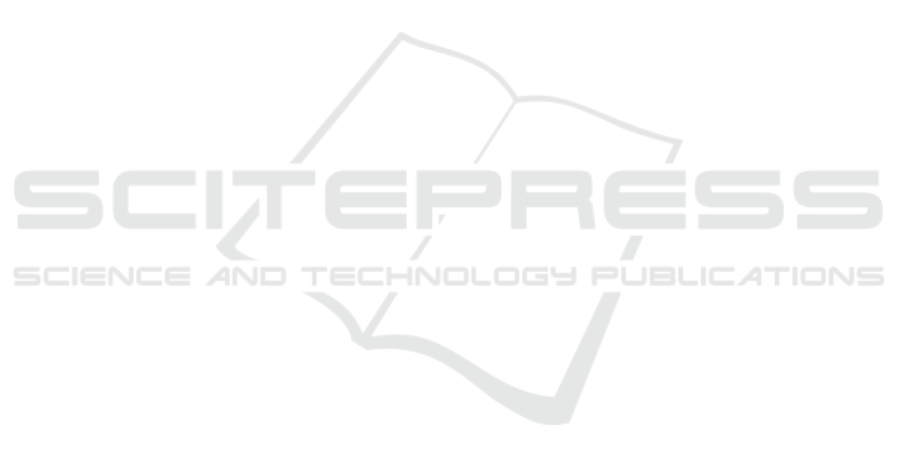
Beamon, B. M. , 1999. Measuring supply chain
performance. International Journal of Operations and
Production Management, 19(3), 275-292.
Bentler, P. M., and Chou, C.-P., 1987. Practical Issues in
Structural Modeling. Sociological Methods Research,
16(1), 78-117.
Bhagwat, R., and Sharma, M. K., 2007. Performance
measurement of supply chain management: A balanced
scorecard approach. Computers and Industrial
Engineering, 53(1), 43-62.
Blodgett, J. G., and Anderson, R. D., 2000. A Bayesian
network model of the consumer compliant process.
Journal of Service Research, 2(4), 321-338.
Boon-itt, S., and Himangshu, P., 2005. Measuring Supply
Chain Integration Using the Q-Sort Technique. In
Research Methodologies in Supply Chain Management
(pp. 47-58). Heidelberg: Physica-Verlag.
Chae, B., 2009. Developing key performance indicators for
supply chain: an industry perspective. Supply Chain
Management: An International Journal, 14(6), 422-
428.
Davis-Sramek, B., Germain, R., and Karthik, l., 2010.
Supply chain technology: the role of environment in
predicting performance. Journal of the Academy of
Marketing Science(38), 42-55.
Devaraj, S., Krajewski, L., and Wei, J. C., 2007. Impact of
eBusiness technologies on operational performance:
The role of production information integration in the
supply chain. Journal of Operations Management,
25(6), 1199-1216.
Dubois, D., and Prade, H., 1983. Ranking fuzzy numbers in
the seting of possibility theory. Information Sciences,
30(3), 183-224.
Fornell, C., and Larcker, D. F., 1981. Evaluating structural
equation models with unobservable variables and
measurement error. Journal of Marketing Research,
18(1), 39-50.
Frohlich, M. T., and Westbrook, R., 2001. Arcs of
integration: an international study of supply chain
strategies. Journal of Operations Management, 19(2),
185-200.
González-Benito, J., 2007. Information technology
investment and operational performance in purchasing
The mediating role of supply chain management
practices and strategic integration of purchasing.
Industrial Management and Data Systems, 107(2), 201-
228.
Hamister, J. W., 2012. Supply chain management practices
in small retailers. International Journal of Retail and
Distribution Management, 40(6), 427-450.
Heckerman, D., 1997. Bayasian networks for data mining.
Data Mining and Knowledge Discovery, 1(1), 79-119.
Hsu, C. C., Tan, K. C., Kannan, V. R., and Keong Leong,
G., 2009. Supply chain management practices as a
mediator of the relationship between operations
capability and firm performance. International Journal
of Production Research, 47(3), 835-855.
Hu, L.-t., and Bentler, P. M., 1999. Cutoff criteria for fit
indexes in covariance structure analysis: Conventional
criteria versus new alternatives. Structural Equation
Modeling: A Multidisciplinary Journal, 6(1), 1-55.
Ibrahim, S. E., and Ogunyemi, O., 2012. The effect of
linkages and information sharing on supply chain and
export performance: An empirical study of Egyptian
textile manufacturers. Journal of Manufacturing
Technology Management, 23(4), 441-463.
Johnson, P. F., Klassen, ,. R., Leenders, M. R., and
Awaysheh, A., 2007. Utilizing e-business technologies
in supply chains: The impact of firm characteristics and
teams. Journal of Operations Management, 25(6),
1255-1274.
Kim, D.-Y., Kumar, V., and Kumar, U., 2012. Relationship
between quality management practices and innovation.
Journal of Operations Management, 30(4), 295–315.
King, G., Honaker, J., Joseph, A., and Scheve, K., 2001.
Analyzing Incomplete Political Science Data: An
Alternative Algorithm for Multiple Imputation.
American Political Science Review, 95(1), 49-69.
Kosko, B., 1986. Fuzzy cognitive maps. International
Journal of Man-Machine Studies, 24(1), 65-75.
Landsheer, J. L., 2010. The Specification of Causal Models
with Tetrad IV: A Review. Structural Equation
Modeling: A Multidisciplinary Journal, 17(4), 703-711.
Lee, C. W., Kwon, I.-W. G., and Severance, D., 2007.
Relationship between supply chain performance and
degree of linkage among supplier, internal integration,
and customer. Supply Chain Management: An
International Journal, 12(6), 444-452.
Li, G., Yang, H., Sun, L., and Sohal, A. S. , 2009. The
impact of IT implementation on supply chain
integration and performance. Intnational Journal of
Production Economics, 120(1), 125-138.
Li, S., Rao, S. S., Ragu-Nathan, T. S., and Ragu-Nathan, B.,
2005. Development and validation of a measurement
instrument for studying supply chain management
practices. Journal of Operations Management, 23, 618-
641.
Lockamy III, A., and McCormack, K., 2004. Linking
SCOR planning practices to supply chain performance:
An exploratory study. International Journal of
Operations and Production Management, 24(12),
1192-1218.
MacKenzie, S. B., and Podsakoff, P. M., 2011. Construct
measurement and validation procedures in mis and
behavioral research: integrating new and existing
techniques. MIS Quarterly, 35(2), 293-334.
McColl-Kennedy, J. R., and Anderson, R. D., 2005.
Subordinate-manager gender combination and
perceived leadership style influence on emotions, self-
esteem and organizational commitment. Journal of
Business Research, 58, 115-125.
Menhaj, M. B., 2006. Fuzzy computations. Tehran, Iran:
Amirkabir University of Technology.
Narasimhan, R., and Jayanth, J., 1998. Causal Linkages in
Supply Chain Management: An Exploratory Study of
North American Manufacturing Firms. Decision
Sciences, 29(3), 579-605.
Integrating Fuzzy Cognitive Mapping and Bayesian Network Learning for Supply Chain Causal Modeling
69