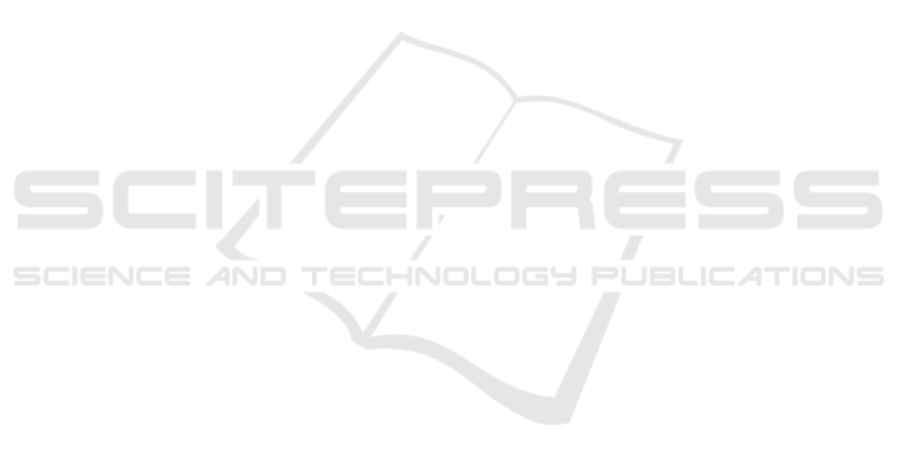
ontology as a last step. A reasoner assigns the clusters
to classes, which represent the operational state based
on its features. The approach was tested at a wind
power plant data set with six clusters. All clusters are
assigned correctly to the operational modes.
Therefore, the aim, to determine the operational
state without explicitly defining it for a use case, is
achieved. There are just generic definitions used,
which are suitable for similar applications. If the
application changes, it has to be adapted only once.
But since a classification is made of many continuous
signals, it can happen that really small changes lead
to another operational state, but this is quite normal
since it is an abstraction.
Further work can deal with the generic part of the
data preprocessing, since it has to be adapted manu-
ally regarding the use case. In particular the norma-
lization can cause some trouble, if there are uncom-
mon values, which deform the range of the values and
lead to wrong classification, which should be handled.
Furthermore, additional machine learning techniques
can be integrated and maybe combined to achieve a
better results.
ACKNOWLEDGEMENT
The work was supported by the German Federal
Ministry of Education and Research (BMBF) under
the projects ”Semantics4Automation” (funding code:
13FH020I3) and ”Provenance Analytics” (funding
code: 03PSIPT5B).
REFERENCES
Ali, I., Madi, N. A., and Melton, A. (2017). Using text
comprehension model for learning concepts, context,
and topic of web content. In 2017 IEEE 11th Inter-
national Conference on Semantic Computing (ICSC),
pages 101–104.
Alibeigi, M., Ahmadabadi, M. N., and Araabi, B. N. (2017).
A fast, robust, and incremental model for learning
high-level concepts from human motions by imitation.
IEEE Transactions on Robotics, 33(1):153–168.
Antoniou, G., , Antoniou, G., Antoniou, G., Harmelen,
F. V., and Harmelen, F. V. (2003). Web ontology lan-
guage: Owl. In Handbook on Ontologies in Informa-
tion Systems, pages 67–92. Springer.
Araki, T., Nakamura, T., and Nagai, T. (2013). Long-term
learning of concept and word by robots: Interactive le-
arning framework and preliminary results. In Interna-
tional Conference on Intelligent Robots and Systems,
pages 2280–2287.
Bunte, A., Li, P., and Niggemann, O. (2017). Learned ab-
straction: Knowledge based concept learning for cy-
ber physical systems. In 3rd Conference on Machine
Learning for Cyber Physical Systems and Industry 4.0
(ML4CPS).
Cimiano, P., Hotho, A., and Staab, S. (2005). Learning con-
cept hierarchies from text corpora using formal con-
cept analysis. J. Artif. Int. Res., 24(1):305–339.
Drumond, L. and Girardi, R. (2008). A survey of onto-
logy learning procedures. In WONTO, volume 427 of
CEUR Workshop Proceedings. CEUR-WS.org.
Jia, R., Heck, L., Hakkani-T
¨
ur, D., and Nikolov, G. (2017).
Learning concepts through conversations in spoken
dialogue systems. In 2017 IEEE International Con-
ference on Acoustics, Speech and Signal Processing
(ICASSP), pages 5725–5729.
Lake, B. M. (2014). Towards more human-like concept le-
arning in machines: Compositionality, causality, and
learning-to-learn. PhD thesis, Massachusetts Institute
of Technology.
Lehmann, J. and Voelker, J. (2014). An introduction to
ontology learning. In Lehmann, J. and Voelker, J.,
editors, Perspectives on Ontology Learning, pages ix–
xvi. AKA / IOS Press.
Mahmoodian, M., Moradi, H., Ahmadabadi, M. N., and
Araabi, B. N. (2013). Hierarchical concept learning
based on functional similarity of actions. In First In-
ternational Conference on Robotics and Mechatronics
(ICRoM), pages 1–6.
Ocampo-Guzman, I., Lopez-Arevalo, I., and Sosa-Sosa, V.
(2009). Data-driven approach for ontology learning.
In 2009 6th International Conference on Electrical
Engineering, Computing Science and Automatic Con-
trol (CCE), pages 1–6.
Suma, T. and Swamy, Y. S. K. (2016). Email classifi-
cation using adaptive ontologies learning. In 2016
IEEE International Conference on Recent Trends in
Electronics, Information Communication Technology
(RTEICT), pages 2102–2106.
Zhu, M., Gao, Z., Pan, J. Z., Zhao, Y., Xu, Y., and Quan, Z.
(2013). Ontology learning from incomplete semantic
web data by belnet. In 2013 IEEE 25th International
Conference on Tools with Artificial Intelligence, pages
761–768.
Mapping Data Sets to Concepts using Machine Learning and a Knowledge based Approach
437