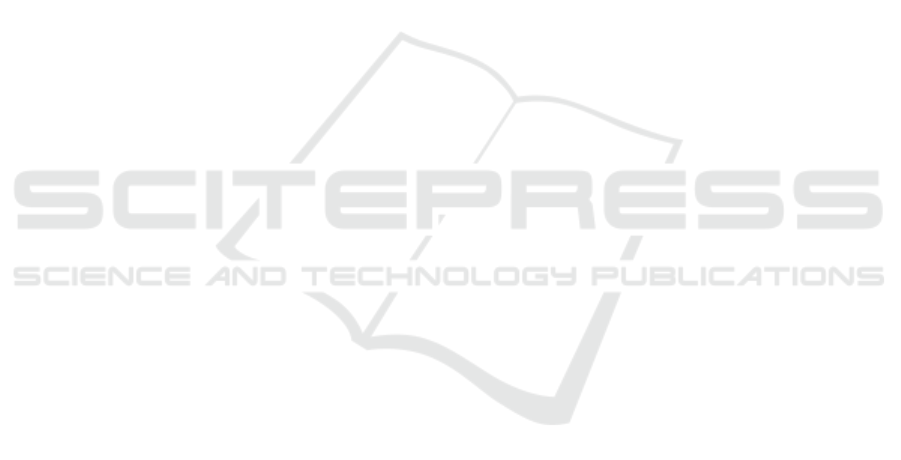
Meng Meng, Drira, H., Daoudi, M., and Boonaert, J.
(2015). Human-object interaction recognition by le-
arning the distances between the object and the skele-
ton joints. In 11th IEEE International Conference and
Workshops on Automatic Face and Gesture Recogni-
tion (FG), pages 1–6.
Niebles, J. C. and Fei-Fei, L. (2007). A Hierarchical Model
of Shape and Appearance for Human Action Classifi-
cation. In IEEE Conference on Computer Vision and
Pattern Recognition (CVPR), pages 1–8.
Nourani-Vatani, N., Borges, P. V. K., and Roberts, J. M.
(2012). A study of feature extraction algorithms for
optical flow tracking. In Proceedings of Australa-
sian Conference on Robotics and Automation, Victo-
ria University of Wellington, New Zealand., pages 1–
7.
Shi, Y., Zeng, W., Huang, T., and Wang, Y. (2015). Learning
Deep Trajectory Descriptor for action recognition in
videos using deep neural networks. IEEE Internatio-
nal Conference on Multimedia and Expo (ICME), pa-
ges 1–6.
Soares Beleboni, M. G. (2014). A brief overview of Mi-
crosoft Kinect and its applications. Interactive Mul-
timedia Conference, University of Southampton, UK,
pages 1–6.
Solmaz, B., Assari, S. M., and Shah, M. (2013). Classifying
web videos using a global video descriptor. Machine
Vision and Applications, 24(7):1473–1485.
Somasundaram, G., Cherian, A., Morellas, V., and Papa-
nikolopoulos, N. (2014). Action recognition using
global spatio-temporal features derived from sparse
representations. Computer Vision and Image Under-
standing, 123:1–13.
Uijlings, J., Duta, I. C., Sangineto, E., and Sebe, N.
(2015). Video classification with Densely extrac-
ted HOG/HOF/MBH features: an evaluation of the
accuracy/computational efficiency trade-off. Interna-
tional Journal of Multimedia Information Retrieval,
4(1):33–44.
Wang, H., Kl
¨
aser, A., Schmid, C., and Liu, C.-L. (2013a).
Dense Trajectories and Motion Boundary Descrip-
tors for Action Recognition. International Journal of
Computer Vision, Springer Verlag, 103(1):60–79.
Wang, H. and Schmid, C. (2013). Action Recognition with
Improved Trajectories. IEEE International Confe-
rence on Computer Vision (ICCV), pages 3551–3558.
Wang, J., Liu, Z., Wu, Y., and Yuan, J. (2014a). Learning
Actionlet Ensemble for 3D Human Action Recogni-
tion. IEEE Transactions on Pattern Analysis and Ma-
chine Intelligence, 36(5):914–927.
