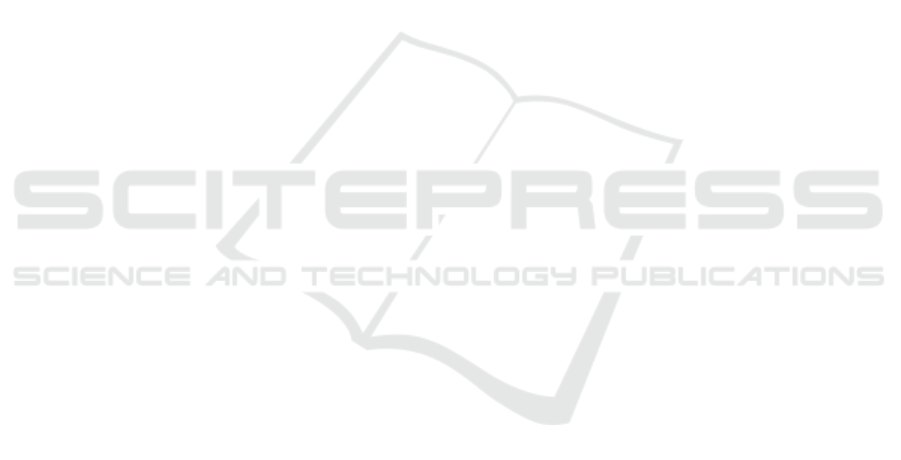
ACKNOWLEDGEMENTS
The authors would like to thank the German
research foundation (Deutsche Forschungsgemein-
schaft, DFG) for the financial support in the context
of the “Organic Computing Techniques for Runtime
Self-Adaptation of Multi-Modal Activity Recognition
Systems” project (SI 674/12-1).
REFERENCES
Abowd, G., Dey, A., Brown, P., Davies, N., Smith, M., and
Steggles, P. (1999). Towards a better understanding
of context and context-awareness. In Proc. of HUC,
pages 304–307. Springer.
Amer, M., Goldstein, M., and Abdennadher, S. (2013). En-
hancing one-class support vector machines for unsu-
pervised anomaly detection. In Proceedings of the
ACM SIGKDD Workshop on Outlier Detection and
Description, ODD ’13, pages 8–15, New York, NY,
USA. ACM.
Atlas, L. E., Cohn, D. A., and Ladner, R. E. (1990). Train-
ing connectionist networks with queries and selective
sampling. In Touretzky, D. S., editor, Advances in
Neural Information Processing Systems 2, pages 566–
573. Morgan-Kaufmann.
Bishop, C. M. (2006a). Pattern Recognition and Machine
Learning, chapter 10 Variational Inference, pages 461
– 486. Springer, New York, NY.
Bishop, C. M. (2006b). Pattern Recognition and Machine
Learning. Springer, New York, NY.
Chen, L., Hoey, J., Nugent, C. D., Cook, D. J., and Yu,
Z. (2012). Sensor-based activity recognition. IEEE
Transactions on Systems, Man, and Cybernetics, Part
C (Applications and Reviews), 42(6):790–808.
Clarkson, B., Mase, K., and Pentland, A. (2000). Recog-
nizing user context via wearable sensors. In Proc. of
ISWC, pages 69–75.
Fernndez-Francos, D., Martnez-Rego, D., Fontenla-
Romero, O., and Alonso-Betanzos, A. (2013). Au-
tomatic bearing fault diagnosis based on one-class ν-
svm. Computers & Industrial Engineering, 64(1):357
– 365.
Fisch, D., J
¨
anicke, M., Kalkowski, E., and Sick, B.
(2009). Learning by teaching versus learning by do-
ing: Knowledge exchange in organic agent systems.
In Intelligent Agents, 2009. IA’09. IEEE Symposium,
pages 31–38. IEEE.
Franke, T., Lukowicz, P., Kunze, K., and Bannach, D.
(2009). Can a mobile phone in a pocket reliably rec-
ognize ambient sounds? In Wearable Computers,
2009. ISWC’09. International Symposium on, pages
161–162. IEEE.
Gellersen, H., Schmidt, A., and Beigl, M. (2002). Multi-
sensor context-awareness in mobile devices and
smart artifacts. Mobile Networks and Applications,
7(5):341–351.
Gruhl, C. and Sick, B. (2016). Detecting novel pro-
cesses with CANDIES - an holistic novelty detec-
tion technique based on probabilistic models. CoRR,
abs/1605.05628.
Guerbai, Y., Chibani, Y., and Hadjadji, B. (2015).
The effective use of the one-class {SVM} classi-
fier for handwritten signature verification based on
writer-independent parameters. Pattern Recognition,
48(1):103 – 113.
Junker, H., Amft, O., Lukowicz, P., and Trster, G. (2008).
Gesture spotting with body-worn inertial sensors to
detect user activities. Pattern Recognition, 41(6):2010
– 2024.
Kephart, J. and Chess, D. (2003). The Vision of Autonomic
Computing. IEEE Computer, 36(1):41–50.
Kwapisz, J., Weiss, G., and Moore, S. (2011). Activity
recognition using cell phone accelerometers. ACM
SIGKDD Explorations Newsletter, 12(2):74–82.
Lau, S. and David, K. (2010). Movement recognition using
the accelerometer in smartphones. In Future Network
and Mobile Summit, 2010, pages 1–9. IEEE.
Lau, S., K
¨
onig, I., David, K., Parandian, B., Carius-D
¨
ussel,
C., and Schultz, M. (2010). Supporting patient moni-
toring using activity recognition with a smartphone. In
Wireless Communication Systems (ISWCS), 2010 7th
International Symposium on, pages 810–814. IEEE.
Li, K.-L., Huang, H.-K., Tian, S.-F., and Xu, W. (2003). Im-
proving one-class svm for anomaly detection. In Ma-
chine Learning and Cybernetics, 2003 International
Conference on, volume 5, pages 3077–3081. IEEE.
Markou, M. and Singh, S. (2003a). Novelty Detection: a
review – part 1: statistical approaches. Signal Pro-
cessing, 83:2481–2497.
Markou, M. and Singh, S. (2003b). Novelty Detection: a re-
view – part 2: neural network based approaches. Sig-
nal Processing, 83:2499–2521.
Sch
¨
olkopf, B., Williamson, R. C., Smola, A. J., Shawe-
Taylor, J., Platt, J. C., et al. (1999). Support vector
method for novelty detection. In NIPS, volume 12,
pages 582–588.
Sun, L., Zhang, D., Li, B., Guo, B., and Li, S. (2010). Activ-
ity recognition on an accelerometer embedded mobile
phone with varying positions and orientations. Ubiq-
uitous Intelligence and Computing, pages 548–562.
Tax, D. and Duin, R. (2002). Uniform Object Generation
for Optimizing One-class Classifiers. The Journal of
Machine Learning Research, 2:155–173.
Tomforde, S., Sick, B., and M
¨
uller-Schloer, C. (2017).
Organic Computing in the Spotlight. arXiv.org.
http://arxiv.org/abs/1701.08125.
Zhang, Y., Meratnia, N., and Havinga, P. (2009). Adap-
tive and online one-class support vector machine-
based outlier detection techniques for wireless sen-
sor networks. In 2009 International Conference on
Advanced Information Networking and Applications
Workshops, pages 990–995.
ICAART 2018 - 10th International Conference on Agents and Artificial Intelligence
142