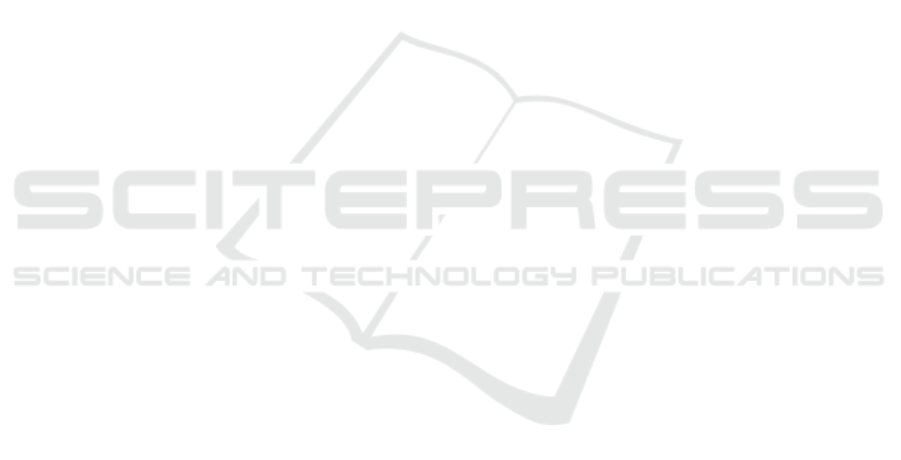
Hansen, N. and Kern, S. (2004). Evaluating the cma evolu-
tion strategy on multimodal test functions. Proceed-
ings of Eighth International Conference on Parallel
Problem Solving from Nature, PPSN VIII, 3242:282–
291.
Hedar, A.-R., Ong, B., and Fukushima, M. (2007). Genetic
algorithms with automatic accelerated termination.
Jain, B., Pohlheim, H., and Wegener, J. (2001). On termi-
nation criteria of evolutionary algorithms. pages 768–
768.
Kitchenham, B. and Charters, S. (2007). Guidelines for per-
forming systematic literature reviews in software en-
gineering.
Konar, A. (2005). Computational Intelligence: Principles,
Techniques and Applications. Springer-Verlag New
York, Inc., Secaucus, NJ, USA.
Lee, C.-Y. and Yao, X. (2004). Evolutionary programming
using mutations based on the levy probability distri-
bution. Trans. Evol. Comp, 8(1):1–13.
Leung, Y. and Wang, Y. (2001). An orthogonal genetic al-
gorithm with quantization for global numerical opti-
mization. Trans. Evol. Comp, 5(1):41–53.
Lozano, J. A., Larra
˜
naga, P., Inza, I. n., and Bengoetxea, E.
(2006). Towards a New Evolutionary Computation:
Advances on Estimation of Distribution Algorithms
(Studies in Fuzziness and Soft Computing). Springer-
Verlag New York, Inc., Secaucus, NJ, USA.
Mart
´
ı, L., Garc
´
ıa, J., Berlanga, A., and Molina, J. M.
(2007). A cumulative evidential stopping criterion for
multiobjective optimization evolutionary algorithms.
In Proceedings of the 9th Annual Conference Com-
panion on Genetic and Evolutionary Computation,
GECCO ’07, pages 2835–2842, New York, NY, USA.
ACM.
Mart
´
ı, L., Garc
´
ıa, J., Berlanga, A., and Molina, J. M.
(2009). An approach to stopping criteria for multi-
objective optimization evolutionary algorithms: The
mgbm criterion. IEEE Congress on Evolutionary
Computation.
Murthy, C., Bhandari, D., and Pal, S. K. (1998). e-optimal
stopping time for genetic algorithms. Fundam. In-
form., 35(1-4):91–111.
Naujoks, B. and Trautmann, H. (2009). Online conver-
gence detection for multiobjective aerodynamic appli-
cations. In Proceedings of the Eleventh Conference
on Congress on Evolutionary Computation, CEC’09,
pages 332–339, Piscataway, NJ, USA. IEEE Press.
