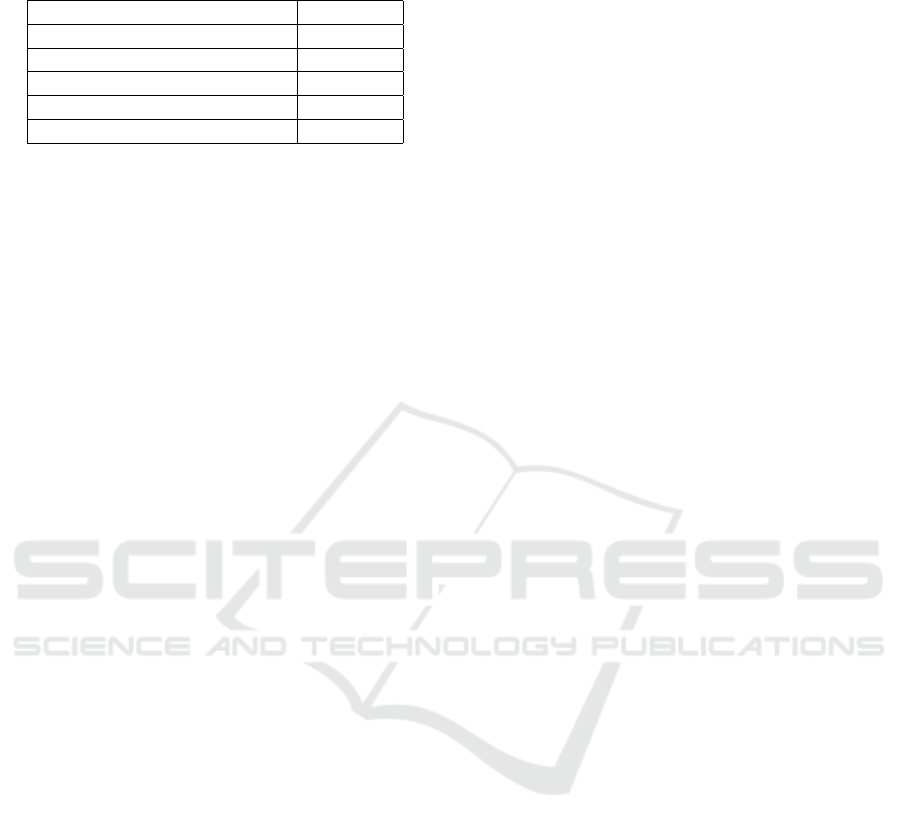
Table 2: Comparative Results on IEMOCAP using FAP-
based features.
Classification approach Accuracy
RBM-SVM (Shah et al., 2014) 60.71%
PFA-SVM (Kim et al., 2013) 65%
DBN-SVM (Kim et al., 2013) 68%
EP-SVM (Mower et al., 2011) 71%
Default ARTMAP 72.2%
tor profiled for that particular emotion (Mower
et al., 2011)
These results are for four class (neutral, happy,
anger, as sadness) classification as those researches
used four class classification. It is evident from the
table that our approach gives the best results for FAP-
based classifier on IEMOCAP data set. Furthermore,
(Mower et al., 2011) and (Kim et al., 2013) used both
facial and vocal features. However, since they had
a similar set of facial features and they tested their
approaches on IEMOCAP, we used their results for
comparison as well.
5 CONCLUSION AND FUTURE
WORK
In this paper, we proposed facial emotion recognition
using a default ARTMAP classifier. The proposed
classification scheme along with the FAP-based fea-
tures was shown to be an effective facial emotion clas-
sifier in the presence of speech. The results show that
our approach also yielded better results than the exist-
ing state-of-the-art on IEMOCAP database.
In future, we plan to integrate our emotion recog-
nition with real time perception. Furthermore, we
also intend to investigate other configurations of
ARTMAP involving distributed training along with
the distributed testing used in this paper.
ACKNOWLEDGEMENT
This work was supported by the ICT R&D program
of MSIP/IITP. [2016-0-00563, Research on Adaptive
Machine Learning Technology Development for In-
telligent Autonomous Digital Companion]
REFERENCES
Amis, G. P. and Carpenter, G. A. (2007). Default artmap
2. In 2007 International Joint Conference on Neural
Networks, pages 777–782.
Busso, C., Bulut, M., Lee, C.-C., Kazemzadeh, A., Mower,
E., Kim, S., Chang, J. N., Lee, S., and Narayanan,
S. S. (2008). Iemocap: interactive emotional dyadic
motion capture database. Language Resources and
Evaluation, 42(4):335.
Carpenter, G. A. and Gaddam, S. C. (2010). Biased art: A
neural architecture that shifts attention toward previ-
ously disregarded features following an incorrect pre-
diction. Neural Networks, 23(3):435 – 451.
Carpenter, G. A., Grossberg, S., Markuzon, N., Reynolds,
J. H., and Rosen, D. B. (1992). Fuzzy artmap: A neu-
ral network architecture for incremental supervised
learning of analog multidimensional maps. Trans.
Neur. Netw., 3(5):698–713.
Carpenter, G. A., Grossberg, S., and Reynolds, J. H.
(1991a). Artmap: Supervised real-time learn-
ing and classification of nonstationary data by a
self-organizing neural network. Neural Networks,
4(5):565 – 588.
Carpenter, G. A., Grossberg, S., and Rosen, D. B. (1991b).
Fuzzy art: Fast stable learning and categorization of
analog patterns by an adaptive resonance system. Neu-
ral Networks, 4(6):759 – 771.
Hirota, K. and Dong, F. (2008). Development of mascot
robot system in nedo project. In Intelligent Systems,
2008. IS ’08. 4th International IEEE Conference, vol-
ume 1, pages 1–38–1–44.
Kim, Y., Lee, H., and Provost, E. M. (2013). Deep learn-
ing for robust feature generation in audio-visual emo-
tion recognition. In IEEE International Conference on
Acoustics, Speech and Signal Processing (ICASSP).
Li, H., Lin, Z., Shen, X., Brandt, J., and Hua, G. (2015a).
A convolutional neural network cascade for face de-
tection. In The IEEE Conference on Computer Vision
and Pattern Recognition (CVPR).
Li, W., Li, M., Su, Z., and Zhu, Z. (2015b). A deep-learning
approach to facial expression recognition with candid
images. In Machine Vision Applications (MVA), 2015
14th IAPR International Conference on, pages 279–
282.
Liu, P., Han, S., Meng, Z., and Tong, Y. (2014). Facial
expression recognition via a boosted deep belief net-
work. In The IEEE Conference on Computer Vision
and Pattern Recognition (CVPR).
Liu, Z.-T., Min, W., Dan-Yun, L., Lue-Feng, C., Fang-Yan,
D., Yoichi, Y., and Kaoru, H. (2013). Communi-
cation atmosphere in humans and robots interaction
based on the concept of fuzzy atmosfield generated
by emotional states of humans and robots. Journal of
Automation, Mobile Robotics and Intelligent Systems,
7(2):52–63.
Mariooryad, S. and Busso, C. (2016). Facial expression
recognition in the presence of speech using blind lex-
ical compensation. IEEE Transactions on Affective
Computing, 7(4):346–359.
Mower, E., Mataric, M. J., and Narayanan, S. (2011). A
framework for automatic human emotion classifica-
tion using emotion profiles. IEEE Transactions on Au-
dio, Speech, and Language Processing, 19(5):1057–
1070.