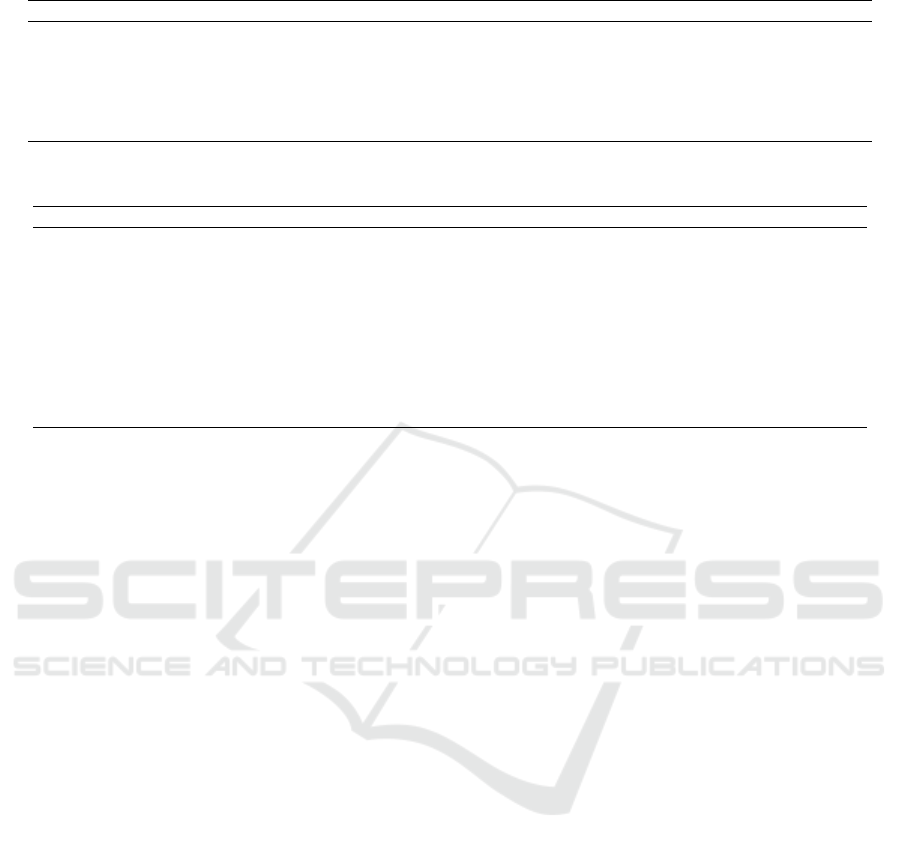
Table 12: Examples for sentiment assignment.
Reference Human System
However, [7] only focuses the disguise handwriting and this does not completely suffice . Neg Neg
The most recent work in [12] achieves an accuracy of 61.9% which is much lower Neg Neg
These preprocessing steps have been described in greater detail in our prior work[7]. Pos Neu
The recent state-of-the-art of signature verification is summarized in [3]. Pos Pos
experiments with a context independent HMM-based system that uses a sliding window [4]. Neu Neu
In [12], authors propose an SVM based active learning that utilizes the support vectors . Neu Pos
Table 13: Examples for nature assignment.
Reference Human System
In the second configuration (SWT), the Stroke Width Transform [2] was used. Usage Usage
The first data set used in this thesis is the IAM database (IAMDB) 1 [10]. Dataset Dataset
For more details on the HMMs and the spotting system, we refer to [9]. Reading Reading
[1] E. Lleida et al., Out-of-vocabulary word modeling and rejection for keyword spotting, Reference Reference
On the other hand, forward-backward computing time is negligible with respect to that of CL generation [7]. Rest Rest
In this approach, as presented in [3], character HMMs are used to build both a filler model. Usage Usage
For the standard ICDAR 2011 dataset [14], the proposed method achieves state-of-the-art results in text localization. Dataset Rest
See [7], [3] for details about the meta-parameters of line-image preprocessing, feature extraction and HMMs. Reading Reading
[10] V. Romero et al., Computer assisted transcript. Reference Reference
More recently, the same basic idea has also been used for KWS in handwriting images [3], [4]. Rest Reading
ference of the North American chapter of the Associa-
tion for Computational Linguistics: Human language
technologies, pages 597–601. Association for Compu-
tational Linguistics.
Bahrainian, S.-A. and Dengel, A. (2013). Sentiment anal-
ysis and summarization of twitter data. In Compu-
tational Science and Engineering (CSE), 2013 IEEE
16th International Conference on, pages 227–234.
IEEE.
Di Iorio, A., Nuzzolese, A. G., and Peroni, S. (2013). To-
wards the automatic identification of the nature of ci-
tations. In SePublica, pages 63–74.
Ding, Y., Zhang, G., Chambers, T., Song, M., Wang, X.,
and Zhai, C. (2014). Content-based citation analysis:
The next generation of citation analysis. Journal of the
Association for Information Science and Technology,
65(9):1820–1833.
Godbole, N., Srinivasaiah, M., and Skiena, S. (2007).
Large-scale sentiment analysis for news and blogs.
ICWSM, 7(21):219–222.
Kouloumpis, E., Wilson, T., and Moore, J. D. (2011). Twit-
ter sentiment analysis: The good the bad and the omg!
Icwsm, 11:538–541.
Ma, Z., Nam, J., and Weihe, K. (2016). Improve sentiment
analysis of citations with author modelling. In Pro-
ceedings of NAACL-HLT, pages 122–127.
Mohammad, S., Kiritchenko, S., Sobhani, P., Zhu, X.-D.,
and Cherry, C. (2016). Semeval-2016 task 6: De-
tecting stance in tweets. In SemEval@ NAACL-HLT,
pages 31–41.
Pak, A. and Paroubek, P. (2010). Twitter as a corpus for
sentiment analysis and opinion mining. In LREC, vol-
ume 10, pages 1320–1326.
Pang, B. and Lee, L. (2004). A sentimental education:
Sentiment analysis using subjectivity summarization
based on minimum cuts. In Proceedings of the 42nd
annual meeting on Association for Computational
Linguistics, page 271. Association for Computational
Linguistics.
Wan, X. and Liu, F. (2014). Are all literature citations
equally important? automatic citation strength esti-
mation and its applications. Journal of the Association
for Information Science and Technology, 65(9):1929–
1938.
Whitelaw, C., Garg, N., and Argamon, S. (2005). Using ap-
praisal groups for sentiment analysis. In Proceedings
of the 14th ACM international conference on Infor-
mation and knowledge management, pages 625–631.
ACM.
Wu, Z., Rao, Y., Li, X., Li, J., Xie, H., and Wang, F. L.
(2015). Sentiment detection of short text via prob-
abilistic topic modeling. In International Confer-
ence on Database Systems for Advanced Applications,
pages 76–85. Springer.
Xu, H., Martin, E., and Mahidadia, A. (2013). Using hetero-
geneous features for scientific citation classification.
In Proceedings of the 13th conference of the Pacific
Association for Computational Linguistics.
Xu, J., Zhang, Y., Wu, Y., Wang, J., Dong, X., and Xu, H.
(2015). Citation sentiment analysis in clinical trial pa-
pers. In AMIA Annual Symposium Proceedings, vol-
ume 2015, page 1334. American Medical Informatics
Association.
Yu, B. (2013). Automated citation sentiment analysis: what
can we learn from biomedical researchers. Proceed-
ings of the American Society for Information Science
and Technology, 50(1):1–9.
SentiCite - An Approach for Publication Sentiment Analysis
429