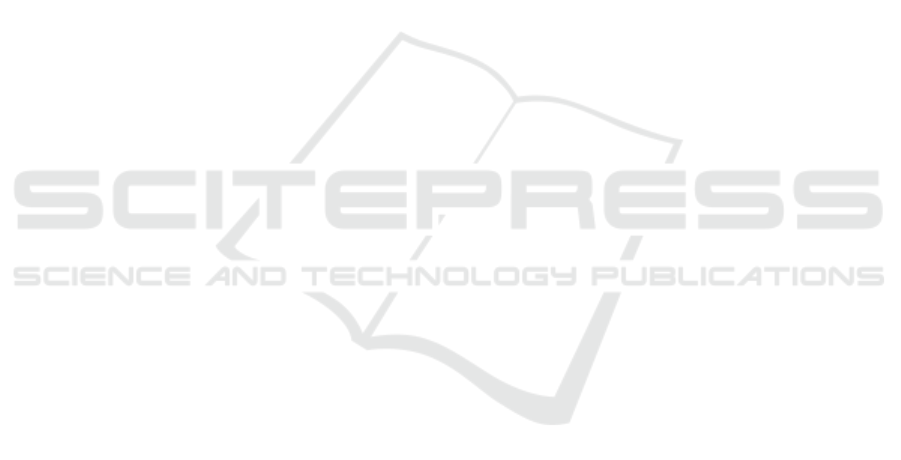
decentralized predictive scheduling for providing en-
ergy services in future smart grid architectures with-
out a need for an (expensive) individual link of each
single device in the ensemble.
REFERENCES
Abarrategui, O., Marti, J., and Gonzalez, A. (2009). Con-
structing the active european power grid. In Proceed-
ings of WCPEE09, Cairo.
Awerbuch, S. and Preston, A. M., editors (1997). The Vir-
tual Utility: Accounting, Technology & Competitive
Aspects of the Emerging Industry, volume 26 of Top-
ics in Regulatory Economics and Policy. Kluwer Aca-
demic Publishers.
Bremer, J. and Lehnhoff, S. (2017). Hybrid Multi-ensemble
Scheduling, pages 342–358. Springer International
Publishing, Cham.
Bremer, J., Rapp, B., and Sonnenschein, M. (2011). Encod-
ing distributed search spaces for virtual power plants.
In IEEE Symposium Series on Computational Intelli-
gence 2011 (SSCI 2011), Paris, France.
Bremer, J. and Sonnenschein, M. (2013a). Constraint-
handling for optimization with support vector sur-
rogate models. In Filipe, J. and Fred, A., editors,
ICAART 2013 – Proceedings of the 5th International
Conference on Agents and Artificial Intelligence, vol-
ume 2, pages 91–105, Barcelona, Spain. SciTePress.
Bremer, J. and Sonnenschein, M. (2013b). Model-based in-
tegration of constrained search spaces into distributed
planning of active power provision. Comput. Sci. Inf.
Syst., 10(4):1823–1854.
Bremer, J. and Sonnenschein, M. (2013c). Sampling the
search space of energy resources for self-organized,
agent-based planning of active power provision. In
Page, B., Fleischer, A. G., G
¨
obel, J., and Wohlgemuth,
V., editors, 27th International Conference on Environ-
mental Informatics for Environmental Protection, En-
viroInfo 2013, pages 214–222. Shaker.
Coello Coello, C. A. (2002). Theoretical and numerical
constraint-handling techniques used with evolutionary
algorithms: a survey of the state of the art. Com-
puter Methods in Applied Mechanics and Engineer-
ing, 191(11-12):1245–1287.
Duckham, M., Kulik, L., Worboys, M., and Galton, A.
(2008). Efficient generation of simple polygons for
characterizing the shape of a set of points in the plane.
Pattern Recognition, 41(10):3224 – 3236.
European Parliament & Council (2009). Directive
2009/28/ec of 23 april 2009 on the promotion of the
use of energy from renewable sources and amending
and subsequently repealing directives 2001/77/ec and
2003/30/ec.
