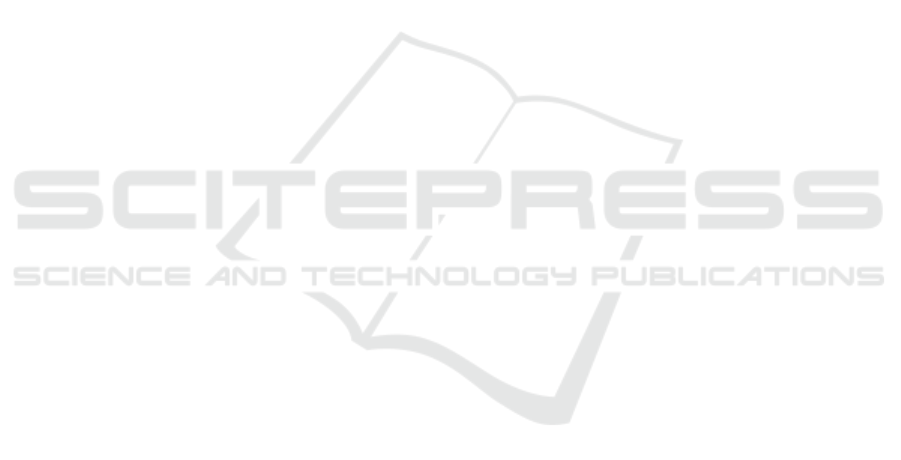
would be able to use the whole World Wide Web as
a domain knowledge, but this possesses challenges
like information inconsistency and sense
disambiguation. The second direction is to improve
the structure of the created sentences. We use subject-
predicate-object triplets extended by adjective and
adverb modifiers. Such structure can be improved by
using more advanced syntactic representation of the
sentence, e.g. graph representation. Finally, some of
the created sentences are not conceptually connected
to each other. Analysing the relations between
concepts on the document level will help in creating
sentences that will be linked to each other
conceptually.
REFERENCES
Barzilay, R. & Elhadad, M., 1999. Using lexical chains for
text summarization. Advances in automatic text
summarization, pp. 111-121.
Bellare, K. et al., 2004. Generic Text Summarization Using
WordNet. Lisbon, Portugal, LREC, pp. 691-694.
Bing, L. et al., 2015. Abstractive multi-document
summarization via phrase selection and merging.
Beijing, China, Association for Computational
Linguistics, pp. 1587-1597.
Cheung, J. C. K. & Penn, G., 2013. Towards Robust
Abstractive Multi-Document Summarization: A
Caseframe Analysis of Centrality and Domain.. Sofia,
Bulgaria, Association for Computational Linguistics,
pp. 1233-1242.
Choi, B. & Huang, X., 2010. Creating New Sentences to
Summarize Documents. Innsbruck, Austria, IASTED,
pp. 458-463.
Cycorp, 2017. Cycorp – Cycorp Making Solutions Better.
[Online]
Available at: http://www.cyc.com/
[Accessed July 2017].
Ganesan, K., Zhai, C. & Han, J., 2010. Opinosis: a graph-
based approach to abstractive summarization of highly
redundant opinions. Beijing, China, Association for
Computational Linguistics, pp. 340-348.
Gong, Y. & Liu, X., 2001. Generic text summarization
using relevance measure and latent semantic analysis.
New Orleans, Louisiana, ACM, pp. 19-25.
Günes, E. & Radev, D. R., 2004. Lexrank: Graph-based
lexical centrality as salience in text summarization.
Journal of Artificial Intelligence Research, Issue 22,
pp. 457-479.
Honnibal, M. & Johnson, M., 2015. An Improved Non-
monotonic Transition System for Dependency Parsing.
Lisbon, Portugal, Association for Computational
Linguistics, pp. 1373-1378.
Hovy, E. & Lin, C.-Y., 1998. Automated text
summarization and the SUMMARIST system.
Baltimore, Maryland, Association for Computational
Linguistics, pp. 197-214.
JPype, 2017. JPype - Java to Python integration. [Online]
Available at: http://jpype.sourceforge.net/
[Accessed July 2017].
Luhn, H. P., 1958. The automatic creation of literature
abstracts. IBM Journal of Research and Development,
2(2), pp. 159-165.
Matuszek, C., Cabral, J., Witbrock, M. & DeOliveira, J.,
2006. An Introduction to the Syntax and Content of Cyc.
Palo Alto, California, AAAI, pp. 44-49.
Mihalcea, R. & Tarau, P., 2004. TextRank: Bringing Order
into Text. Barcelona, Spain, EMNLP, pp. 404-411.
Moawad, I. F. & Aref, M., 2012. Semantic graph reduction
approach for abstractive Text Summarization. Cairo,
Egypt, IEEE, pp. 132-138.
Nallapati, R., Zhai, F. & Zhou, B., 2017. SummaRuNNer:
A recurrent neural network based sequence model for
extractive summarization of documents. San Francisco,
California, AAAI.
Nenkova, A. & McKeown, K., 2012. A survey of text
summarization techniques. In: C. C. Aggarwal & C.
Zhai, eds. Mining Text data. s.l.:Springer, pp. 43-76.
Pal, A. R. & Saha, D., 2014. An approach to automatic text
summarization using WordNet. Gurgaon, India, IEEE,
pp. 1169-1173.
Radev, D. R., Jing, H., Styś, M. & Tam, D., 2004. Centroid-
based summarization of multiple documents.
Information Processing & Management, 40(6), pp.
919-938.
Rodriguez, A. & Laio, A., 2014. Clustering by fast search
and find of density peaks. Science, 344(6191), pp.
1492-1496.
Rush, A. M., Chopra, S. & Wetson, J., 2015. A neural
attention model for abstractive sentence
summarization. Lisbon, Portugal, EMNLP.
Shen, D. et al., 2007. Document Summarization Using
Conditional Random Fields. Hyderabad, India, IJCAI,
pp. 2862-2867.
Wong, K.-F., Wu, M. & Li, W., 2008. Extractive
summarization using supervised and semi-supervised
learning. Manchester, United Kingdom, Association
for Computational Linguistics, pp. 985-992.
Ye, S., Chua, T.-S., Kan, M.-Y. & Qiu, L., 2007. Document
concept lattice for text understanding and
summarization. Information Processing &
Management, 43(6), pp. 1643-1662.