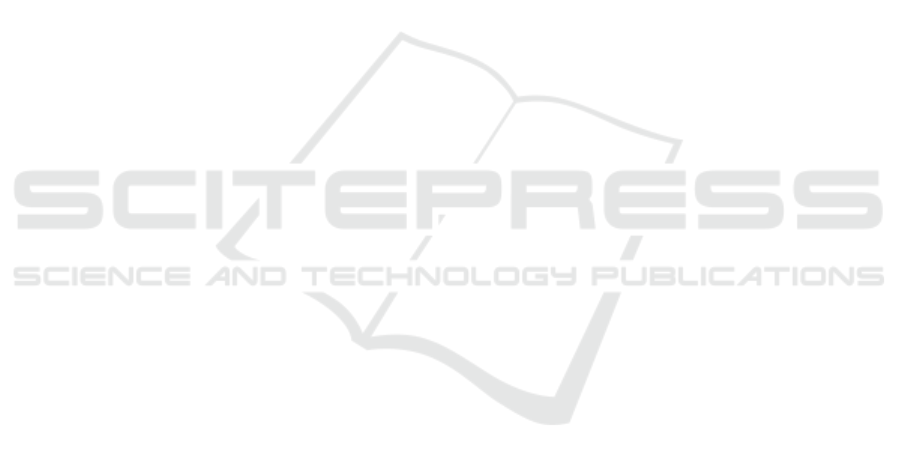
bret, H., Fielding, A., Fo
`
a, R., G
¨
okbuget, N., Gold-
stone, A., Goulden, N., Henze, G., Hoelzer, D., Janka-
Schaub, G., Macintyre, E., Pieters, R., Rambaldi, A.,
Ribera, J.-M., Schmiegelow, K., Spinelli, O., Stary,
J., von Stackelberg, A., Kneba, M., Schrappe, M., and
van Dongen, J. (2010). Standardized MRD quantifica-
tion in European ALL trials: proceedings of the Sec-
ond International Symposium on MRD assessment in
Kiel, Germany, 18-20 September 2008. Leukemia :
official journal of the Leukemia Society of America,
Leukemia Research Fund, U.K, 24(3):521–535.
Creutzig, U., Zimmermann, M., Bourquin, J.-p., Dworzak,
M. N., Fleischhack, G., Graf, N., Klingebiel, T., Kre-
mens, B., Lehrnbecher, T., Neuhoff, C. V., Sander,
A., Stackelberg, A. V., Star, J., and Reinhardt, D.
(2013a). Randomized trial comparing liposomal
daunorubicin with idarubicin as induction for pedi-
atric acute myeloid leukemia : results from study.
Blood, 122(1):37–44.
Creutzig, U., Zimmermann, M., Dworzak, M. N., Ritter,
J., Schellong, G., and Reinhardt, D. (2013b). Devel-
opment of a curative treatment within the AML-BFM
studies. Klinische Padiatrie, 225(SUPPL1):79–86.
Dworzak, M. (2013). Minimal residual disease in pedi-
atric acute lymphoblastic leukemia: Bfm experience.
Hematolog
´
ıa, 17.
Dworzak, M., Fr
¨
oschl, G., Printz, D., Mann, G., P
¨
otschger,
U., M
¨
uhlegger, N., Fritsch, G., and Gadner, H.
(2002). Prognostic significance and modalities of
flow cytometric minimal residual disease detection
in childhood acute lymphoblastic leukemia. Blood,
99(6):1952–1958.
Gaipa, G., Cazzaniga, G., Valsecchi, M., Panzer-Gr
¨
umayer,
R., Buldini, B., Silvestri, D., Karawajew, L., Maglia,
O., Ratei, R., Benetello, A., Sala, S., Schumich, A.,
Schrauder, A., Villa, T., Veltroni, M., Ludwig, W.-
D., Conter, V., Schrappe, M., Biondi, A., Dworzak,
M., and Basso, G. (2012). Time point-dependent
concordance of flow cytometry and real-time quan-
titative polymerase chain reaction for minimal resid-
ual disease detection in childhood acute lymphoblas-
tic leukemia. Haematologica, 97(10):1582–93.
