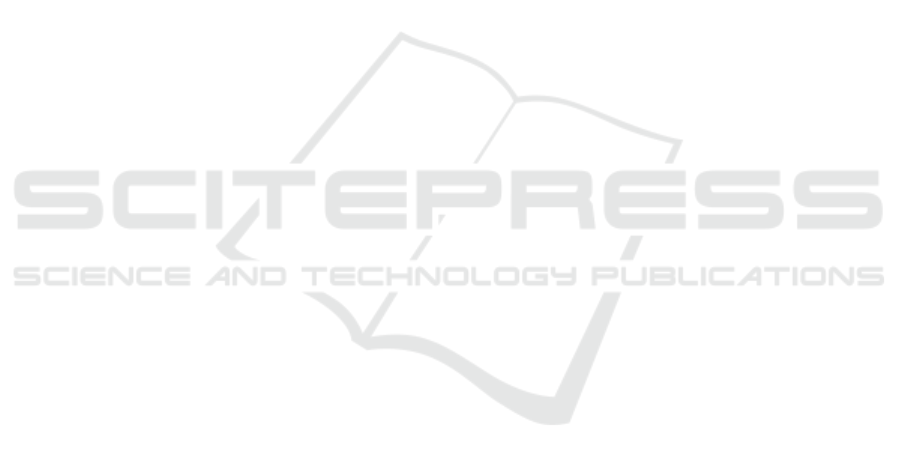
attacks. Compared with the simple pre-processing
process, the system can select the malicious traffic to
a smaller range, while ensuring the recall rate. How-
ever, it is also very fast to achieve their goals, which
is to narrow the range for further detection. Our eval-
uation uses real data from passive DNS data of pro-
vincial telecommunication at different times. Ampli-
fication attacks and random sub-domain name attacks
involved in the domain name recall rate reached
100%, DGA domain name recall rate of 90% or more.
REFERENCES
Antonakakis, M., Perdisci, R., Dagon, D., Lee, W., and
Feamster, N. (2010). Building a dynamic reputation
system for dns. InUsenix Security Symposium, Wash-
ington, Dc, Usa, August 11-13, 2010, Proceed-
ings,pages 273–290.
Antonakakis, M., Perdisci, R., Nadji, Y., Vasiloglou, N.,
Abu-Nimeh, S., Lee, W., and Dagon, D. (2012). From
throw-away traffic to bots: Detecting the rise of dga-
based malware. In Presented as part of the 21
st
USENIX
Security Symposium (USENIX Security 12), pages 491–
506, Bellevue, WA. USENIX.
Bilge, L., Kirda, E., Kruegel, C., and Balduzzi, M. (2011).
Exposure: Finding malicious domains using passive
dns analysis. InNetwork and Distributed System Secu-
rity Symposium, NDSS 2011, San Diego, California,
Usa, February - February.
Plohmann, D., Yakdan, K., Klatt, M., Bader, J., and Ger-
hards-Padilla, E. (2016). A comprehensive measure-
ment study of domain generating malware. In 25th
USENIX Security Symposium (USENIX Security 16),
pages 263–278, Austin, TX. USENIX Association.
Serdar Argic, Shane Atkinson, R. C. Dsbl. http://www.
dsbl.org/. A blocklist specialized in listing open relays
and open proxies.
Perdisci R, Corona I, Giacinto G. Early detection of mali-
cious flux networks via large-scale passive DNS traffic
analysis. IEEE Transactions on Dependable and Se-
cure Computing, 2012, 9(5): 714–726.
Woodbridge J, Anderson H S, Ahuja A, et al.(2016). Pre-
dicting Domain Generation Algorithms with Long
Short-Term Memory Networks[J]..
Goldberg, Y., & Levy, O. (2014). Word2vec explained: de-
riving mikolov et al.'s negative-sampling word-embed-
ding method. Eprint Arxiv.
Thomas Orthbandt. (2015). Nominum. https://nomi-
num.com/press_item/sharp-rise-in-dns-based-ddos-
last-year-signals-larger-more-frequent-attacks-in-
2015/. DNS-based DDoS rise signals more attacks in
2015.
Tama, B. A., & Rhee, K. H. (2015). Data mining techniques
in DoS/DDoS attack detection: A literature review. In-
ternational Information Institute (Tokyo). Information,
18(8), 3739.
Karnwal, T., Sivakumar, T., & Aghila, G. (2013). A comber
approach to protect cloud computing against xml ddos
and http ddos attack, 182, 1-5.
Wang, B., Zheng, Y., Lou, W., & Hou, Y. T. (2015). Ddos
attack protection in the era of cloud computing and soft-
ware-defined networking. Computer Networks, 81(C),
308-319.
Eduard Kovacs. (2014). Large DNS Text Records Used to
Amplify DDoS Attacks: Akamai. http://www.securi-
tyweek.com/large-dns-text-records-used-amplify-
ddos-attacks-akamai.
C. Liu. A new kind of ddos threat: The â ˘ AIJnonsense
nameâ ˘ IA˙ attack. Network World, 2015. [Online;
posted 27-January-2015]
VeriSign, Verisign distributed denial of service trends re-
port q4 2015. https://www.verisign.com/assets/report-
ddos-trends-Q42015.pdf, 2015.
Rizzo, G., Van Erp, M., Plu, J., & Troncy, R. (2016). Mak-
ing sense of microposts (#Microposts2016) named en-
tity recognition and linking (NEEL) challenge. CEUR
Workshop Proceedings, 1691, 50–59. https://doi.org/
10.1145/1235.
Andrew. (2015). https://secure64.com/defenses-pseudo-
random-subdomain-attacks-prsd/. More Defenses
Against Pseudo Random Subdomain attacks (PRSD).
S. Stover, D. Dittrich, J. Hernandez, and S. Diet- rich. Anal-
ysis of the storm and nugache trojans: P2P is here. In
USENIX ;login:, vol. 32, no. 6, December 2007.
Wikipedia. The storm botnet. http://en. wikipe-
dia.org/wiki/Storm_botnet, 2010.
J.Williams. What we know (and learned) from the waledac
takedown. http://tinyurl.com/ 7apnn9b, 2010.
abuse.ch. ZeuS Gets More Sophisticated Using P2P Tech-
niques. http://www.abuse.ch/ ?p=3499, 2011.
Rahbarinia, B. (2016). Segugio:Efficient and Accurate Be-
havior-Based Tracking of Malware-Control Domains
in Large ISP Networks, 19(2). https://doi.org/
10.1145/2960409.
Yadav, S., Reddy, A. K. K., Reddy, A. N., and Ranjan, S.
Detecting Algorithmically Generated Malicious Do-
main Names. In Proceedings of the 10th ACM
SIGCOMM Conference on Internet Measurement
(2010), IMC ’10.
ICISSP 2018 - 4th International Conference on Information Systems Security and Privacy
198