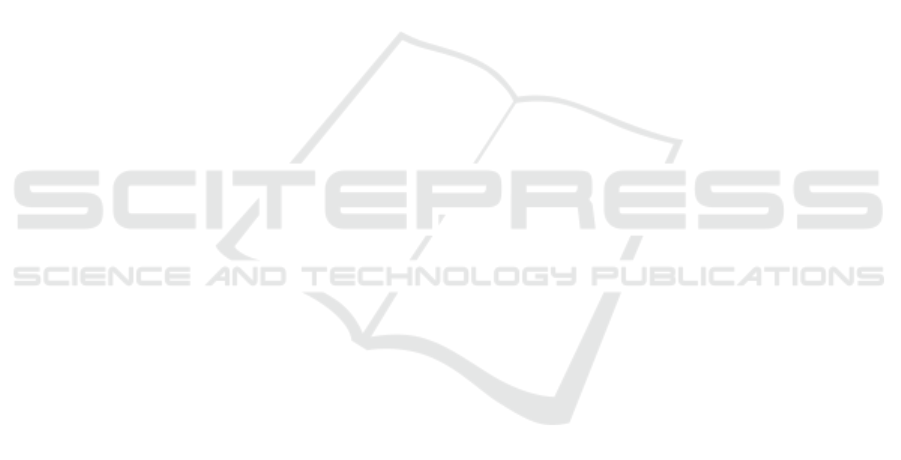
indicates that it is important to achieve such point as
faster as robots can. Although we have provided a
good alternative for the deterministic solution, in fu-
ture works, we want to explore alternatives to improve
the speed to reach such point without depending on
TAMs or losing autonomy.
Moreover, the phenomenon of diminishing returns
is present in many scenarios where the incorporation
of an additional worker to a job may improve the per-
formance, but each additional worker increases (grad-
ually) in smaller portions the performance. We have
seen this kind of shapes in many previous works of
MRTA because robots are sharing limited resources.
Therefore, there is a great opportunity to adjust this
same set of rules for those systems. It would be easily
adjusted for each environment and its conditions.
ACKNOWLEDGEMENTS
GMBO is grateful to Fapemig, CNPq and CAPES for
their financial support. Juan M Nogales also is grate-
ful with the OEA for the scholarship.
REFERENCES
Ahuja, R. K., Ergun,
¨
O., Orlin, J. B., and Punnen, A. P.
(2002). A survey of very large-scale neighborhood
search techniques. Discrete Applied Mathematics,
123(1):75–102.
Akbarimajd, A. and Simzan, G. (2014). Application of ar-
tificial capital market in task allocation in multi-robot
foraging. Int. Journal of Computational Intelligence
Systems, 7(3):401–417.
Anderson, C. and Bartholdi, J. J. (2000). Centralized versus
decentralized control in manufacturing: lessons from
social insects. Complexity and complex systems in in-
dustry, pages 92–105.
Antoun, A., Valentini, G., Hocquard, E., Wiandt, B., Tri-
anni, V., and Dorigo, M. (2016). Kilogrid: A modular
virtualization environment for the kilobot robot. In
Proc. of the IEEE/RSJ Int. Conf. on Intelligent Robots
and Systems, pages 3809–3814, Daejeon, South Ko-
rea. IEEE.
Bertsekas, D. P. (1999). Nonlinear programming. Athena
scientific Belmont.
Bobadilla, L., Martinez, F., Gobst, E., Gossman, K., and
LaValle, S. M. (2012). Controlling wild mobile robots
using virtual gates and discrete transitions. In Ameri-
can Control Conf., pages 743–749. IEEE.
Bonabeau, E., Dorigo, M., and Theraulaz, G. (1999).
Swarm intelligence: from natural to artificial systems.
Number 1. Oxford university press.
Bonabeau, E., Sobkowski, A., Theraulaz, G., Deneubourg,
J.-L., et al. (1997). Adaptive task allocation inspired
by a model of division of labor in social insects. In
Proc. Biocomputing and emergent computation, pages
36–45, Skovde, Sweden.
Brutschy, A., Garattoni, L., Brambilla, M., Francesca, G.,
Pini, G., Dorigo, M., and Birattari, M. (2015). The
TAM: abstracting complex tasks in swarm robotics re-
search. Swarm Intelligence, 9(1):1–22.
Buchanan, E., Pomfret, A., and Timmis, J. (2016). Dynamic
task partitioning for foraging robot swarms. Proc. of
the 10th Int. Conf. on Swarm Intelligence, pages 113–
124.
Cao, Y. U., Fukunaga, A. S., and Kahng, A. (1997). Coop-
erative mobile robotics: Antecedents and directions.
Autonomous Robots, 4(1):7–27.
Chaimowicz, L., Campos, M. F. M., and Kumar, V. (2002).
Dynamic role assignment for cooperative robots. In
Proc. of the IEEE Int. Conf. on Robotics and Automa-
tion, volume 1, pages 293–298 vol.1.
Dasgupta, P. (2011). Multi-Robot Task Allocation for Per-
forming Cooperative Foraging Tasks in an Initially
Unknown Environment, pages 5–20. Springer Berlin
Heidelberg, Berlin, Heidelberg.
De Marco, R. and Farina, W. (2001). Changes in food
source profitability affect the trophallactic and dance
behavior of forager honeybees (apis mellifera). Be-
havioral Ecology and Sociobiology, 50(5):441–449.
F
¨
are, R. (1980). Laws of diminishing returns. Number 176.
Springer Verlag.
Gerkey, B. P. and Matari, M. J. (2004). A formal anal-
ysis and taxonomy of task allocation in multi-robot
systems. The Int. Journal of Robotics Research,
23(9):939–954.
Jeanne, R. L. and Nordheim, E. V. (1996). Productivity in
a social wasp: per capita output increases with swarm
size. Behavioral Ecology, 7(1):43–48.
Khan, M. U., Li, S., Wang, Q., and Shao, Z. (2016). Cps ori-
ented control design for networked surveillance robots
with multiple physical constraints. IEEE Transactions
on Computer-Aided Design of Integrated Circuits and
Systems, 35(5):778–791.
Krause, A. and Guestrin, C. (2007). Near-optimal obser-
vation selection using submodular functions. In Proc.
of the 22nd Conf. on Artificial Intelligence, volume 7,
pages 1650–1654.
Kube, C. and Bonabeau, E. (2000). Cooperative transport
by ants and robots. Robotics and Autonomous Sys-
tems, 30(1):85 – 101.
Lerman, K. and Galstyan, A. (2002). Mathematical model
of foraging in a group of robots: Effect of interference.
Autonomous Robots, 13(2):127–141.
Liu, Y. and Nejat, G. (2013). Robotic urban search and res-
cue: A survey from the control perspective. Journal
of Intelligent & Robotic Systems, 72(2):147–165.
Marden, J. R., Arslan, G., and Shamma, J. S. (2009). Co-
operative control and potential games. IEEE Transac-
tions on Systems, Man, and Cybernetics, Part B (Cy-
bernetics), 39(6):1393–1407.
Mataric, M. J. (1994). Reward functions for accelerated
learning. In Proc. of the Eleventh Int. Conf. on Ma-
chine Learning, pages 181–189. Morgan Kaufmann.
Team Distribution between Foraging Tasks with Environmental Aids to Increase Autonomy
35