Ong, Y., Lim, M., Zhu, N., and Wong, K. (2006). Classi-
fication of adaptive memetic algorithms: A compara-
tive study. IEEE Transactions on Systems, Man, and
Cybernetics-Part B, 36(1):141–152.
Petersen, K., Feldt, R., Mujtaba, S., and Mattsson, M.
(2008). Systematic mapping studies in software en-
gineering. In Proceedings of the 12th International
Conference on Evaluation and Assessment in Software
Engineering, EASE’08, pages 68–77, Swindon, UK.
BCS Learning & Development Ltd.
Rudenko, O. and Schoenauer, M. (2004). A steady perfor-
mance stopping criterion for pareto-based evolution-
ary algorithms. The 6th International Multi-Objective
Programming and Goal Programming Conference.
Rudolph, G. (1994). Convergence analysis of canonical ge-
netic algorithms. Trans. Neur. Netw., 5(1):96–101.
Suzuki, J. (1995). A markov chain analysis on simple ge-
netic algorithms. IEEE Transactions on Systems, Man,
and Cybernetics, 25(4):655–659.
Trautmann, H., Ligges, U., Mehnen, J., and Preuss, M.
(2008). A convergence criterion for multiobjective
evolutionary algorithms based on systematic statis-
tical testing. In Proceedings of the 10th Interna-
tional Conference on Parallel Problem Solving from
Nature: PPSN X, pages 825–836, Berlin, Heidelberg.
Springer-Verlag.
Trautmann, H., Wagner, T., Naujoks, B., Preuss, M., and
Mehnen, J. (2009). Statistical methods for conver-
gence detection of multi-objective evolutionary algo-
rithms. Evol. Comput., 17(4):493–509.
Tsai, J.-T., Liu, T.-K., and Chou, J.-H. (2004). Hybrid
taguchi-genetic algorithm for global numerical opti-
mization. IEEE Transactions on Evolutionary Com-
putation, 8(4):365–377.
Wagner, T. and Trautmann, H. (2010). Online convergence
detection for evolutionary multi-objective algorithms
revisited. IEEE Congress on Evolutionary Computa-
tion.
Wagner, T., Trautmann, H., and Mart
´
ı, L. (2011). A
taxonomy of online stopping criteria for multi-
objective evolutionary algorithms. In Proceedings
of the 6th International Conference on Evolutionary
Multi-criterion Optimization, EMO’11, pages 16–30,
Berlin, Heidelberg. Springer-Verlag.
Wagner, T., Trautmann, H., and Naujoks, B. (2009).
Ocd: Online convergence detection for evolutionary
multi-objective algorithms based on statistical testing.
In Proceedings of the 5th International Conference
on Evolutionary Multi-Criterion Optimization, EMO
’09, pages 198–215, Berlin, Heidelberg. Springer-
Verlag.
Zapotecas Mart
´
ınez, S., Y
´
a
˜
nez Oropeza, E. G., and
Coello Coello, C. A. (2011). Self-adaptation tech-
niques applied to multi-objective evolutionary algo-
rithms. In Proceedings of the 5th International
Conference on Learning and Intelligent Optimiza-
tion, LION’05, pages 567–581, Berlin, Heidelberg.
Springer-Verlag.
Zhong, W., Liu, J., Xue, M., and Jiao, L. (2004). A mul-
tiagent genetic algorithm for global numerical opti-
mization. IEEE Transactions on Systems, Man, and
Cybernetics-Part B, 34(2):1128–1141.
Zitzler, E., Deb, K., and Thiele, L. (2000). Comparison
of multiobjective evolutionary algorithms: Empirical
results. Evol. Comput., 8(2):173–195.
Zitzler, E., Thiele, L., Laumanns, M., Fonseca, C. M., and
da Fonseca, V. G. (2003). Performance assessment
of multiobjective optimizers: An analysis and review.
Trans. Evol. Comp, 7(2):117–132.