Inaba, H., Greaves, M., and Mullighan, C. (2013). Acute
lymphoblastic leukaemia. Lancet (London, England),
381(9881):1943–55.
Juliusson, G., Antunovic, P., Derolf, A., Lehmann, S.,
M
¨
ollg
˚
ard, L., Stockelberg, D., Tidefelt, U., Wahlin,
A., and H
¨
oglund, M. (2009). Age and acute myeloid
leukemia: real world data on decision to treat and out-
comes from the Swedish Acute Leukemia Registry.
Blood, 113(18):4179–87.
Langs, G., Menze, B. H., Lashkari, D., and Golland,
P. (2011). Detecting stable distributed patterns of
brain activation using gini contrast. Neuroimage,
56(2):497–507.
Licandro, R., Rota, P., Reiter, M., and Kampel, M. (2016).
Flow Cytometry based automatic MRD assessment in
Acute Lymphoblastic Leukaemia: Longitudinal eval-
uation of time-specific cell population models. In
2016 14th International Workshop on Content-Based
Multimedia Indexing (CBMI), pages 1–6. IEEE.
L
¨
owenberg, B., Griffin, J. D., and Tallman, M. S. (2003).
Acute myeloid leukemia and acute promyelocytic
leukemia. Hematology / the Education Program of the
American Society of Hematology. American Society of
Hematology. Education Program, pages 82–101.
Naim, I., Datta, S., Rebhahn, J., Cavenaugh, J., Mosmann,
T., and Sharma, G. (2014). Swift - scalable clustering
for automated identification of rare cell populations in
large, high-dimensional flow cytometry datasets, part
1: Algorithm design. Cytometry Part A, 85(5):408–
421.
Pedregosa, F., Varoquaux, G., Gramfort, A., Michel, V.,
Thirion, B., Grisel, O., Blondel, M., Prettenhofer,
P., Weiss, R., Dubourg, V., Vanderplas, J., Passos,
A., Cournapeau, D., Brucher, M., Perrot, M., and
Duchesnay, E. (2011). Scikit-learn: Machine learning
in Python. Journal of Machine Learning Research,
12:2825–2830.
Powers, D. M. W. (2011). Evaluation: From precision, re-
call and f-measure to roc., informedness, markedness
& correlation. Journal of Machine Learning Tech-
nologies, 2(1):37–63.
Pui, C.-H., Robison, L., and Look, A. (2008). Acute lym-
phoblastic leukaemia. The Lancet, 371(9617):1030–
1043.
Puumala, S. E., Ross, J. A., Aplenc, R., and Spector, L. G.
(2013). Epidemiology of childhood acute myeloid
leukemia. Pediatric blood & cancer, 60(5):728–33.
Reiter, M., Rota, P., Kleber, F., Diem, M., Groeneveld-
Krentz, S., and Dworzak, M. (2016). Clustering of
cell populations in flow cytometry data using a com-
bination of Gaussian mixtures. Pattern Recognition,
60:1029–1040.
Rota, P., Reiter, M., Groeneveld-Krentz, S., and Kampel,
M. (2016). The role of machine learning in medical
data analysis. a case study: Flow cytometry. In Pro-
ceedings of the Internaction Conference on Computer
Vision Theory and Applications.
Rubnitz, J. E. and Inaba, H. (2012). Childhood acute
myeloid leukaemia. British Journal of Haematology,
159(3):259–276.
Zare, H., Shooshtari, P., Gupta, A., and Brinkman, R.
(2010). Data reduction for spectral clustering to ana-
lyze high throughput flow cytometry data. BMC bioin-
formatics, 11(1):403.
ICPRAM 2018 - 7th International Conference on Pattern Recognition Applications and Methods
408