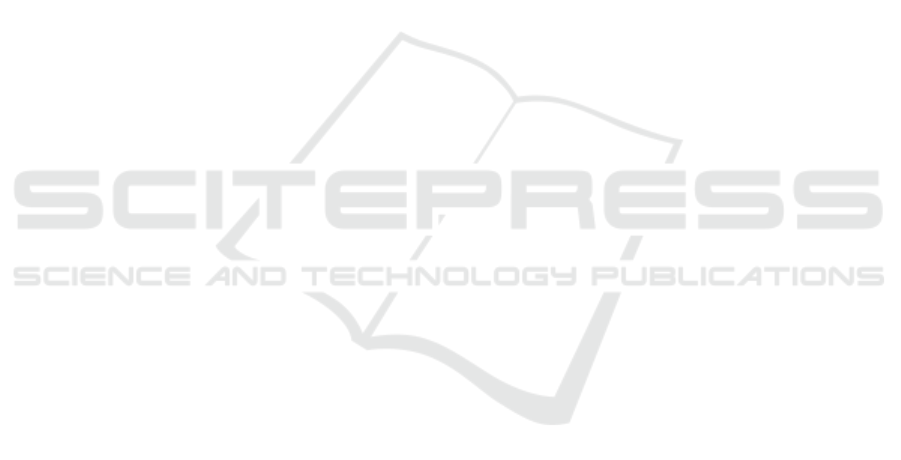
component, the proposed method is not restricted to
SPoC and can be utilized to characterize any type of
spatial filter / component.
We propose to use a component’s persistence in
the time- and frequency domain in order to track
changes over sessions and we argue that this is use-
ful in various scenarios. Examples are cognitive and
memory tasks (Klimesch, 1999), when changes of
oscillatory activity is induced by motor learning in
sports, or over the course of BCI-supported motor re-
habilitation after stroke — a field which recently re-
ceived a lot of attention (Soekadar et al., 2015; Rem-
sik et al., 2016). In experimental scenarios with a re-
stricted, similar functional context, this form of anal-
ysis may even help to identify corresponding oscil-
latory components across users and can thus support
novel forms of group level analyses.
ACKNOWLEDGEMENTS
The authors are thankful for support by the Clus-
ter of Excellence BrainLinks-BrainTools, funded by
the German Research Foundation (DFG, grant num-
ber EXC 1086) and by state of Baden-W
¨
urttemberg
through bwHPC and the German Research Founda-
tion (DFG) through grant no INST 39/963-1 FUGG.
Finally, we want to thank Katharina Eggensperger and
Frank Hutter for providing software on the hyperpa-
rameter analysis.
REFERENCES
Casta
˜
no-Candamil, J. S., Meinel, A., D
¨
ahne, S., and Tanger-
mann, M. (2015). Probing meaningfulness of oscilla-
tory EEG components with bootstrapping, label noise
and reduced training sets. In Engineering in Medicine
and Biology Society (EMBC), 2015 37th Annual Inter-
national Conference of the IEEE, pages 5159–5162.
IEEE.
D
¨
ahne, S., Meinecke, F. C., Haufe, S., H
¨
ohne, J., Tanger-
mann, M., M
¨
uller, K.-R., and Nikulin, V. V. (2014).
SPoC: a novel framework for relating the amplitude of
neuronal oscillations to behaviorally relevant parame-
ters. NeuroImage, 86(0):111–122.
Fukunaga, K. (1990). Introduction to statistical pattern
recognition (2nd ed.). Academic Press Professional,
Inc., San Diego, CA, USA.
Haufe, S., Meinecke, F., G
¨
orgen, K., D
¨
ahne, S., Haynes, J.-
D., Blankertz, B., and Biemann, F. (2014). On the
interpretation of weight vectors of linear models in
multivariate neuroimaging. NeuroImage, 87(0):96 –
110.
H
¨
ohne, J., Holz, E., Staiger-S
¨
alzer, P., M
¨
uller, K.-R.,
K
¨
ubler, A., and Tangermann, M. (2014). Motor im-
agery for severely motor-impaired patients: Evidence
for brain-computer interfacing as superior control so-
lution. PLoS ONE, 9(8):e104854.
Hutter, F., Hoos, H., and Leyton-Brown, K. (2014). An
efficient approach for assessing hyperparameter im-
portance. In Proceedings of International Conference
on Machine Learning 2014 (ICML 2014), pages 754–
762.
Klimesch, W. (1999). EEG alpha and theta oscillations re-
flect cognitive and memory performance: a review
and analysis. Brain Research Reviews, 29(2):169–
195.
Koles, Z. J. (1991). The quantitative extraction and topo-
graphic mapping of the abnormal components in the
clinical EEG. Electroencephalography and clinical
Neurophysiology, 79(6):440–447.
Lotte, F. and Guan, C. (2011). Regularizing common spa-
tial patterns to improve BCI designs: unified theory
and new algorithms. IEEE Transactions on Biomedi-
cal Engineering, 58(2):355–362.
Meinel, A., Casta
˜
no-Candamil, S., Reis, J., and Tanger-
mann, M. (2016a). Pre-trial EEG-based single-trial
motor performance prediction to enhance neuroer-
gonomics for a hand force task. Frontiers in Human
Neuroscience, 10:170.
Meinel, A., Castao-Candamil, J. S., D
¨
ahne, S., Reis, J., and
Tangermann, M. (2015). EEG band power predicts
single-trial reaction time in a hand motor task. In Proc.
Int. IEEE Conf. on Neural Eng. (NER), pages 182–
185, Montpellier, France. IEEE.
Meinel, A., Eggensperger, K., Tangermann, M., and Hut-
ter, F. (2016b). Hyperparameter optimization for ma-
chine learning problems in BCI. In M
¨
uller-Putz, G.,
Huggins, J., and Steyrl, D., editors, Proceedings of the
6th International Brain-Computer Interface Meeting:
BCI Past, Present, and Future, page 184, Asilomar
Conference Center, Pacific Grove, California, USA.
Verlag der Technischen Universit
¨
at Graz.
Meyer, T., Peters, J., Zander, T. O., Sch
¨
olkopf, B.,
and Grosse-Wentrup, M. (2014). Predicting motor
learning performance from electroencephalographic
data. Journal of Neuroengineering and Rehabilitation,
11(1):24.
Mousavi, M., Koerner, A. S., Zhang, Q., Noh, E., and
de Sa, V. R. (2017). Improving motor imagery BCI
with user response to feedback. Brain-Computer In-
terfaces, 4(1-2):74–86.
M
¨
uller, K.-R., Tangermann, M., Dornhege, G., Krauledat,
M., Curio, G., and Blankertz, B. (2008). Machine
learning for real-time single-trial EEG-analysis: From
brain-computer interfacing to mental state monitoring.
Journal of Neuroscience Methods, 167(1):82–90.
Pfutscheller, G., Neuper, C., Flotzinger, D., and Pregen-
zer, M. (1997). EEG-based discrimination between
imagination of right and left hand movement. Elec-
troencephalography and Clinical Neurophysiology,
103:642–651.
Ramoser, H., M
¨
uller-Gerking, J., and Pfurtscheller, G.
(2000). Optimal spatial filtering of single trial EEG
during imagined hand movement. Rehabilitation En-
gineering, IEEE Transactions on, 8(4):441–446.