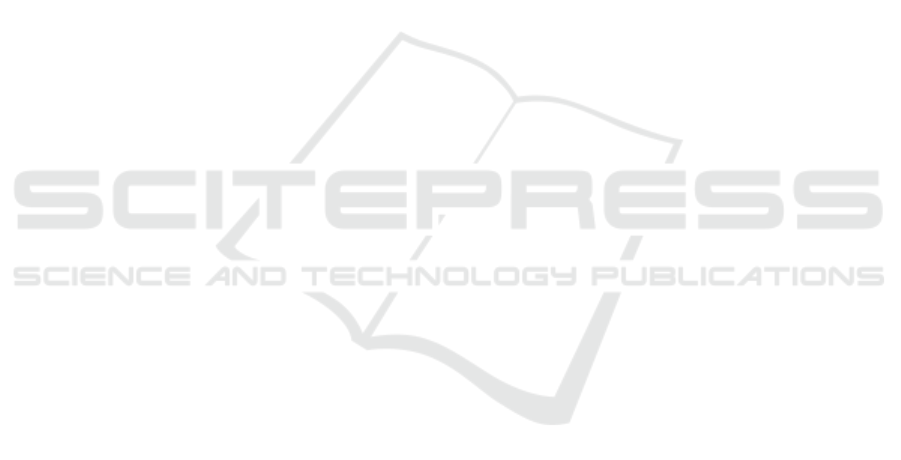
6 CONCLUSIONS
A hierarchical human activity recognition model that
recognizes human activities by the combinations of the
basic actions and involved objects has been proposed
in order to realize an easy-to-deploy activity recogni-
tion system. Unlike conventional activity recognition
models, the proposed model does not need retraining
for recognizing a new activity if the activity is repre-
sented by a combination of predefined basic actions
and basic objects. Two wearable sensors, namely Myo
armband sensor and ETG, have been utilized for the
action recognition and object recognition, respectively.
The experimental results have shown that the accuracy
of both basic modules are reasonably high, and the
proposed model could recognize 3 types of activities
with precision of 77% and recall rate of 82%. The
future works include expansion of target activities as
well as enhancing the basic modules.
REFERENCES
Aggarwal, J. (1999). Human Motion Analysis: A Review.
CVIU, 73(3):428–440.
Aggarwal, J. K. and Ryoo, M. S. (2011). Human activity
analysis: A review. ACM Computing Surveys, 43(3):1–
43.
Betancourt, A., Morerio, P., Regazzoni, C. S., and Rauter-
berg, M. (2015). The evolution of first person vision
methods: A survey. IEEE Trans. Circuits and Systems
for Video Technology, 25(5):744–760.
Cheng, H.-T., Sun, F.-T., Griss, M., Davis, P., Li, J., and You,
D. (2013). Nuactiv: Recognizing unseen new activities
using semantic attribute-based learning. In Proc. Inter-
national Conference on Mobile Systems, Applications,
and Services, pages 361–374. ACM.
Donahue, J., Hendricks, L. A., Guadarrama, S., Rohrbach,
M., Venugopalan, S., Saenko, K., and Darrell, T.
(2015). Long-term recurrent convolutional networks
for visual recognition and description. In CVPR.
Fathi, A., Li, Y., and Rehg, J. M. (2012). Learning to recog-
nize daily actions using gaze. In ECCV.
Jain, M., van Gemert, J. C., and Snoek, C. G. (2015). What
do 15,000 object categories tell us about classifying
and localizing actions? In CVPR, pages 46–55.
Lavee, G., Rivlin, E., and Rudzsky, M. (2009). Understand-
ing video events: a survey of methods for automatic
interpretation of semantic occurrences in video. IEEE
Trans. Systems, Man and Cybernetics Part C: Applica-
tions and Reviews, 39(5):489–504.
Li, Y., Ye, Z., and Rehg, J. M. (2015). Delving into egocen-
tric actions. In CVPR, pages 287–295.
Liu, J., Kuipers, B., and Savarese, S. (2011). Recognizing
human actions by attributes. In CVPR, pages 3337–
3344. IEEE.
Ma, M., Fan, H., and Kitani, K. M. (2016). Going deeper
into first-person activity recognition. In CVPR, pages
1894–1903.
Maekawa, T., Yanagisawa, Y., Kishino, Y., Ishiguro, K.,
Kamei, K., Sakurai, Y., and Okadome, T. (2010).
Object-based activity recognition with heterogeneous
sensors on wrist. In Proc. International Conference on
Pervasive Computing, pages 246–264. Springer.
Nguyen, T.-H.-C., Nebel, J.-C., Florez-Revuelta, F., et al.
(2016). Recognition of activities of daily living with
egocentric vision: A review. Sensors, 16(1):72.
Ohashi, H., A. Naser, M., Ahmed, S., Akiyama, T., Sato,
T., Nguyen, P., Nakamura, K., and Dengel, A. (2017).
Augmenting Wearable Sensor Data with Physical Con-
straint for DNN-Based Human-Action Recognition. In
Time Series Workshop @ ICML.
Palatucci, M., Pomerleau, D., Hinton, G. E., and Mitchell,
T. M. (2009). Zero-shot learning with semantic output
codes. In NIPS, pages 1410–1418.
Peng, X. and Schmid, C. (2016). Multi-region two-stream
r-cnn for action detection. In ECCV, pages 744–759.
Simonyan, K. and Zisserman, A. (2014). Two-stream convo-
lutional networks for action recognition in videos. In
NIPS, pages 568–576.
Socher, R., Ganjoo, M., Manning, C. D., and Ng, A. (2013).
Zero-shot learning through cross-modal transfer. In
NIPS, pages 935–943.
Spriggs, E. H., De La Torre, F., and Hebert, M. (2009).
Temporal segmentation and activity classification from
first-person sensing. In CVPR Workshops, pages 17–24.
IEEE.
Szegedy, C., Liu, W., Jia, Y., Sermanet, P., Reed, S.,
Anguelov, D., Erhan, D., Vanhoucke, V., and Rabi-
novich, A. (2015). Going deeper with convolutions. In
CVPR, pages 1–9.
Tran, D., Bourdev, L., Fergus, R., Torresani, L., and Paluri,
M. (2015). Learning spatiotemporal features with 3D
convolutional networks. In ICCV.
Turaga, P., Chellappa, R., Subrahmanian, V. S., and Udrea,
O. (2008). Machine recognition of human activities:
A survey. IEEE Trans. Circuits and Systems for Video
Technology, 18(11):1473–1488.
Wang, H., Kl, A., Schmid, C., and Liu, C.-l. (2011). Action
recognition by dense trajectories. In CVPR, pages
3169–3176.
Wang, H. and Schmid, C. (2013). Action recognition with
improved trajectories. In ICCV, pages 3551–3558.
Wang, L., Xiong, Y., Wang, Z., Qiao, Y., Lin, D., Tang, X.,
and Van Gool, L. (2016). Temporal segment networks:
towards good practices for deep action recognition. In
ECCV, pages 20–36.
Yao, B., Jiang, X., Khosla, A., Lin, A. L., Guibas, L., and Fei-
Fei, L. (2011). Human action recognition by learning
bases of action attributes and parts. In ICCV, pages
1331–1338.
Hierarchical Model for Zero-shot Activity Recognition using Wearable Sensors
485