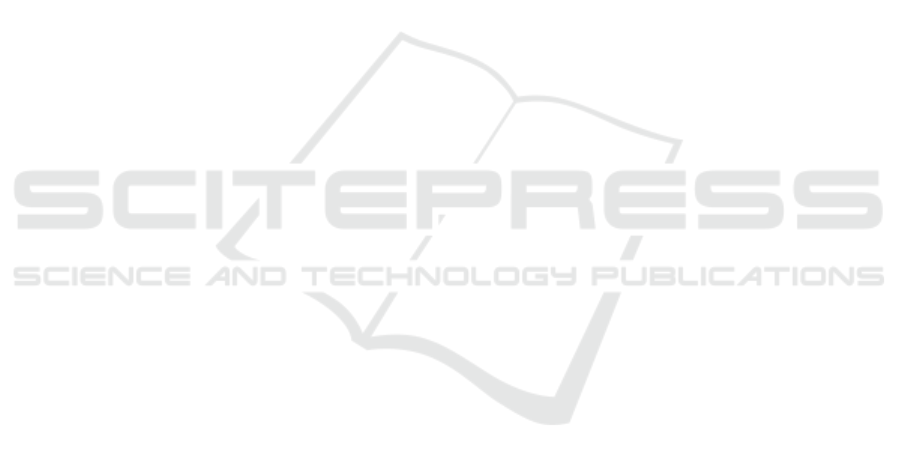
Pattern Recognition (CVPR), 2005 IEEE Conference
on, volume 1, pages 886–893.
Deng, J., Dong, W., Socher, R., Li, L.-J., Li, K., and Fei-Fei,
L. (2009). Imagenet: A large-scale hierarchical image
database. In Computer Vision and Pattern Recogni-
tion, 2009. CVPR 2009. IEEE Conference on, pages
248–255. IEEE.
Dileep, A. D. and Chandra Sekhar, C. (2014). GMM-
based intermediate matching kernel for classification
of varying length patterns of long duration speech us-
ing support vector machines. IEEE Transactions on
Neural Networks and Learning Systems, 25(8):1421–
1432.
Gao, B.-B., Wei, X.-S., Wu, J., and Lin, W. (2015). Deep
spatial pyramid: The devil is once again in the details.
CoRR, abs/1504.05277.
Girshick, R., Donahue, J., Darrell, T., and Malik, J. (2014).
Rich feature hierarchies for accurate object detec-
tion and semantic segmentation. In Proceedings of
the IEEE conference on computer vision and pattern
recognition, pages 580–587.
Gong, Y., Wang, L., Guo, R., and Lazebnik, S. (2014).
Multi-scale orderless pooling of deep convolutional
activation features. In European conference on com-
puter vision, pages 392–407. Springer.
Gupta, S., Dileep, A. D., and Thenkanidiyoor, V. (2016a).
Segment-level pyramid match kernels for the classi-
fication of varying length patterns of speech using
svms. In Signal Processing Conference (EUSIPCO),
2016 24th European, pages 2030–2034. IEEE.
Gupta, S., Thenkanidiyoor, V., and Dinesh, D. A. (2016b).
Segment-level probabilistic sequence kernel based
support vector machines for classification of varying
length patterns of speech. In International Confer-
ence on Neural Information Processing, pages 321–
328. Springer.
He, K., Zhang, X., Ren, S., and Sun, J. (2015). Spatial pyra-
mid pooling in deep convolutional networks for visual
recognition. IEEE transactions on pattern analysis
and machine intelligence, 37(9):1904–1916.
J
´
egou, H., Douze, M., Schmid, C., and P
´
erez, P. (2010). Ag-
gregating local descriptors into a compact image rep-
resentation. In Computer Vision and Pattern Recogni-
tion (CVPR), 2010 IEEE Conference on, pages 3304–
3311. IEEE.
Juneja, M., Vedaldi, A., Jawahar, C., and Zisserman, A.
(2013). Blocks that shout: Distinctive parts for scene
classification. In Proceedings of the IEEE Conference
on Computer Vision and Pattern Recognition, pages
923–930.
