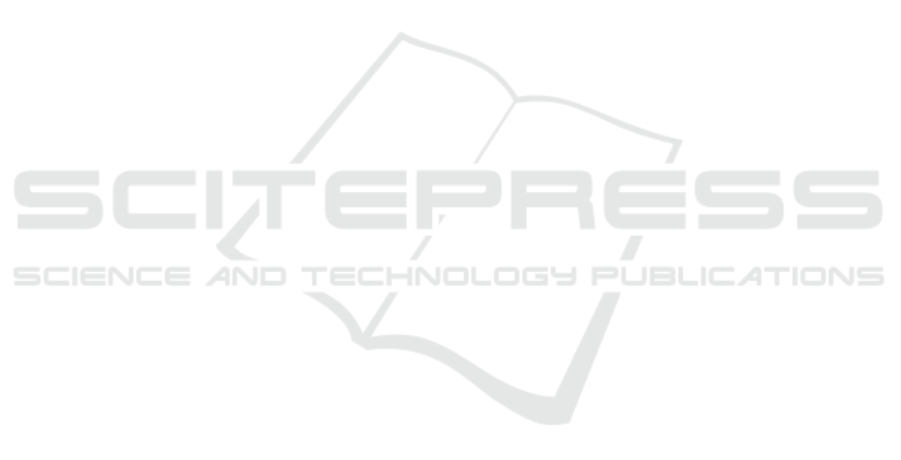
the action in the future, is a marketing manipula-
tion. Indeed, these methods have been invented for
e-business purposes (Mayer-Schonberger and Cukier,
2013). But unfortunately, in the present state, they are
not relevant for other domains such as digital collec-
tions, e-learning or also social networks.
5.2 Limits and Further Work
We are aware that our experiment is subject to limi-
tations. Some limitations may be pushed away with
further work.
• Multiplying empirical experiments with a similar
methodology, relying on large, reliable and vari-
ous datasets. Moreover, meta information that we
have used in confirmation measure, is difficult to
obtain. Other data might be used. In addition, we
have chosen to connect successively visited items.
It’s a common way to structure users activities.
But, some other models of interactions might be
experimented.
• Formalizing mathematical validations: repro-
ducing empirical demonstrations should not be
enough to fully validate our purpose. Mathemati-
cal demonstrations should be given.
• Experimenting in real-time: working with termi-
nated user activities makes a key factor invisible:
whether or not the user has been influenced by
the suggestion. Experimenting in real-time should
help to concretely measure the impact and con-
ceptualize the mutual influences.
• Organizing a longitudinal study: another way to
represent the iterative shift is by observing trajec-
tories and influences other a larger period. This
can give access to several views, that we could
compare through a longitudinal representation.
REFERENCES
Adamic, L. and Adar, E. (2005). Friends and neighbors on
the web, in social networks.
Adamic, L. and Glance, N. (2005). The political blogo-
sphere and the 2004 u.s election: Divided they blog. In
3rd international workshop on Link discovery, pages
36–43.
Adomavicius, G., Bockstedt, J., Shawn, C., and Zhang, J.
(2014). De-biasing user preference ratings in recom-
mender systems. In Interfaces and Human Decision
Making for Recommender Systems, Foster City, USA.
Adomavicius, G., Bockstedt, J. C., Curley, S. P., and Zhang,
J. (2013). Do recommender systems manipulate con-
sumer preferences? a study of anchoring effects. Info.
Sys. Research, 24(4):956–975.
Anderson, C. (2006). The Long Tail: How the Future of
Business is Selling Less of More. Hyperion Books,
New York, USA.
Arekar, T., Sonar, S., and Uke, J. (2015). A survey on rec-
ommendation system.
Austerweil, J. and Griffiths, T. (2011). Seeking confirma-
tion is rational for deterministic hypotheses.
Avazpour, I., Pitakrat, T., Grunske, L., and Grundy, J.
(2014). Dimensions and Metrics for Evaluating Rec-
ommendation Systems, pages 245–273. Springer
Berlin Heidelberg, Berlin, Heidelberg.
Beuscart, J.-S. and Couronn
´
e, T. (2009). The distribution
of online reputation: Audience and influence of musi-
cians on myspace. In ICWSM Conference, pages 36–
43, San Jose, USA.
Bikhchandani, S., Hirshleifer, D., and Welch, I. (1998).
Learning from the behavior of others: Conformity,
fads, and informational cascades.
Blot, G., Rousseaux, F., and Saurel, P. (2014). Pattern dis-
covery in e-learning courses : a time based approach.
In CODIT14, Metz, France. IEEE.
Blot, G., Saurel, P., and Rousseaux, F. (2015). Recom-
mender engines under the influence of popularity. In
6th International MCETECH Conference, Montreal,
Canada. IEEE.
Bourcier, D. and Van Andel, P. (2011). La s
´
erendipit
´
e - Le
hasard heureux. Hermann.
Bronner, G. (2013). La d
´
emocratie des cr
´
edules. Presses
universitaires de France, Paris, France.
Candillier, L., Chevalier, M., Dudognon, D., and Mothe,
J. (2011). Diversity in recommender systems: bridg-
ing the gap between users and systems. In CENTRIC,
page 48–53.
Cha, M., Kwak, H., Rodriguez, P., Ahn, Y.-Y., and Moon,
S. (2007). I tube, you tube, everybody tubes. In
7th ACM SIGCOMM conference on Internet measure-
ment, New York, USA.
Ellis, C. and Fender, J. (2011). Riots, revolutions,
democratisation, and information cascades. In VOX.
CEPR.
Ge, M., Delgado-battenfeld, C., and Jannach, D. (2010).
Beyond accuracy: evaluating recommender systems
by coverage and serendipity. In In RecSys ’10, page
257.
Herring, S., Kouper, I., Kouper, J., Scheidt, L.-A., Tyworth,
M., Wright, P., and Ning, Y. (2005). Conversations in
the blogosphere: An analysis ”from the bottom up”.
Hawaii.
Hindman, M., Tsioutsiouliklis, K., and Johnson, J. (2003).
Googlearchy: How a few heavily-linked sites domi-
nate politics on the web. In Annual Meeting of the
Midwest Political Science Ass.
Katz, D. and Allport, F. H. (1931). Students’ Attitudes:
A Report of the Syracuse University Reaction Study.
Craftsman Press, New York, USA.
Mayer-Schonberger, V. and Cukier, K. (2013). Big Data: A
Revolution That Will Transform How We Live, Work,
and Think. HAL.
Origgi, G. (2015). La r
´
eputation. Presses universitaires de
France, Paris, France.