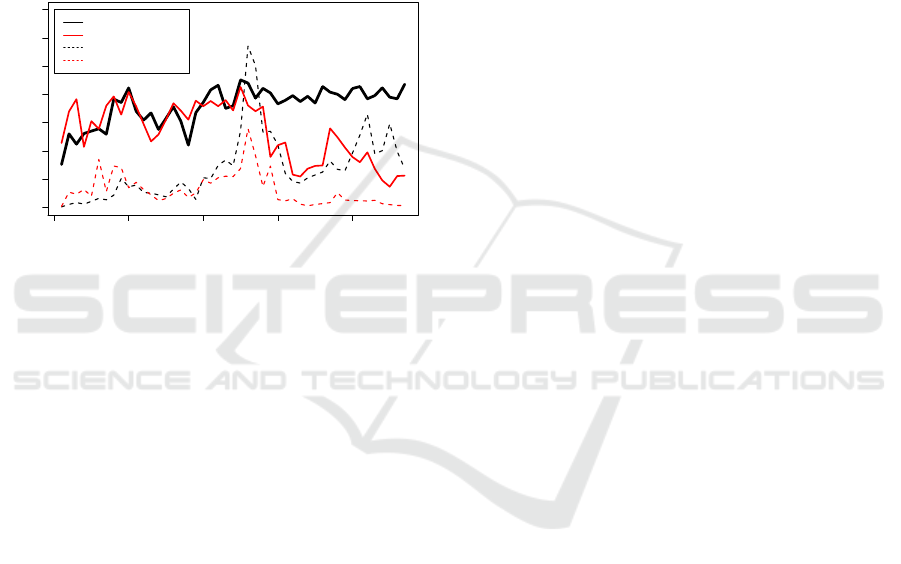
can’t be explained just by frequency. Especially for
the word brexit, we notice continuously a high volatil-
ity value irrespective of its frequency. In the time
immediately around the referendum the frequency of
both words have their highest peaks, which is caused
by the amount of articles released at that time span.
However, we measure almost the same amount of
contextual change for brexit already in February just
after Cameron announced the referendum, indicating
at that stage a high degree of public controversy. Us-
ing the MinMax-algorithm, we can compare a word’s
volatility with its frequency over time as well as 2
words that differ in frequency.
0.00 0.10 0.20 0.30
0 10 20 30 40
context volatility
weeks
brexit−cv
cameron−cv
brexit−frequency
cameron−frequency
Figure 4: Context volatility for the words brexit, cameron
and their frequencies.
7 CONCLUSION
In this work we presented an evaluation and prac-
tical application of the context volatility methodol-
ogy. We’ve shown a solution for evaluating con-
textual change measurements, especially the con-
text volatility measure, by creating a synthetic data
set, which can simulate various cases of contextual
change. Also, we introduced alternative algorithms,
which are superior to the baseline context volatility
algorithm, when facing problems on real diachronic
corpora (co-occurrence gaps). In the evaluation and
application the MinMax-algorithms shows its robust-
ness when competing with other methods by the abil-
ity to use the gap information directly and handle
the dynamics in the number of co-occurrences for a
word. With those improvements in the calculation of
context-volatility, we believe that the measure is able
to produce new results in various applications.
REFERENCES
Blei, D. M. and Lafferty, J. D. (2006). Dynamic topic mod-
els. In Proceedings of the 23rd international confer-
ence on Machine learning, pages 113–120.
Bordag, S. (2008). A comparison of co-occurrence and sim-
ilarity measures as simulations of context. In Com-
putational Linguistics and Intelligent Text Processing,
pages 52–63. Springer.
Fernndez-Silva, S., Freixa, J., and Cabr, M. T. (2011).
Picturing short-period diachronic phenomena in spe-
cialised corpora: A textual terminology description
of the dynamics of knowledge in space technologies.
Terminology, 17(1):134–156.
Hamilton, W. L., Leskovec, J., and Jurafsky, D. (2016). Di-
achronic word embeddings reveal statistical laws of
semantic change. arXiv preprint arXiv:1605.09096.
Heyer, G., Holz, F., and Teresniak, S. (2009). Change
of Topics over Time and Tracking Topics by Their
Change of Meaning. In KDIR ’09: Proc. of Int. Conf.
on Knowledge Discovery and Information Retrieval.
Heyer, G., Kantner, C., Niekler, A., Overbeck, M., and
Wiedemann, G. (2016). Modeling the dynamics of
domain specific terminology in diachronic corpora.
In TERM BASES AND LINGUISTIC LINKED OPEN
DATA, pages 75 – 90.
Heyer, G., Keim, D., Teresniak, S., and Oelke, D. (2011).
Interaktive explorative Suche in groen Dokumentbest-
nden. Datenbank-Spektrum, 11(3):195–206.
Holz, F. and Teresniak, S. (2010). Towards Automatic De-
tection and Tracking of Topic Change. In Computa-
tional Linguistics and Intelligent Text Processing, vol-
ume 6008, pages 327–339. Springer, Berlin, Heidel-
berg.
J
¨
ahnichen, P. (2016). Time Dynamic Topic Models.
J
¨
ahnichen, P., Oesterling, P., Liebmann, T., Heyer, G.,
Kuras, C., and Scheuermann, G. (2015). Exploratory
Search Through Interactive Visualization of Topic
Models. Digital Humanities Quarterly.
Jatowt, A. and Duh, K. (2014). A framework for analyzing
semantic change of words across time. In Proceed-
ings of the 14th ACM/IEEE-CS Joint Conference on
Digital Libraries, pages 229–238.
Jurish, B. (2015). DiaCollo: On the trail of diachronic col-
locations. In De Smedt, K., editor, CLARIN Annual
Conference 2015 (Wro\law, Poland, October 1517
2015), pages 28–31.
Kenter, T., Wevers, M., Huijnen, P., and de Rijke, M.
(2015). Ad hoc monitoring of vocabulary shifts over
time. In Proceedings of the 24th ACM International
on Conference on Information and Knowledge Man-
agement, CIKM ’15, pages 1191–1200.
Kim, Y., Chiu, Y.-I., Hanaki, K., Hegde, D., and Petrov, S.
(2014). Temporal analysis of language through neu-
ral language models. In Proceedings of the ACL 2014
Workshop on Language Technologies and Computa-
tional Social Science, pages 61–65.
Michel, J.-B., Shen, Y. K., Aiden, A. P., Veres, A.,
Gray, M. K., The Google Books Team, Pickett, J. P.,
Hoiberg, D., Clancy, D., Norvig, P., Orwant, J.,
Pinker, S., Nowak, M. A., and Aiden, E. L. (2011).
Quantitative Analysis of Culture Using Millions of
Digitized Books. Science, 331(6014):176–182.