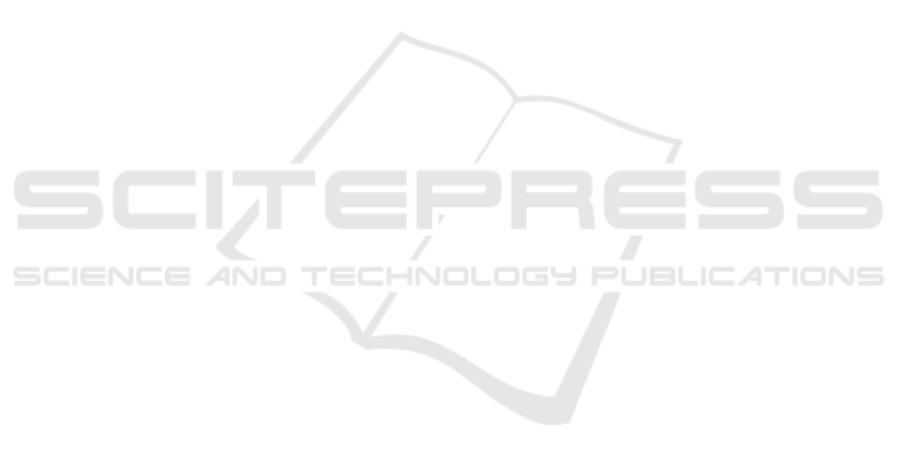
A non-aggregated information is rather different from
the true value hints in the Bestr scenario and the most
popular interval in the Bin scenario. However the rea-
sons for it being not perceptible to the point of the re-
sults being similar to a no-information scenario need
further investigation. It may happen that the amount
of data becomes simply too large for the participants
to integrate and use as a meaningful hint. They need
to look at and infer mean, medium, extreme values
and other statistical measurements. Maybe the bonus
payment to the Mechanical Turk workers did not re-
ward the extra cognitive effort required to obtain the
hint. Consequently, workers may have ignored the
hint and act as if no information was displayed. We
plan to repeat this experiment varying the amount of
payment to confirm this. Also, a textual form of the
previous estimates can replace the graphic to evalu-
ate if the form of representation plays a role in the
influence. Finally we want to assess the power of the
metrics in detecting influence as soon as possible in
the data stream. This will be important to enable the
expansion of CI experiments, since an early detection
of influence may prevent unnecessary time and costs,
leading to an improved redesign of the experiment.
REFERENCES
Amirat, A. and Bouri, A. (2009). Modeling informational
cascade via behavior biases. Global Economy & Fi-
nance Journal, 2(2):81–103.
Annas, G. J. (2003). Hipaa regulations-a new era of
medical-record privacy? New England Journal of
Medicine, 348(15):1486–1490.
Avery, C., Resnick, P., and Zeckhauser, R. (1999). The
market for evaluations. American Economic Review,
pages 564–584.
Banerjee, A. V. (1992). A simple model of herd behav-
ior. The Quarterly Journal of Economics, 107(3):797–
817.
Bansal, G., Gefen, D., et al. (2010). The impact of personal
dispositions on information sensitivity, privacy con-
cern and trust in disclosing health information online.
Decision support systems, 49(2):138–150.
Barreto, I. and Baden-Fuller, C. (2006). To conform or
to perform? mimetic behaviour, legitimacy-based
groups and performance consequences. Journal of
Management Studies, 43(7):1559–1581.
Berg, J. E. and Rietz, T. A. (2003). Prediction markets as
decision support systems. Information systems fron-
tiers, 5(1):79–93.
Bikhchandani, S., Hirshleifer, D., and Welch, I. (1998).
Learning from the behavior of others: Conformity,
fads, and informational cascades. The Journal of Eco-
nomic Perspectives, 12(3):151–170.
Bikhchandani, S. and Sharma, S. (2000). Herd behavior in
financial markets. IMF Staff papers, pages 279–310.
Chevalier, J. A. and Mayzlin, D. (2006). The effect of word
of mouth on sales: Online book reviews. Journal of
marketing research, 43(3):345–354.
Christie, W. G. and Huang, R. D. (1995). Following the pied
piper: Do individual returns herd around the market?
Financial Analysts Journal, 51(4):31–37.
Curtis, V. (2015). Motivation to participate in an online
citizen science game: A study of foldit. Science Com-
munication, 37(6):723–746.
Duflo, E. and Saez, E. (2002). Participation and invest-
ment decisions in a retirement plan: The influence
of colleagues’ choices. Journal of public Economics,
85(1):121–148.
Gostin, L. O. and Hodge Jr, J. G. (2001). Personal privacy
and common goods: a framework for balancing under
the national health information privacy rule. Minn. L.
Rev., 86:1439.
Kelly, M. and Gráda, C. Ó. (2000). Market contagion: Ev-
idence from the panics of 1854 and 1857. American
Economic Review, pages 1110–1124.
Kempe, D., Kleinberg, J., and Tardos, É. (2003). Maximiz-
ing the spread of influence through a social network.
In Proceedings of the ninth ACM SIGKDD interna-
tional conference on Knowledge discovery and data
mining, pages 137–146. ACM.
King, A. J., Cheng, L., Starke, S. D., and Myatt, J. P. (2012).
Is the true ‘wisdom of the crowd’to copy successful
individuals? Biology Letters, 8(2):197–200.
Kiss, Á. and Simonovits, G. (2014). Identifying the band-
wagon effect in two-round elections. Public Choice,
160(3-4):327–344.
Lakonishok, J., Shleifer, A., and Vishny, R. W. (1992). The
impact of institutional trading on stock prices. Journal
of financial economics, 32(1):23–43.
Lorenz, J., Rauhut, H., Schweitzer, F., and Helbing, D.
(2011). How social influence can undermine the wis-
dom of crowd effect. Proceedings of the National
Academy of Sciences, 108(22):9020–9025.
Malone, T. W., Laubacher, R., and Dellarocas, C. (2009).
Harnessing crowds: Mapping the genome of collec-
tive intelligence. Technical report, MIT Sloan Re-
search Paper 4732-09.
Marshall, R. C. and Meurer, M. J. (2004). Bidder collusion
and antitrust law: refining the analysis of price fixing
to account for the special features of auction markets.
Antitrust Law Journal, 72(1):83–118.
Muchnik, L., Aral, S., and Taylor, S. J. (2013). Social
influence bias: A randomized experiment. Science,
341(6146):647–651.
Park, A. and Sgroi, D. (2012). Herding, contrarianism and
delay in financial market trading. European Economic
Review, 56(6):1020–1037.
Pathak, B., Garfinkel, R., Gopal, R. D., Venkatesan, R., and
Yin, F. (2010). Empirical analysis of the impact of rec-
ommender systems on sales. Journal of Management
Information Systems, 27(2):159–188.
Raafat, R. M., Chater, N., and Frith, C. (2009). Herding
in humans. Trends in cognitive sciences, 13(10):420–
428.
Detecting Influence in Wisdom of the Crowds
23