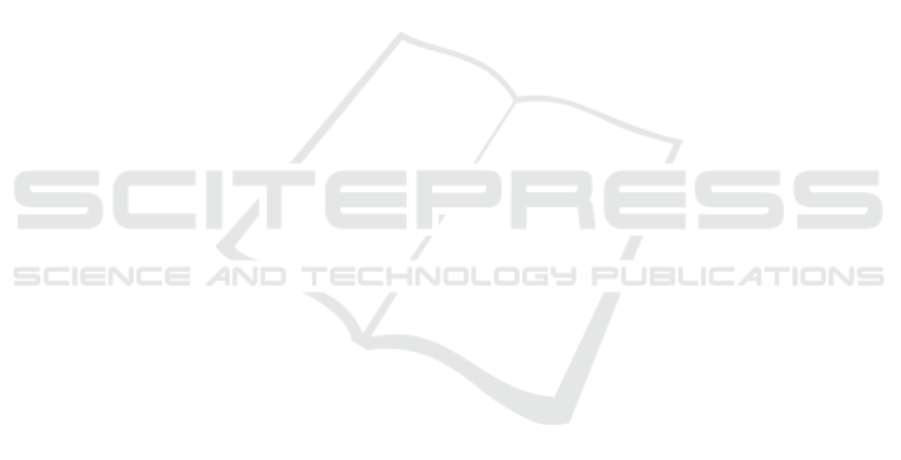
expresses the effect of network statistics in the
monitored network model. The coefficient k
represents the number of numerators displayed in a
possible network with n number of elements.
Parameters are initially estimated in ERGM
using pseudo-similarity (Strauss and Ikeda, 1990)
but this approach is often not reliable. Instead, it is
more appropriate to use the Markov Chain of Monte
Carlo as a similarity estimate (Geyer and Thompson,
1992). Monte Carlo simulates the distribution of a
random graph using the initial values of the
parameters, and this process is repeated until we
reach the cleaned values that we compare with the
simulated distribution of the given graph with the
observed data (Snijders, 2002). The advantage of
this approach is that with an infinite number of
network configuration distributions, we give an
estimate equivalent to maximum like-hood
estimation and provide a reliable number of standard
errors (Wasserman and Robins, 2005). A series of
other network specifications, called the social circuit
dependence (Pattison and Robins, 2002), have been
developed that significantly reduce cases where the
high level of triads often occurs due to poor model
specification (Hunter and Handcock, 2006, Robins
et al., 2009).
5 DISCUSSION
The possibilities of using the ERGM method on
economic themes have been demonstrated in (Kim et
al., 2016), when the connections between firms and
their boards of directors (Mizruchi, 1996) were
modeled. The number of selected companies were
set on 95. The influence of the independent variables
on the characteristics of various companies was
studied, namely the company characteristics, dyad
co-variates and structural effects. These properties
identifies as important features for establishing a
connection within a multinational network in
international trade. If companies have similar or
even the same company characteristics, or Structural
effects, it is possible with some probability to define
that there is a connection within the network.
The ERGM methodology allows significant
expansion for the development of a strategy to
expand business opportunities or attract new
potential customers and businesses in the field of
transnational trade. ERGM's ability to directly
describe network dependencies allows for a more
accurate analysis of the factors that were examined
in the previous research. The results can be used to
test the formation of connections to create rigorous
empirical evidence for developing models that are
not only attractive but also scientifically valid
(Colquitt and Zapata-Phelan, 2007). Previous
research proves that the influence of organizational
resources on alliance formation is important. Multi-
source companies have more opportunities to build
links, and their higher level of resources makes it
possible to eliminate the need for co-operation
through the alliances.
The promising area where ERGM can be used is
also the area of micro-transactions in the area of
inter-organizational and transnational cooperation.
Various research highlights the important role of
existing links between individuals and their
connection to the creation of links between
organizations (Gulati and Westphal, 1999,
Rosenkopf et al., 2001). Typical examples are new
startups that are founded by people who previously
worked in multinational companies. These people
are then linked to the staff of these large companies
and can be contacted for further cooperation.
In conclusion, ERGM is an important technique
that can be used in various areas of research, be it in
the field of international trade and modeling of
producer and vendor networks, but also in other
economic areas such as strategic research, that can
be taking into account of transnational trade. As the
interconnection between individuals, organizations
or entire communities grows, there is a growing
need to better understand and understand structures
within networks. The ERGM method can build on
the classic foundations of social network analysis
and move them in the next direction to better
understand the organization network genesis.
ACKNOWLEDGEMENTS
This paper is supported by project number
SG470022.
REFERENCES
Albert, R., Jeong, H. & Barabasi, A. L. 1999. Internet -
Diameter of the World-Wide Web. Nature, 401, 130-
131.
Anderson, J. E. & Marcouiller, D. 2002. Insecurity and the
pattern of trade: An empirical investigation. Review of
Economics and Statistics, 84, 342-352.
Eaton, J. & Kortum, S. 2002. Technology, geography, and
trade. Econometrica, 70, 1741-1779.
Erdos, P. & Renyi, A. 1966. On Existence Of A Factor Of
Degree 1 Of A Connected Random Graph. Acta