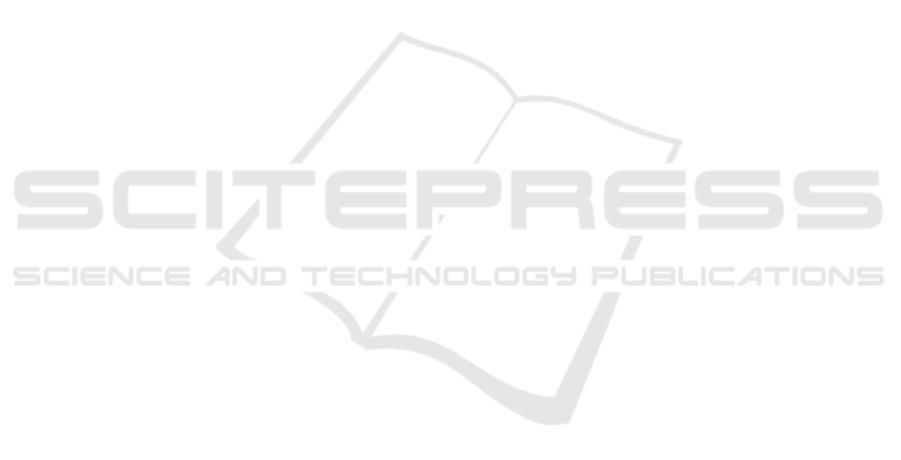
on Security and High Performance Computing Systems (SHPCS 2018). pp. 3:1–3:8. IEEE
Computer Society (July 2018)
5. Biryukov, A. (ed.): The 128-Bit Blockcipher CLEFIA (Extended Abstract), Lecture Notes in
Computer Science, vol. 4593. Springer (2007). https://doi.org/10.1007/978-3-540-74619-5,
https://doi.org/10.1007/978-3-540-74619-5
6. Box, G.E., Cox, D.R.: An analysis of transformations. Journal of the Royal Statistical Soci-
ety. Series B (Methodological) pp. 211–252 (1964)
7. Box, G.E., Jenkins, G.M., Reinsel, G.C., Ljung, G.M.: Time series analysis: forecasting and
control. John Wiley & Sons (2015)
8. Cleveland, R.B., Cleveland, W.S., Terpenning, I.: Stl: A seasonal-trend decomposition pro-
cedure based on loess. Journal of Official Statistics 6(1), 3 (1990)
9. Enev, M., Takakuwa, A., Koscher, K., Kohno, T.: Automobile driver fingerprint-
ing. Proceedings on Privacy Enhancing Technologies 2016(1), 34–50 (2016).
https://doi.org/10.1515/popets-2015-0029
10. Ester, M., Kriegel, H., Sander, J., Xu, X.: A density-based algorithm for discovering clusters
in large spatial databases with noise. In: Proceedings of the 2
nd
International Conference
on Knowledge Discovery and Data Mining (KDD-96), Portland, Oregon, USA. pp. 226–231
(1996), http://www.aaai.org/Library/KDD/1996/kdd96-037.php
11. Fugiglando, U., Massaro, E., Santi, P., Milardo, S., Abida, K., Stahlmann, R., Netter, F.,
Ratti, C.: Driving behavior analysis through CAN bus data in an uncontrolled environment.
CoRR abs/1710.04133 (2017), http://arxiv.org/abs/1710.04133
12. Government, U.: Driving licence holding and vehicle availability (nts02) (2016), national
Travel Survey
13. Holt, C.C.: Forecasting seasonals and trends by exponentially weighted moving averages.
International journal of forecasting 20(1), 5–10 (2004)
14. Kwak, B.I., Woo, J., Kim, H.K.: Know your master: Driver profiling-based anti-theft method.
CoRR abs/1704.05223 (2017), http://arxiv.org/abs/1704.05223
15. Lachmair, J., Mer
´
enyi, E., Porrmann, M., R
¨
uckert, U.: A reconfigurable neuropro-
cessor for self-organizing feature maps. Neurocomputing 112, 189 – 199 (2013).
https://doi.org/https://doi.org/10.1016/j.neucom.2012.11.045, http://www.sciencedirect.
com/science/article/pii/S0925231213002385, advances in artificial neural networks,
machine learning, and computational intelligence
16. Ly, M.V., Martin, S., Trivedi, M.M.: Driver classification and driving style recognition using
inertial sensors. In: 2013 IEEE Intelligent Vehicles Symposium (IV). pp. 1040–1045 (June
2013). https://doi.org/10.1109/IVS.2013.6629603
17. Martinez, C.M., Heucke, M., Wang, F.Y., Gao, B., Cao, D.: Driving style recogni-
tion for intelligent vehicle control and advanced driver assistance: A survey. IEEE
Transactions on Intelligent Transportation Systems 19(3), 666–676 (March 2018).
https://doi.org/10.1109/TITS.2017.2706978
18. Oleksiak, A., T.Ciesielczyk, Kierzynka, M., Piatek, W.: Minimising energy costs of data
centers using high dense heterogeneous systems and intelligent resource management. In:
e-Energy ’18 Proceedings of the Ninth International Conference on Future Energy Systems.
pp. 499–505 (2018)
19. Oleksiak, A., Kierzynka, M., Piatek, W., Agosta, G., Barenghi, A., Brandolese, C., For-
naciari, W., Pelosi, G., Cecowski, M., Plestenjak, R.,
ˇ
Cinkelj, J., Porrmann, M., Hage-
meyer, J., Griessl, R., Lachmair, J., Peykanu, M., Tigges, L., vor dem Berge, M., Christ-
mann, W., Krupop, S., Carbon, A., Cudennec, L., Goubier, T., Philippe, J.M., Rosinger, S.,
Schlitt, D., Pieper, C., Adeniyi-Jones, C., Setoain, J., Ceva, L., Janssen, U.: M2DC – Modu-
lar Microserver DataCentre with heterogeneous hardware. Microprocessors and Microsys-
tems 52, 117 – 130 (2017). https://doi.org/https://doi.org/10.1016/j.micpro.2017.05.019,
http://www.sciencedirect.com/science/article/pii/S0141933116304574
175
The M2DC Approach towards Resource-efficient Computing
175