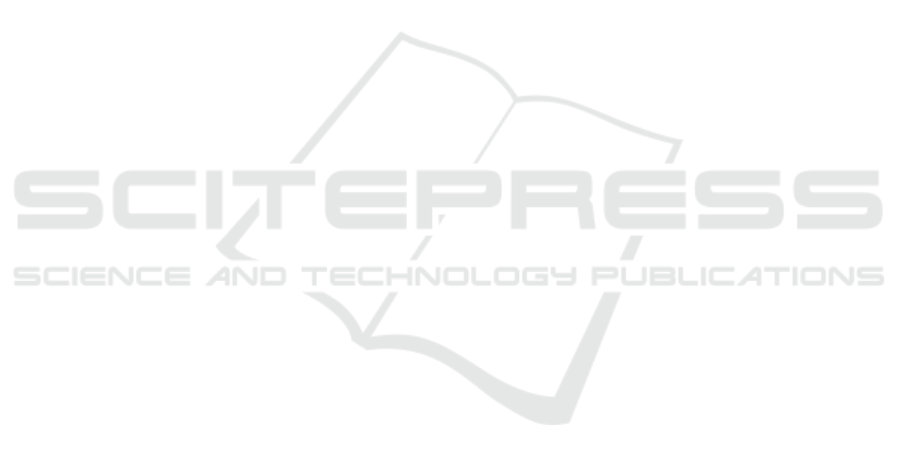
the model and importantly, the efficiency of the ap-
proaches in the absence of a large amount of data.
The multilayer Perceptron showed a different fig-
ure. While it performed well for the long texts, it per-
formed quite poor for the sentence classification. This
shows that the classifier has not been able to guess the
dialect with a high accuracy for short sentences. The
preliminary investigations show that this primarily is
due to the close relation between Kurmanji and So-
rani dialects which makes it difficult to differentiate
between the two dialects based on short sentences.
However, it requires further study to find out other
possible reasons for this outcome.
4 CONCLUSIONS
The article discussed the importance of the task of di-
alect identification in Kurdish NLP and CL. Through
emphasizing the dialect diversity and resource paucity
we presented the idea of using ANN to identify the
different Kurdish dialects in Kurdish texts. We in-
vestigated the efficiency and accuracy of ANN based
classifiers in the absence of large amount of texts
or corpora. We also compared the outcomes of
this approach with the previous work (see (Hassani
and Medjedovic, 2016)) on automatic Kurdish dialect
identification to compare the accuracy and perfor-
mance among the two approaches. The results sug-
gested that while the two approaches do not show
a significant difference in their accuracy and perfor-
mance with regard to long documents, the ANN ap-
proach performs better than traditional approach for
the single sentence classification. However, because
we were not able to find any baseline for the sentence
classifiers in Kurdish dialect identification studies, we
were not able to compare this part of the outcome.
Nevertheless, the sentence classifier performed with a
high accuracy at 99% for Kurmanji and 96% for So-
rani.
The multilayer Perceptron acted differently. It
provided quite a poor result for the sentence classi-
fication, while showed a reasonable accuracy for the
long texts. The early investigations suggest that this
behavior could be justified based on the close rela-
tion between Kurmanji and Sorani dialects. However,
more research is needed to become more certain about
this situation and to enhance the classifier to be able
to classify short sentences with a higher accuracy.
As for future work, we are interested in expanding
the research to cover the texts written in other scripts
for example, Persian/Arabic. We are also interested
in including other Kurdish dialects such as Hawrami
in the classification process. In addition, we believe
that the multilayer Perceptron requires further studies,
particularly on the error minimization process. We
are planning to work on the mentioned areas in an
extended paper that follows the current work.
ACKNOWLEDGEMENTS
The authors would like to thank the anonymous re-
viewers for their constructive suggestions and recom-
mendations which have improved the content of the
paper.
REFERENCES
Ali, A., Dehak, N., Cardinal, P., Khurana, S., Yella, S. H.,
Glass, J., Bell, P., and Renals, S. (2015). Automatic
Dialect Detection in Arabic Broadcast Speech. arXiv
preprint arXiv:1509.06928.
Belinkov, Y. and Glass, J. (2016). A Character-level
Convolutional Neural Network for Distinguishing
Similar Languages and Dialects. arXiv preprint
arXiv:1609.07568.
Foundation Institute Kurde de Paris (2017a). The Kurdish
Diaspora.
Foundation Institute Kurde de Paris (2017b). The Kurdish
Population.
Ghiassi, M., Olschimke, M., Moon, B., and Arnaudo, P.
(2012). Automated text classification using a dynamic
artificial neural network model. Expert Systems with
Applications, 39(12):10967–10976.
Gkanogiannis, A. and Kalamboukis, T. (2009). A Modi-
fied and Fast Perceptron Learning Rule and its Use
for Tag Recommendations in Social Bookmarking
Systems. ECML PKDD Discovery Challenge 2009
(DC09), page 71.
Gl
¨
uge, S., Hamid, O. H., and Wendemuth, A. (2010).
A Simple Recurrent Network for Implicit Learning
of Temporal Sequences. Cognitive Computation,
2(4):265–271.
Haig, G. and
¨
Opengin, E. (2014). Introduction to Special
Issue-Kurdish: A critical research overview. Kurdish
Studies, 2(2):99–122.
Hassani, H. (2017a). BLARK for multi-dialect languages:
towards the Kurdish BLARK. Language Resources
and Evaluation, pages 1–20.
Hassani, H. (2017b). Kurdish Interdialect Machine Transla-
tion. In Proceedings of the Fourth Workshop on NLP
for Similar Languages, Varieties and Dialects (Var-
Dial), pages 63–72. Association for Computational
Linguistics.
Hassani, H. and Medjedovic, D. (2016). Automatic Kurdish
Dialects Identification. Computer Science & Informa-
tion Technology, 6(2):61–78.
Hassanpour, A. (1992). Nationalism and language in Kur-
distan, 1918-1985. Edwin Mellen Pr.