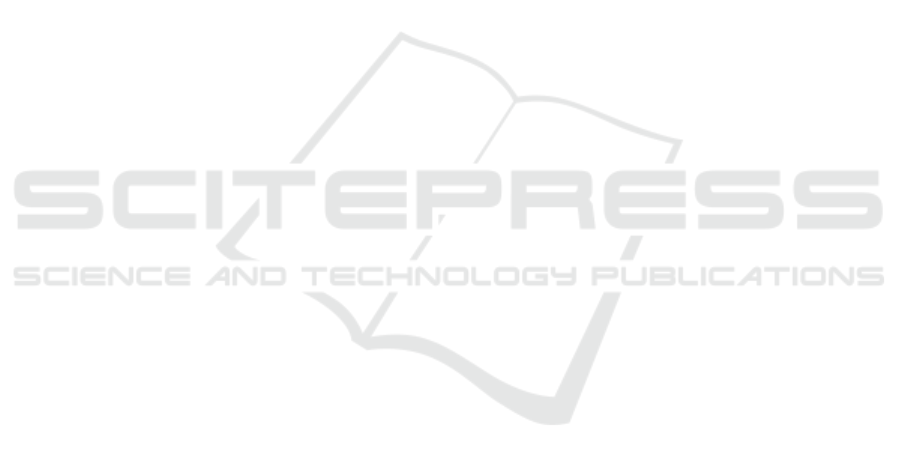
decrease the cost of electricity used. The result of sav-
ing energy can be seen clearly in subplot B of figure
5. When there is no room occupancy, the amount of
energy consumed becomes zero. As a result,the total
cost of electricity decreases to $3 as shown in subplot
D of figure 5.
5 CONCLUSIONS
We have designed a neuro-fuzzy HVAC control sys-
tem for regulating room temperature. Our controller
achieves a higher indoor environment quality by bal-
ancing thermal comfort and energy consumption. The
underlying model of the proposed controller utilizes
a Sugeno-style fuzzy inference system with two sen-
sory inputs: one for temperature and another for mo-
tion. It outputs a signal that represents the mode of
the air conditioner and the compressor speed for each
mode. The testing of the controller showed that the air
conditioner of the controlled HVAC system turns off
automatically 10 minutes after the last detected mo-
tion of room occupants. Accordingly, simulations of
the cost levels and energy consumption were shown
when the room was empty as justified through the ab-
sence of occupants’ motion.
For the future, we aim at expanding the number
of environmental factors to be considered compared
with only one variable, that is, the room temperature
in the current analysis. Also, we shall deploy the con-
troller to monitor a whole house rather than merely
one room.
ACKNOWLEDGEMENTS
The authors would like to thank the anonymous re-
viewers for their valuable time and helpful comments.
REFERENCES
Afram, A. and Janabi-Sharifi, F. (2014). Theory and ap-
plications of hvac control systems–a review of model
predictive control (mpc). Building and Environment,
72:343–355.
˚
Astr
¨
om, K. J. and H
¨
agglund, T. (1984). Automatic tuning
of simple regulators with specifications on phase and
amplitude margins. Automatica, 20(5):645–651.
Bi, Q., Cai, W.-J., Wang, Q.-G., Hang, C.-C., Lee, E.-
L., Sun, Y., Liu, K.-D., Zhang, Y., and Zou, B.
(2000). Advanced controller auto-tuning and its appli-
cation in hvac systems. Control Engineering Practice,
8(6):633–644.
Caldo, R. B. (2015). FLC-based indoor air quality assess-
ment for ashrae standard conformance. In Industrial
Engineering, Management Science and Applications
2015, pages 711–718. Springer.
Cavcar, M. (2000). The international standard atmosphere
(isa). Anadolu University, Turkey, 30:1–7.
Collotta, M., Messineo, A., Nicolosi, G., and Pau, G.
(2014). A dynamic fuzzy controller to meet thermal
comfort by using neural network forecasted parame-
ters as the input. Energies, 7(8):4727–4756.
Dash, S. K., Mohanty, G., and Mohanty, A. (2012). Intelli-
gent air conditioning system using fuzzy logic. In-
ternational Journal of Scientific & Engineering Re-
search, 3(12):1–6.
Gl
¨
uge, S., Hamid, O. H., and Wendemuth, A. (2010). A
simple recurrent network for implicit learning of tem-
poral sequences. Cognitive Computation, 2(4):265–
271.
Hamid, O. H. (2015). A model-based markovian context-
dependent reinforcement learning approach for neu-
robiologically plausible transfer of experience. In-
ternational Journal of Hybrid Intelligent Systems,
12(2):119–129.
Hamid, O. H., Wendemuth, A., and Braun, J. (2010).
Temporal context and conditional associative learn-
ing. BMC neuroscience, 11(1):45.
Jaynes, E. T. (2003). Probability theory: The logic of sci-
ence. Cambridge university press.
Keller, J. M., Fogel, D. B., and Liu, D. (2016). Fundamen-
tals of computational intelligence. Wiley-IEEE Press.
Kobersi, I. S., Finaev, V. I., Almasani, S. A., and Abdo,
K. W. A. (2013). Control of the heating system
with fuzzy logic. World Applied Sciences Journal,
23(11):1441–1447.
Air Conditioning Systems (2017). Air Conditioning Calcu-
lations. (online accessed: 2017, January 27).
Canadian Centre for Occupational Health and Safety
(2017). Thermal Comfort for Office Work. (2017,
February 17).
Engineering 360 Powered by IEEE GlobalSpec (2017).
HVAC - Ventilation. (online accessed: 2017, January
27).
McCormick, B. W. (1995). Aerodynamics, aeronautics, and
flight mechanics, volume 2. Wiley New York.
Mirinejad, H., Welch, K. C., and Spicer, L. (2012). A re-
view of intelligent control techniques in hvac systems.
In Energytech, 2012 IEEE, pages 1–5. IEEE.
Mohindru, P. and Sharma, G. (2015). Simulation perfor-
mance of pid and fuzzy logic controller for higher or-
der system. Simulation, 1(7).
Nowak, M. and Urbaniak, A. (2016). Application of predic-
tive control algorithms for thermal comfort and energy
saving in the classroom. In Carpathian Control Con-
ference (ICCC), 2016 17th International, pages 527–
532. IEEE.
Salsbury, T. I. (2005). A survey of control technologies in
the building automation industry. IFAC Proceedings
Volumes, 38(1):90–100.