Wang, L. and Suter, D. (2007). Recognizing Human Activi-
ties from Silhouettes: Motion Subspace and Factorial
Discriminative Graphical Model. In EEE Computer
Society Conference on Computer Vision and Pattern
Recognition (CVPR).
Wang, Y., Li, Y., and Ji, X. (2013b). Recognizing Human
Actions Based on Gist Descriptor and Word Phrase.
Proceedings of International Conference on Mecha-
tronic Sciences, Electric Engineering and Computer
(MEC), pages 1104–1107.
Wang, Y., Li, Y., and Ji, X. (2014b). Human Action Re-
cognition Using Compact Global Descriptors Derived
from 2DPCA-2DLDA. In Proceedings of IEEE In-
ternational Conference on Computer and Information
Technology (CIT), pages 182–186.
Wang, Y., Li, Y., and Ji, X. (2015a). Human Action Recog-
nition Based on Global Gist Feature and Local Patch
Coding. International Journal of Signal Processing,
Image Processing and Pattern Recognition, 8(2):235–
246.
Wang, Y., Li, Y., Ji, X., and Liu, Y. (2015b). Compari-
son of Grid-Based Dense Representations for Action
Recognition. In Intelligent Robotics and Applicati-
ons, Springer International Publishing, pages 435–
444, Cham. Springer International Publishing.
Wang, Y. and Mori, G. (2010). Hidden Part Models for
Human Action Recognition: Probabilistic vs. Max-
Margin. IEEE Transactions on Pattern Analysis and
Machine Intelligence, 33(7):1310–1323.
Weinland, D., Ronfard, R., and Boyer, E. (2006). Free Vie-
wpoint Action Recognition Using Motion History Vo-
lumes. Computer Vision and Image Understanding,
104(2):249–257.
Xiao, Y., Zhao, G., Yuan, J., and Thalmann, D. (2014).
Activity Recognition in Unconstrained RGB-D Video
Using 3D Trajectories. In SIGGRAPH Asia Autono-
mous Virtual Humans and Social Robot for Telepre-
sence, pages 1–4, New York, NY, USA. ACM.
Xie, J., Jiang, S., Xie, W., and Gao, X. (2011). An efficient
global K-means clustering algorithm. JOURNAL OF
COMPUTERS (JCP), 6(2):271–279.
Xue, H., Zhang, S., and Cai, D. (2017). Depth Image In-
painting: Improving Low Rank Matrix Completion
With Low Gradient Regularization. IEEE Transacti-
ons on Image Processing, (9):4311–4320.
Yang, X. and Tian, Y. (2016). Super Normal Vector for Hu-
man Activity Recognition with Depth Cameras. IEEE
Transactions on Pattern Analysis and Machine Inteli-
gence, pages 1–12.
Yu, G., Liu, Z., and Yuan, J. (2015). Discriminative order-
let mining for real-time recognition of human-object
interaction. Lecture Notes in Computer Science (in-
cluding subseries in Artificial Intelligence and Bioin-
formatics), 9007:50–65.
Zanfir, M., Leordeanu, M., and Sminchisescu, C. (2013).
The Moving Pose: An Efficient 3D Kinematics Des-
criptor for Low-Latency Action Recognition and De-
tection. Proceedings of the IEEE International Confe-
rence on Computer Vision (ICCV), pages 2752–2759.
Zhang, H. and Parker, L. E. (2016). oDe4D: Color-Depth
Local Spatio-Temporal Features for Human Activity
Recognition from RGB-D Videos. IEEE Transacti-
ons on Circuits and Systems for Video Technology,
26(3):541–555.
Zhao, Y., Liu, Z., Yang, L., and Cheng, H. (2012). Combing
RGB and Depth Map Features for Human Activity
Recognition. In Proceedings of The Asia Pacific Sig-
nal and Information Processing Association Annual
Summit and Conference, pages 1–4.
Zhu, G., Zhang, L., Shen, P., and Song, J. (2016). An
Online Continuous Human Action Recognition Algo-
rithm Based on the Kinect Sensor. MDPI, Sensors
(Basel), 16(2):1–18.
ICPRAM 2018 - 7th International Conference on Pattern Recognition Applications and Methods
272