Gieseke, F. and Kramer, O. (2013). Towards non-linear
constraint estimation for expensive optimization. In
Esparcia-Alc
´
azar, A., editor, Applications of Evolu-
tionary Computation, volume 7835 of LNCS, pages
459–468. Springer Berlin Heidelberg.
Hall, P. (1927). The distribution of means for samples of
size n drawn from a population in which the variate
takes values between 0 and 1, all such values being
equally probable. Biometrika, 19(3/4):pp. 240–245.
Hinrichs, C. (2014). Selbstorganisierte Einsatzplanung
dezentraler Akteure im Smart Grid. PhD thesis, Carl
von Ossietzky Universitt Oldenburg.
Hinrichs, C., Bremer, J., and Sonnenschein, M. (2013). Dis-
tributed Hybrid Constraint Handling in Large Scale
Virtual Power Plants. In IEEE PES Conference on In-
novative Smart Grid Technologies Europe (ISGT Eu-
rope 2013). IEEE Power & Energy Society.
Kamphuis, R., Warmer, C., Hommelberg, M., and Kok, K.
(2007). Massive coordination of dispersed generation
using powermatcher based software agents. In 19th
International Conference on Electricity Distribution.
Kirkpatrick, S., Gelatt, C. D., and Vecchi, M. P. (1983).
Optimization by simulated annealing. Science,
220(4598):671–680.
Kramer, O. (2010). A review of constraint-handling tech-
niques for evolution strategies. Appl. Comp. Intell.
Soft Comput., 2010:1–19.
Li, Y., Protopopescu, V. A., Arnold, N., Zhang, X., and
Gorin, A. (2009). Hybrid parallel tempering and sim-
ulated annealing method. Applied Mathematics and
Computation, 212(1):216–228.
McArthur, S., Davidson, E., Catterson, V., Dimeas, A.,
Hatziargyriou, N., Ponci, F., and Funabashi, T. (2007).
Multi-agent systems for power engineering applica-
tions – Part I: Concepts, approaches, and technical
challenges. IEEE Transactions on Power Systems,
22(4):1743–1752.
Nieße, A., Lehnhoff, S., Tr
¨
oschel, M., Uslar, M., Wiss-
ing, C., Appelrath, H.-J., and Sonnenschein, M.
(2012). Market–based self–organized provision of ac-
tive power and ancillary services. IEEE.
Padhy, N. (2004). Unit Commitment - A Bibliographi-
cal Survey. IEEE Transactions on Power Systems,
19(2):1196–1205.
Pinto, R., Bessa, R. J., and Matos, M. A. (2017). Surrogate
model of multi-period flexibility from a home energy
management system. CoRR, abs/1703.08825.
Ramchurn, S. D., Vytelingum, P., Rogers, A., and Jennings,
N. R. (2011). Agent-based homeostatic control for
green energy in the smart grid. ACM Trans. Intell.
Syst. Technol., 2(4):35:1–35:28.
Schiendorfer, A., Stegh
¨
ofer, J.-P., and Reif, W. (2014). Syn-
thesised constraint models for distributed energy man-
agement. In Ganzha, M., Maciaszek, L. A., and Pa-
przycki, M., editors, Proceedings of the Federated
Conference on Computer Science and Information
Systems, pages 1529–1538, Warsaw, Poland.
Smith, A. and Coit, D. (1997). Handbook of Evolutionary
Computation, chapter Penalty Functions, page Section
C5.2. Oxford University Press and IOP Publishing,
Department of Industrial Engineering, University of
Pittsburgh, USA.
Sonnenschein, M., L
¨
unsdorf, O., Bremer, J., and Tr
¨
oschel,
M. (2014). Decentralized control of units in smart
grids for the support of renewable energy supply. En-
vironmental Impact Assessment Review, (0):–.
Tax, D. M. J. and Duin, R. P. W. (2004). Support vector data
description. Mach. Learn., 54(1):45–66.
Unfolding Ensemble Training Sets for Improved Support Vector Decoders in Energy Management
329