine learning. University of Waikato Hamilton.
Nichols, M., Townsend, N., Scarborough, P., and Rayner,
M. (2014). Cardiovascular disease in Europe 2014:
epidemiological update. European Heart Journal,
35(42):2950–2959.
Senyukova, O. V. (2014). Segmentation of blurred objects
by classification of isolabel contours. Pattern Recog-
nition, 47(12):3881–3889.
Wang, Z., Salah, M. B., Gu, B., Islam, A., Goela, A., and
Li, S. (2014). Direct estimation of cardiac biven-
tricular volumes with an adapted Bayesian formula-
tion. IEEE Transactions on Biomedical Engineering,
61(4):1251–1260.
Wong, K., Tee, M., Chen, M., Bluemke, D. A., Summers,
R. M., and Yao, J. (2016). Regional infarction iden-
tification from cardiac CT images: a computer-aided
biomechanical approach. Int J Comput Assist Radiol
Surg, 11(9):1573–1583.
Wong, K. C., Tee, M., Chen, M., Bluemke, D. A., Summers,
R. M., and Yao, J. (2015). Computer-aided infarction
identification from cardiac CT images: a biomechan-
ical approach with SVM. In 18th International Con-
ference on Medical Image Computing and Computer-
Assisted Intervention, MICCAI 2015, pages 144–151.
Springer Verlag.
Xu, C., Xu, L., Gao, Z., Zhang, H., Zhang, Y., Du, X., Zhao,
S., Ghista, D., Li, S., et al. (2017). Direct detection
of pixel-level myocardial infarction areas via a deep-
learning algorithm. arXiv preprint arXiv:1706.03182.