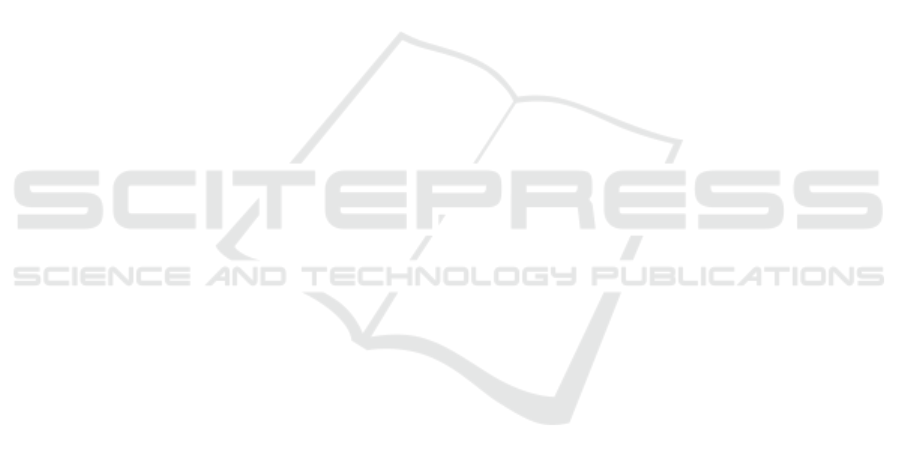
6 CONCLUSION
We propose and analyze a multi-item LUBA game
with budget constraint, registration fee and resubmis-
sion cost. We show that the analysis can be reduced
into a finite game (with incomplete information) by
eliminating the bids that are higher than the value of
the item or by the bid that are higher the total available
budget. Using classical fixed-point theorem, there is
at least one Bayes-Nash equilibrium in mixed strate-
gies. Next, we address the question of computation
and stability of such an equilibrium. We provide ex-
plicitly the equilibrium structure in simple cases. In
the general setting, we provide a learning algorithm
that is able to locate equilibria. We propose an im-
itative combined fully distributed payoff and strat-
egy learning (imitative CODI- PAS learning) that is
adapted to LUBA. We examine how the bidders of the
game are able to learn about the online system output
using their own-independent learning strategies and
own-independent valuation. The revenue of the auc-
tionner is explicitly derived in a situation where a ran-
dom number of bids are placed.
REFERENCES
Vickrey, W.: Counterspeculation, auctions, and competitive
sealed tenders. J. Finance 16, 837.1961.
Maskin E. S., Riley J. G. Asymmetric auctions. Rev.
Econom. Stud. 67, 413-438, 2000.
Lebrun B: First-price auctions in the asymmetric n bid-
der case. International Economic Review 40:125-142,
(1999)
Lebrun B.: Uniqueness of the equilibrium in first-price
auctions. Games and Economic Behavior 55:131-151,
2006.
Myerson R., Optimal Auction Design, Mathematics of Op-
erations Research, 6 (1981),pp. 58-73.
Bang-Qing Li, Jian-Chao Zeng, Meng Wang and Gui-Mei
Xia, A negotiation model through multi-item auction
in multi-agent system, Machine Learning and Cyber-
netics, 2003 International Conference on, 2003, pp.
1866-1870 Vol.3.
C. Yi and J. Cai, Multi-Item Spectrum Auction for Recall-
Based Cognitive Radio Networks With Multiple Het-
erogeneous Secondary Users, in IEEE Transactions
on Vehicular Technology, vol. 64, no. 2, pp. 781-792,
Feb. 2015.
N. Wang and D. Wang, Model and algorithm of winner
determination problem in multi-item E-procurement
with variable quantities,” The 26th Chinese Control
and Decision Conference (2014 CCDC), Changsha,
2014, pp. 5364-5367.
Rituraj and A. K. Jagannatham, Optimal cluster head se-
lection schemes for hierarchical OFDMA based video
sensor networks,” Wireless and Mobile Networking
Conference (WMNC), 2013 6th Joint IFIP, Dubai,
2013, pp. 1-6.
J. Zhao, X. Chu, H. Liu, Y. W. Leung and Z. Li, Online
procurement auctions for resource pooling in client-
assisted cloud storage systems, IEEE Conference on
Computer Communications (INFOCOM), 2015, pp.
576-584.
R. Zhou, Z. Li and C. Wu, An online procurement auc-
tion for power demand response in storage-assisted
smart grids, IEEE Conference on Computer Commu-
nications, INFOCOM, 2015, pp. 2641-2649.
H. Houba, D. Laan, D. Veldhuizen, Endogenous entry in
lowest-unique sealed-bid auctions,Theory and Deci-
sion, 71, 2, 2011, pp. 269-295
Stefan De Wachter and T. Norman, The predictive power
of Nash equilibrium in difficult games: an empirical
analysis of minbid games, Department of Economics
at the University of Bergen, 2006
Rapoport, Amnon and Otsubo, Hironori and Kim, Bora
and Stein, William E.: Unique bid auctions: Equi-
librium solutions and experimental evidence, MPRA
Paper 4185, University Library of Munich, Germany,
Jul 2007.
J.Eichberger and D. Vinogradov, Least Unmatched Price
Auctions : A First Approach, Discussion Paper Series
471, 2008
J. Eichberger, Dmitri Vinogradov: Lowest-Unmatched
Price Auctions, International Journal of Industrial Or-
ganization, 2015, Vol. 43, pp. 1-17
J. Eichberger, Dmitri Vinogradov: Efficiency of Lowest-
Unmatched Price Auctions, Economics Letters, 2016,
Vol. 141, pp. 98?102
Erik Mohlin, Robert Ostling, Joseph Tao-yi Wang, Lowest
unique bid auctions with population uncertainty, Eco-
nomics Letters, Vol. 134, Sept. 2015, Pages 53-57.
Huang, G.Q., Xu, S.X., 2013. Truthful multi-unit
transportation procurement auctions for logistics e-
marketplaces. Transport. Res. Part B: Methodol. 47,
127-148.
Dong-Her Shih, David C. Yen, Chih-Hung Cheng, Ming-
Hung Shih, A secure multi-item e-auction mechanism
with bid privacy, Computers and Security 30 (2011)
273-287
Tembine, H., Distributed strategic learning for wireless en-
gineers, Master Course, CRC Press, Taylor & Francis,
2012.
Harsanyi, J. 1973. Games with randomly disturbed payoffs:
A new rationale for mixed strategy equilibrium points.
Internat. J. Game Theory 2, 1-23.
Weibull, J., Evolutionary game theory, MIT Press, 1995.
PROOFS
Proof of Proposition 2. By budget constraint, j
0
s
bids must fulfill
∑
i
b
ji
≤
¯
b
j
. If b
i j
> v
ji
− ˜c, j gets
v
ji
− ˜c−b
ji
which is negative (loss), and j could guar-
antee zero by not participating. Therefore the strategy
0 dominates any b
ji
higher than v
ji
− ˜c.
ICAART 2018 - 10th International Conference on Agents and Artificial Intelligence
336