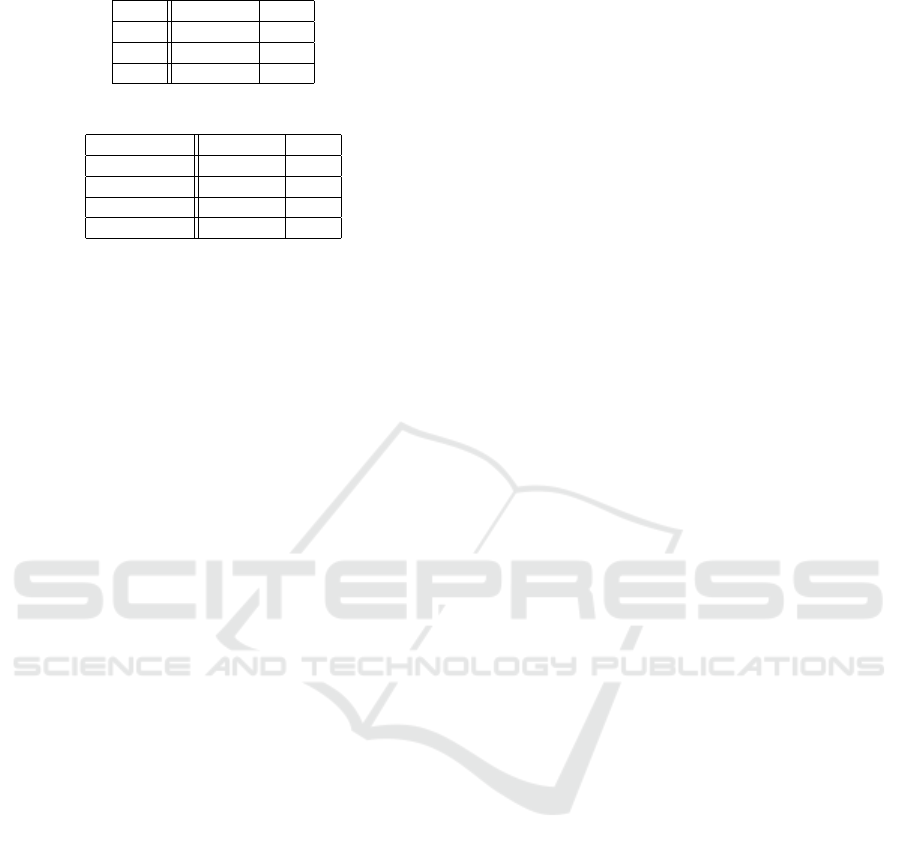
Table 1: Detection accuracy (KITTI dataset.)
# of frames DA
Curb 127 0.095
Other 56 0.071
Total 183 0.088
Table 2: Detection accuracy (HRB dataset.)
# of frames DA
Curb 100 0.046
Grass 161 0.118
Snow side wall 142 0.080
Total 403 0.087
This result shows that the error of 0.27m occurs when
the width of the driving lane is about 3m. However,
this result shows that a vehicle has some space to run
in the driving lane if the vehicle width is less than
2.5m. Therefore, the proposed method can also be
applied to ordinary vehicles.
Table 2 shows the detection accuracy DA in the
HRB dataset. The average DA of all scenes is 0.087
and DA of the snow side wall is 0.08. This result
shows that the proposed method is effective for road
boundary detection on snowy roads.
8 CONCLUSION
This paper proposed the method to detect the shoulder
line of a road including the boundary with the snow
side wall from an image of an in-vehicle monocular
camera. Vertical lines on an object whose height is
different from a road surface are projected onto slant-
ing lines when an input image is mapped to a road
surface by the inverse perspective mapping. The pro-
posed method detects a road boundary using this char-
acteristic. In the IPM edge image, the degree of road
boundary that responds strongly at the boundary with
the area where slant edges are dense is calculated
by using the parallelogram shaped mask. The road
boundary is tracked by the Snakes whose image force
is the degree of road boundary. Experimental results
using the KITTI dataset and our own dataset including
snow scenes show the effectiveness of the proposed
method. The future work is to improve the detection
accuracy of distant shoulder.
REFERENCES
A. Laddha, M. K. Kocamaz , L. E. N-serment, and M.
Hebert (2016). Map-supervised road detection. In
Proceedings of IV2016.
B. Hillel, R. Lerner, D. Levi, and G. Raz (2014). Recent
progress in road and lane detection: A survey. Ma-
chine Vision and Applications, 25(3):727–745.
C. Brust, S. Sickert, M. Simon, E. Rodner and J. Denzler
(2015). Convolutional patch networks with spatial
prior for road detection and urban scene understans-
ing. In Proceedings of VISAPP2015.
C. Guo, J. Meguro, Y. Kojima and T. Naito (2013). Cadas: a
multimodal advanced driver assistance system for nor-
mal urban streets based on road context understand-
ing. In Proceedings of IV2013, pages 228–235.
C. Kreucher and S. Lakshmanan (1999). Lana: A lane
extraction algorithm that uses frequency domain fea-
tures. IEEE Trans. on Robotics and Automation,
15(2):343–350.
D. Costea and S. Nedevschi (2017). Traffic scene segmen-
tation based on boosting over multimodal low, inter-
mediate and high order multi-range channel features.
In Proceedings of IV2017.
D. Hoiem, A. A. Efros and M. Hebert (2007). Recovering
surface layout from an image. International Journal
of Computer Vision, 75(1):151–172.
D. Levi, N. Garnett and E. Fetaya (2015). Stixelnet: A depp
convolutional network for obstacle detection and road
segmentation. In Proceedings of BMVC2015.
D. Pfeiffer and U. Franke (2010). Efficient representation
of traffic scenes by means of dynamic stixels. In Pro-
ceedings of IV2010, pages 217–224.
G. L. Oliveira, W. Burgard and T. Brox (2016). Efficient
deep models for monocular road segmentation. In
Proceedings of IROS2016, pages 586–595.
G. Thomas, N. Jerome and S. Laurent (2010). Frequency
filtering and connected components charaterization
for zebra-crossing and hatched markings detection.
In Proceedings of ISPRS Commision III Symposium,
pages 43–47.
J. C. McCall and M. M. Trivedi (2006). Video-based lane
estimation and tracking for driver assistance: Survey,
system, and evaluation. IEEE Trans. on Intelligent
Transportation Systems, 7(1):20–37.
J. K. Suhr and H. G. Jung (2013). Noise resilient road sur-
face and free space estimation using dense stereo. In
Proceedings of IV2013, pages 461–466.
J. M. Alvarez, T. Gevers and A. M. Lopez (2010). 3d
scene priors for road detection. In Proceedings of
CVPR2010.
J. M. Alvarez, T. Gevers, Y. LeCum and A. M. Lopez
(2012). Road scene segmentation from a single im-
age. In Proceedings of ECCV2012, pages 376–389.
J. M. Alvarez, Y. LeCum, T. Gevers and A. M. Lopez
(2012). Semantic road segmentation via multi-scale
ensembles of learned features. In Proceedings of
ECCV2012, pages 586–595.
J. Siegemund, D. Pfeiffer, U. Franke and W. Forstner
(2010). Curb reconstruction using conditional random
fields. In Proceedings of IV2010, pages 203–210.
J. Zhang and H. Nagel (1994). Texture-based segmentation
of road images. In Proceedings of IV1994.
J.Douret, R. Labayrade, J. Laneurit and R. Chapuis (2005).
A reliable and robust lane detection system based on
ICPRAM 2018 - 7th International Conference on Pattern Recognition Applications and Methods
386