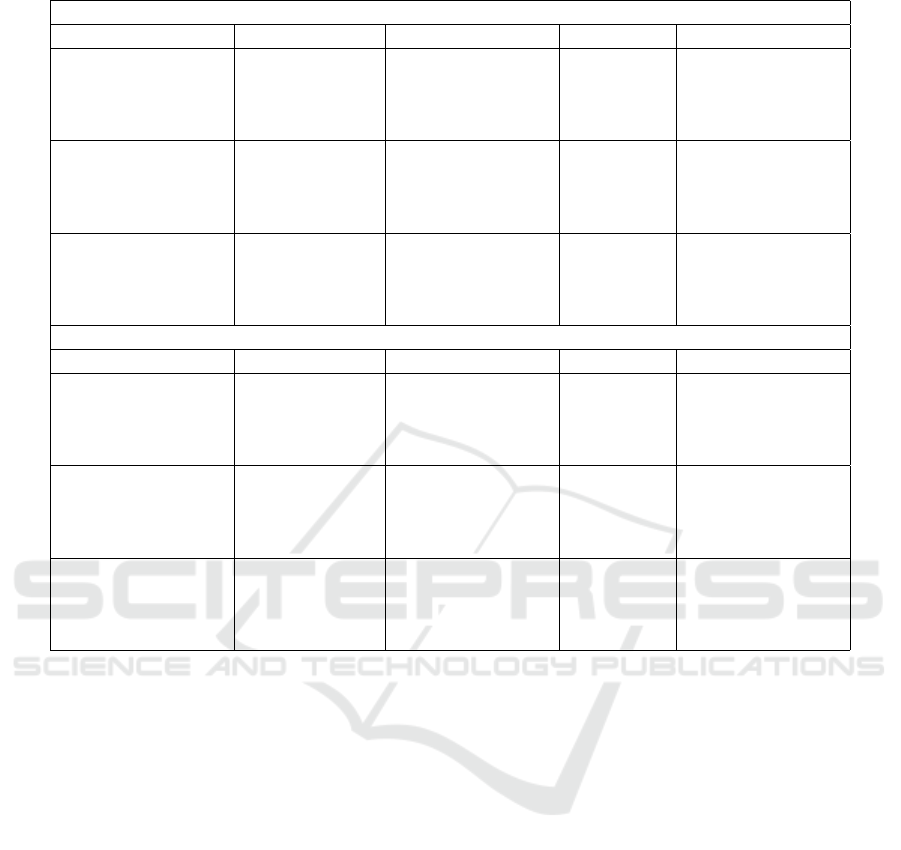
Table 4: Classification results.
SVM
Sets Recall score, % Precision score, % F
1
score, % Accuracy score, %
tf-idf 57.69 63.83 60.61 90.27
tf-idf + stl 63.46 61.11 62.26 90.02
tf-idf + mrph 63.46 56.89 60.02 89.02
tf-idf + stl + mrph 61.53 65.31 63.36 90.77
emb 84.61 47.31 60.68 85.78
emb + stl 84.61 48.35 61.53 86.28
emb + mrph 80.76 46.66 59.15 85.53
emb + stl + mrph 78.84 43.15 55.78 83.79
bigram 65.87 53.80 57.38 86.30
bigram + stl 61.62 51.40 53.30 84.66
bigram + mrph 54.12 61.34 50.42 84.44
bigram + stl + mrph 52.23 59.06 47.80 83.76
Random forest
Sets Recall score, % Precision score, % F
1
score, % Accuracy score, %
tf-idf 51.92 72.97 60.67 91.27
tf-idf + stl 51.92 77.14 62.06 91.77
tf-idf + mrph 51.92 79.41 62.79 92.01
tf-idf + stl + mrph 51.92 77.14 62.06 91.77
emb 55.76 53.70 54.71 88.02
emb + stl 57.69 53.57 55.55 88.02
emb + mrph 61.53 56.14 58.71 88.77
emb + stl + mrph 57.69 54.54 56.07 88.27
bigram 64.42 45.50 53.30 85.36
bigram + stl 63.88 45.32 52.99 85.30
bigram + mrph 69.54 44.07 53.92 84.58
bigram + stl + mrph 68.35 43.72 53.31 84.46
REFERENCES
Almeida, H., Briand, A., and Meurs, M.-J. (2017). Detect-
ing early risk of depression from social media user-
generated content.
De Choudhury, M., Gamon, M., Counts, S., and Horvitz,
E. (2013). Predicting depression via social media. In
ICWSM, page 2.
Farıas-Anzald
´
ua, A. A., Montes-y G
´
omez, M., L
´
opez-
Monroy, A. P., and Gonz
´
alez-Gurrola, L. C. (2017).
Uach-inaoe participation at erisk2017.
Ke
ˇ
selj, V., Peng, F., Cercone, N., and Thomas, C. (2003).
N-gram-based author profiles for authorship attribu-
tion. In Proceedings of the conference pacific asso-
ciation for computational linguistics, PACLING, vol-
ume 3, pages 255–264.
Losada, D. E. and Crestani, F. (2016). A test collection
for research on depression and language use. In In-
ternational Conference of the Cross-Language Eval-
uation Forum for European Languages, pages 28–39.
Springer.
Losada, D. E., Crestani, F., and Parapar, J. (2017). Clef
2017 erisk overview: Early risk prediction on the in-
ternet: Experimental foundations.
Maaten, L. v. d. and Hinton, G. (2008). Visualizing data
using t-sne. Journal of Machine Learning Research,
9(Nov):2579–2605.
Malam, I. A., Arziki, M., Bellazrak, M. N., Benamara,
F., El Kaidi, A., Es-Saghir, B., He, Z., Housni, M.,
Moriceau, V., Mothe, J., et al. (2017). Irit at e-risk.
Moussavi, S., Chatterji, S., Verdes, E., Tandon, A., Pa-
tel, V., and Ustun, B. (2007). Depression, chronic
diseases, and decrements in health: results from the
world health surveys. The Lancet, 370(9590):851–
858.
Padr
´
o, L. and Stanilovsky, E. (2012). Freeling 3.0: Towards
wider multilinguality. In LREC2012.
Pak, A. and Paroubek, P. (2010). Twitter as a corpus for
sentiment analysis and opinion mining. In LREc, vol-
ume 10.
Pedregosa, F., Varoquaux, G., Gramfort, A., Michel, V.,
Thirion, B., Grisel, O., Blondel, M., Prettenhofer, P.,
Weiss, R., Dubourg, V., et al. (2011). Scikit-learn:
Machine learning in python. Journal of Machine
Learning Research, 12(Oct):2825–2830.
Pennington, J., Socher, R., and Manning, C. D. (2014).
Glove: Global vectors for word representation. In
ICPRAM 2018 - 7th International Conference on Pattern Recognition Applications and Methods
430