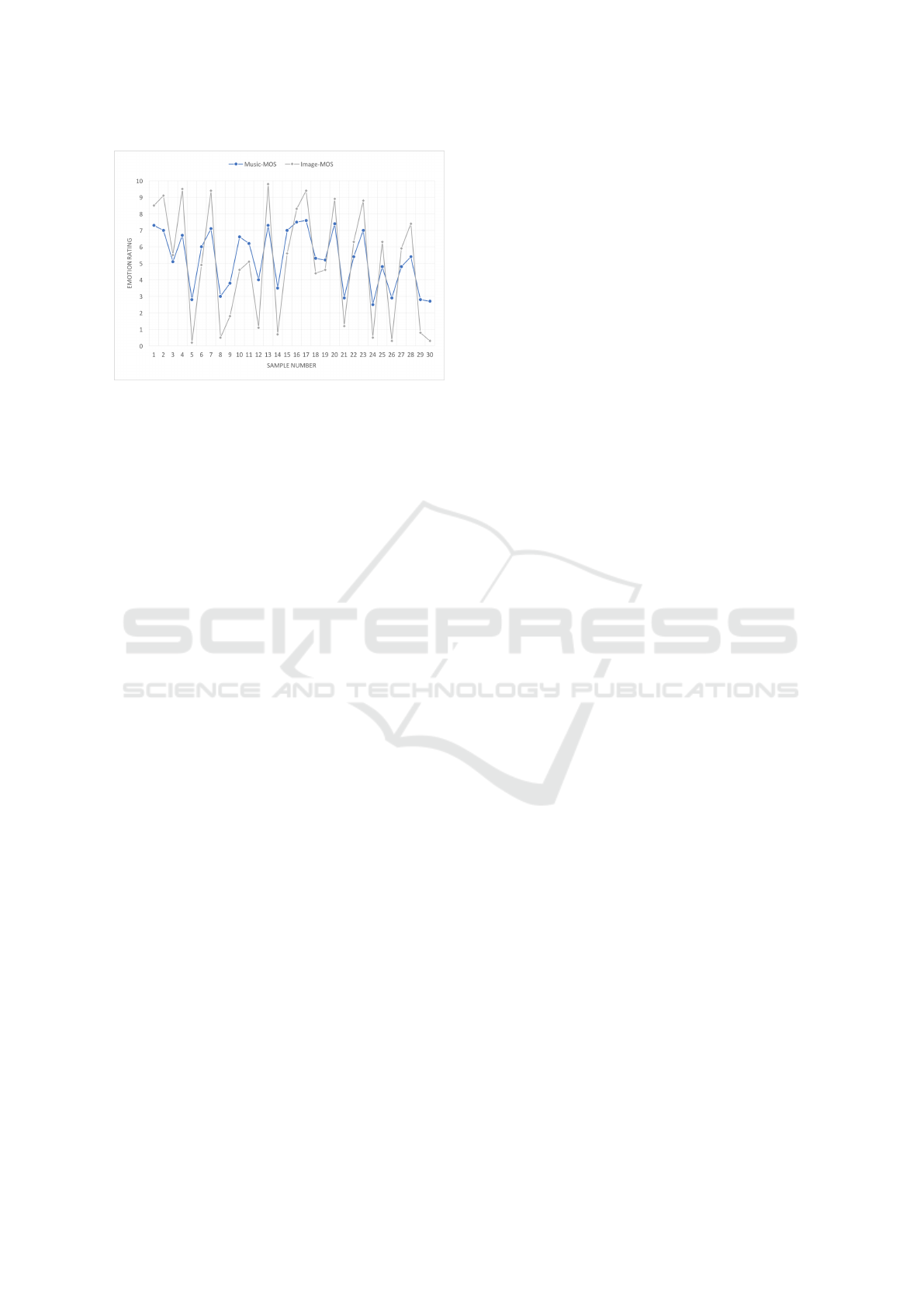
Figure 4: MOS Graph.
the self-sufficiency of the number of training samples
in the Music Generation Model.
Music is not just art and creativity but is also based
on a strong mathematical background. So, often com-
puter creativity is doubted in its ability to produce
fresh and creative works. New notions of computer
creativity can evolve by amalgamation of different
techniques, use of high performing systems and bio
inspiration.
Also, computer scientists and music composers
must work in synergy together. Making music com-
posers able enough to use the program by having a
basic understanding of the program and learning var-
ious commands would enable them to give construc-
tive feedback and radically change the process of mu-
sic composition, and consequently the way market
for music operates. Market opportunities would in-
clude incorporating such features in instant multime-
dia messaging applications such as Snapchat, Insta-
gram and any other application which deals with im-
ages.
ACKNOWLEDGEMENTS
We whole heartedly thank our mentor Dr. Rajni Jin-
dal, Head of Department, Computer Science Depart-
ment at Delhi Technological University for guiding us
through this project.
REFERENCES
Boulanger-Lewandowski, N., Bengio, Y., and Vincent, P.
(2012). Modeling Temporal Dependencies in High-
Dimensional Sequences: Application to Polyphonic
Music Generation and Transcription. ArXiv e-prints.
Chung, J., Gulcehre, C., Cho, K., and Bengio, Y. (2014).
Empirical Evaluation of Gated Recurrent Neural Net-
works on Sequence Modeling. ArXiv e-prints.
Goodfellow, I. J., Erhan, D., Carrier, P. L., Courville,
A., Mirza, M., Hamner, B., Cukierski, W., Tang, Y.,
Thaler, D., Lee, D.-H., Zhou, Y., Ramaiah, C., Feng,
F., Li, R., Wang, X., Athanasakis, D., Shawe-Taylor,
J., Milakov, M., Park, J., Ionescu, R., Popescu, M.,
Grozea, C., Bergstra, J., Xie, J., Romaszko, L., Xu,
B., Chuang, Z., and Bengio, Y. (2013). Challenges in
Representation Learning: A report on three machine
learning contests. ArXiv e-prints.
Graves, A. (2013). Generating Sequences With Recurrent
Neural Networks. ArXiv e-prints.
Gregory, G. (2014). Generating music through image anal-
ysis.
Hirve, R., Jagdale, S., Banthia, R., Kalal, H., and Pathak, K.
(2016). Emoplayer: An emotion based music player.
Imperial Journal of Interdisciplinary Research, 2(5).
Ip, H. H. S., Law, K. C. K., and Kwong, B. (2005). Cy-
ber composer: Hand gesture-driven intelligent music
composition and generation. In 11th International
Multimedia Modelling Conference, pages 46–52.
Jaques, N., Gu, S., Bahdanau, D., Hern
´
andez-Lobato, J. M.,
Turner, R. E., and Eck, D. (2016). Sequence Tu-
tor: Conservative Fine-Tuning of Sequence Genera-
tion Models with KL-control. ArXiv e-prints.
Rubin, S. and Agrawala, M. (2014). Generating emotion-
ally relevant musical scores for audio stories. In Pro-
ceedings of the 27th Annual ACM Symposium on User
Interface Software and Technology, UIST ’14, pages
439–448, New York, NY, USA. ACM.
Sergio, G. C., Mallipeddi, R., Kang, J.-S., and Lee, M.
(2015). Generating music from an image. In Proceed-
ings of the 3rd International Conference on Human-
Agent Interaction, HAI ’15, pages 213–216, New
York, NY, USA. ACM.
Zaware, N., Rajgure, T., Bhadang, A., and Sapkal, D.
(2014). Emotion based music player. International
Journal of Innovative Research and Development,
0(0).
ICAART 2018 - 10th International Conference on Agents and Artificial Intelligence
506