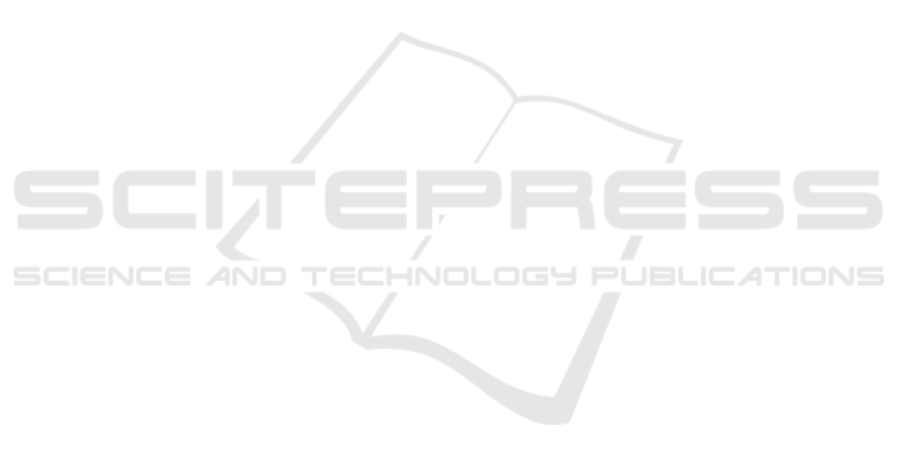
households with the highest degrees of primary
school (71.56 percent), working in agriculture (64.65
percent) with unpaid employment assisted workers
(53.65 percent), 72 percent) and those with free
workers (24.88 percent). This suggests that poor
households working in the informal sector are more
than 70 percent educated with a low formal education
so that they will have limited skills and skills. They
mostly work on agricultural sectors that do not
require certain skills.
Other employment variables that can describe
the characteristics of poverty in D.I. Yogyakarta is the
average member of the working household (MPKR).
Based on the results of logit estimation, obtained the
estimated value of MPKR coefficient of -0.371064
with an odd ratio of 0.6900. This means that the
average number of household members working
increased 1, the probability that households will fall
into the poor category will be 0.6900 house-holds
with fewer household members working. This means
that the more stout the members of the house-hold are
working, the less likely the households will be poor.
The marginal effect of the MPKR variable is -0.0281,
which means that households will fall into the poor
category reduced by 2.81 percent if the number of
working households increases by one per-son.
Increasing the average number of working household
members will lower the likelihood of households
being categorised as poor.
The estimated value of the dummy of residence
(GEO3) is significant at the 5 percent significance
level with an estimated value of 0.404787, while the
odds ratio of 0.6671. This means that the probability
of households falling into the category of poor living
in the mainland area is 0.6671 times than in non-
mainland areas. These results indicate that non-land
areas have a higher poverty risk than inland areas.
The marginal effect of -0.0307. This means that
households in the poor category for households in the
mainland area will be reduced by 3.07 percent com-
pared with households living in non-mainland areas.
Characteristics of residence based on the location of
topography turned out to affect poverty. This is be-
cause the topography of the non-mainland area is
made up of slopes and valleys. This region is a
mountainous region so that transportation facilities
are still difficult, facilities and infrastructure that
support the fulfilment of household needs such as
education, health, economy or entertainment is still
very mini-mal. This result is not much different from
the results of the study of the determinants of poverty
in Kenya showing that poverty rates differ in different
regions (Kabananukye, et al., 2004).
4 CONCLUSIONS
The results of estimation and analysis, obtained some
conclusions as follows. Household size, age of head
of household, occupation of head of household,
employment status of head of household, occupation
of head of household, average of working member of
household, highest certificate owned by head of
household, average length of school of household
member And residential areas are the variables that
are able to explain the characteristics of poor
households in Special Province of Yogyakarta in
2013. Variables that can increase poverty by 2013
from the order of magnitude of marginal effect are:
employment in the agricultural sector, informal
employment status, and household size. Variables
that can reduce poverty by 2013 are the area of
mainland residence, the average member of the
working household, the average length of the
schooling of household members, the highest
certificate held by the head of the household and the
age of the head of the household. Increased
investment of human resources through formal
education can reduce the chances of poor households.
In contrast, agricultural employment, informal
employment status will increase the likelihood of
poor households.
REFERENCES
Afza, et al. 2013. Relationship among Education, Poverty
and Economic Growth in Pakistan: An Econometric
Analysis, Journal of Elementary Education Vol.22, No.
1 pp.23-45
Barnes, S., 2005. The Cost of being Poor: A Comparative
Study of Life in Poor Urban Neighbourhoods in Gary,
Indiana. State University of New York Press. Albany.
Bassetti, T., 2008. Economic Growth : Institutional and
Social Dynamics Education and Poverty in a Solow
Growth Model, (February).
Bassetti, T., 2012. Education as a social agreement. Journal
of Socio-Economics, 41(1), 8–17.
https://doi.org/10.1016/j.socec.2011.10.005
Brück, T., n.d.. Determinants of Poverty During
Transition : Household Survey Evidence From
Ukraine, (December 2007), 1–40.
Christiaensen, L., Todo, Y., 2014. Poverty reduction during
the rural-urban transformation - The role of the missing
middle. World Development, 63, 43–58.
https://doi.org/10.1016/j.worlddev.2013.10.002
Geda, A., de Jong, N., Kimenyi, M. S., Mwabu, G., 2005.
Determinants of Poverty in Kenya : A Household Level
Analysis. Economic Working Papers - Digital
Commons, (August). https://doi.org/ISSN 0921-0210
Grimm, M., 2005. Educational policies and poverty
reduction in C??te d’Ivoire. Journal of Policy
ICEEE 2017 - 2nd International Conference on Economic Education and Entrepreneurship
730