Kang, K. and Wang, X. (2014). Fully convolutional neu-
ral networks for crowd segmentation. arXiv preprint
arXiv:1411.4464.
Krizhevsky, A., Sutskever, I., and Hinton, G. E. (2012). Im-
agenet classification with deep convolutional neural
networks. In Advances in neural information process-
ing systems, pages 1097–1105.
Lazebnik, S., Schmid, C., and Ponce, J. (2006). Beyond
bags of features: Spatial pyramid matching for recog-
nizing natural scene categories. In Computer Vision
and Pattern Recognition (CVPR), 2006 IEEE Confer-
ence on, volume 2, pages 2169–2178.
Lowe, D. G. (2004). Distinctive image features from scale-
invariant keypoints. International journal of computer
vision, 60(2):91–110.
Mandar, Dixit, S., Chen, D., Gao, N., Rasiwasia, Nuno, and
Vasconcelos (2015). Scene classification with seman-
tic fisher vectors. In Proceedings of the IEEE con-
ference on computer vision and pattern recognition,
pages 2974–2983.
Oliva, A. and Torralba, A. (2001). Modeling the shape
of the scene: A holistic representation of the spatial
envelope. International journal of computer vision,
42(3):145–175.
Quattoni, A. and Torralba, A. (2009). Recognizing indoor
scenes. In Computer Vision and Pattern Recognition,
2009. CVPR 2009. IEEE Conference on, pages 413–
420. IEEE.
Simonyan, K. and Zisserman, A. (2014). Very deep con-
volutional networks for large-scale image recognition.
arXiv preprint arXiv:1409.1556.
Szegedy, C., Liu, W., Jia, Y., Sermanet, P., Reed, S.,
Anguelov, D., Erhan, D., Vanhoucke, V., and Rabi-
novich, A. (2015). Going deeper with convolutions.
In Proceedings of the IEEE conference on computer
vision and pattern recognition, pages 1–9.
Thenkanidiyoor, V., Dileep, A. D., and Chandra Sekhar, C.
(2017). Dynamic kernels based approaches to anal-
ysis of varying length patterns in speech and image
processing tasks. In Amita Pal, S. K. P., editor, Pat-
tern Recognition and Big Data. World Scientific.
Vogel, J. and Schiele, B. (2004). Natural scene retrieval
based on a semantic modeling step. In International
Conference on Image and Video Retrieval, pages 207–
215. Springer.
Xiao, J., Hays, J., Ehinger, K. A., Oliva, A., and Torralba,
A. (2010). Sun database: Large-scale scene recogni-
tion from abbey to zoo. In Computer vision and pat-
tern recognition (CVPR), 2010 IEEE conference on,
pages 3485–3492. IEEE.
Yoo, D., Park, S., Lee, J.-Y., and Kweon, I. S. (2014). Fisher
kernel for deep neural activations. arXiv preprint
arXiv:1412.1628.
Yoo, D., Park, S., Lee, J.-Y., and So Kweon, I. (2015).
Multi-scale pyramid pooling for deep convolutional
representation. In Proceedings of the IEEE Confer-
ence on Computer Vision and Pattern Recognition
Workshops, pages 71–80.
Zhao, F., Huang, Y., Wang, L., and Tan, T. (2015). Deep
semantic ranking based hashing for multi-label im-
age retrieval. In Proceedings of the IEEE Conference
on Computer Vision and Pattern Recognition, pages
1556–1564.
Zhou, B., Lapedriza, A., Khosla, A., Oliva, A., and Tor-
ralba, A. (2017). Places: A 10 million image database
for scene recognition. IEEE Transactions on Pattern
Analysis and Machine Intelligence.
Zhou, B., Lapedriza, A., Xiao, J., Torralba, A., and Oliva,
A. (2014). Learning deep features for scene recog-
nition using places database. In Advances in neural
information processing systems, pages 487–495.
ICPRAM 2018 - 7th International Conference on Pattern Recognition Applications and Methods
148