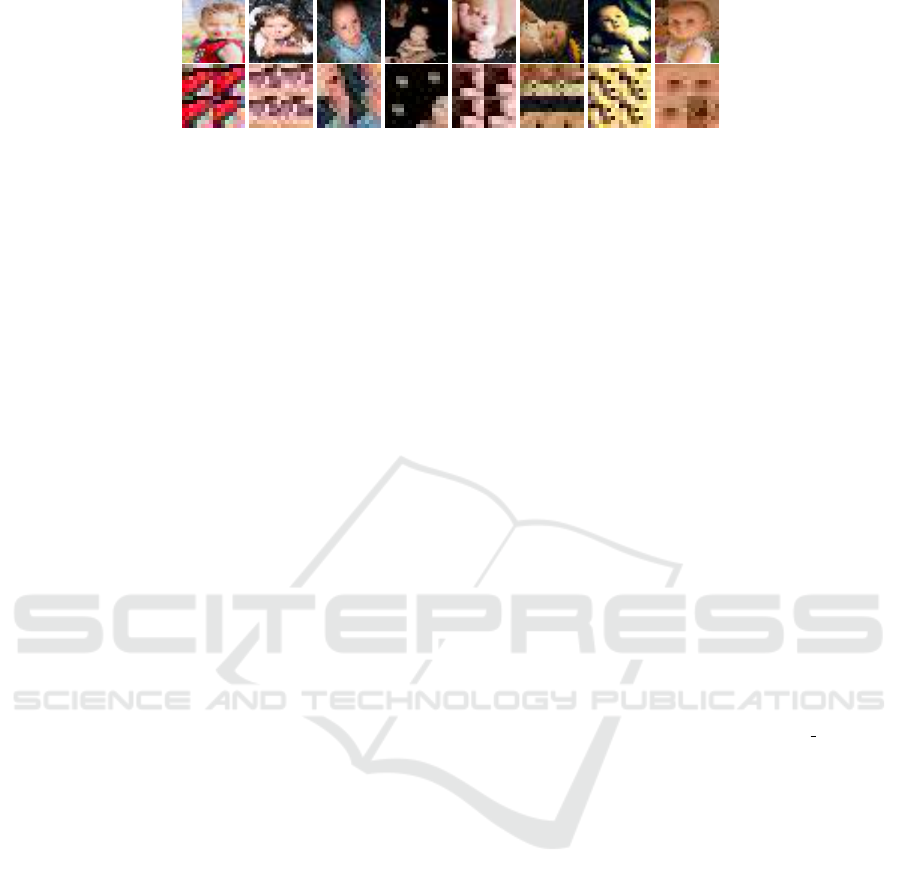
Figure 6: This figure shows eight example images from the ANP “amazing baby” in the upper row. The lower row shows
their corresponding images as they are created by the patches method. (The patched image belongs to the image above it).
This highlights the problem of the method to create those patched images. Namely that it is not capable of selecting distinct
areas but instead very similar ones are used.
categories, “Normal”, “Same Adjective” and “Same
Noun”. We found out that our deeper network showed
some slight improvements by incorporating saliency
data using the alpha value in all three categories.
But these improvements were not statistically signif-
icant besides for the case of “Same Noun” with the
technique of “Alpha Value (Binarized)”. But we ex-
pect better improvements with a bigger dataset, where
each class contains more images. A huge dataset
which satisfies all the needs, e.g. the support of mul-
tiple ANPs and synonyms, for this problem. Creating
such a dataset is a difficult and interesting problem
for future work. Furthermore our results are showing
big differences between the different the two CNNs,
which we used. Therefore the impact of different net-
work architectures can also be investigated in future
work to maximize the precision of the approach.
REFERENCES
Al-Naser, M., Chanijani, S. S. M., Bukhari, S. S., Borth,
D., and Dengel, A. (2015). What makes a beautiful
landscape beautiful: Adjective noun pairs attention by
eye-tracking and gaze analysis. In Proceedings of the
1st International Workshop on Affect & Sentiment in
Multimedia, pages 51–56. ACM.
Borth, D., Ji, R., Chen, T., Breuel, T., and Chang, S.-F.
(2013). Large-scale visual sentiment ontology and de-
tectors using adjective noun pairs. In Proceedings of
the 21st ACM international conference on Multime-
dia, pages 223–232. ACM.
Cao, D., Ji, R., Lin, D., and Li, S. (2016). A cross-media
public sentiment analysis system for microblog. Mul-
timedia Systems, 22(4):479–486.
Chen, T., Borth, D., Darrell, T., and Chang, S.-F. (2014a).
Deepsentibank: Visual sentiment concept classifica-
tion with deep convolutional neural networks. arXiv
preprint arXiv:1410.8586.
Chen, T., Yu, F. X., Chen, J., Cui, Y., Chen, Y.-Y., and
Chang, S.-F. (2014b). Object-based visual sentiment
concept analysis and application. In Proceedings of
the 22nd ACM international conference on Multime-
dia, pages 367–376. ACM.
Harel, J., Koch, C., and Perona, P. (2006). Graph-based vi-
sual saliency. In Advances in neural information pro-
cessing systems, pages 545–552.
Jou, B., Chen, T., Pappas, N., Redi, M., Topkara, M., and
Chang, S.-F. (2015). Visual affect around the world:
A large-scale multilingual visual sentiment ontology.
In Proceedings of the 23rd ACM international confer-
ence on Multimedia, pages 159–168. ACM.
Krizhevsky, A. and Hinton, G. (2009). Learning multiple
layers of features from tiny images.
Otsu, N. (1975). A threshold selection method from gray-
level histograms. Automatica, 11(285-296):23–27.
Plutchik, R. (1980). Emotion: A psychoevolutionary syn-
thesis. Harpercollins College Division.
Russakovsky, O., Deng, J., Su, H., Krause, J., Satheesh,
S., Ma, S., Huang, Z., Karpathy, A., Khosla, A.,
Bernstein, M., Berg, A. C., and Fei-Fei, L. (2015).
ImageNet Large Scale Visual Recognition Challenge.
International Journal of Computer Vision (IJCV),
115(3):211–252.
Stricker, M., Bukhari, S. S., Al-Naser, M., Mozafari, S.,
Borth, D., and Dengel, A. (2017). Which saliency
detection method is the best to estimate the human at-
tention for adjective noun concepts?. In ICAART (2),
pages 185–195.
Tensorflow (2017). Neural network proposed by tensorflow.
https://www.tensorflow.org/tutorials/deep cnn.
Vadicamo, L., Carrara, F., Cimino, A., Cresci, S., DellOr-
letta, F., Falchi, F., and Tesconi, M. (2017). Cross-
media learning for image sentiment analysis in the
wild. In Proceedings of the IEEE Conference on Com-
puter Vision and Pattern Recognition, pages 308–317.
You, Q., Luo, J., Jin, H., and Yang, J. (2016). Cross-
modality consistent regression for joint visual-textual
sentiment analysis of social multimedia. In Proceed-
ings of the Ninth ACM International Conference on
Web Search and Data Mining, pages 13–22. ACM.
ICAART 2018 - 10th International Conference on Agents and Artificial Intelligence
394