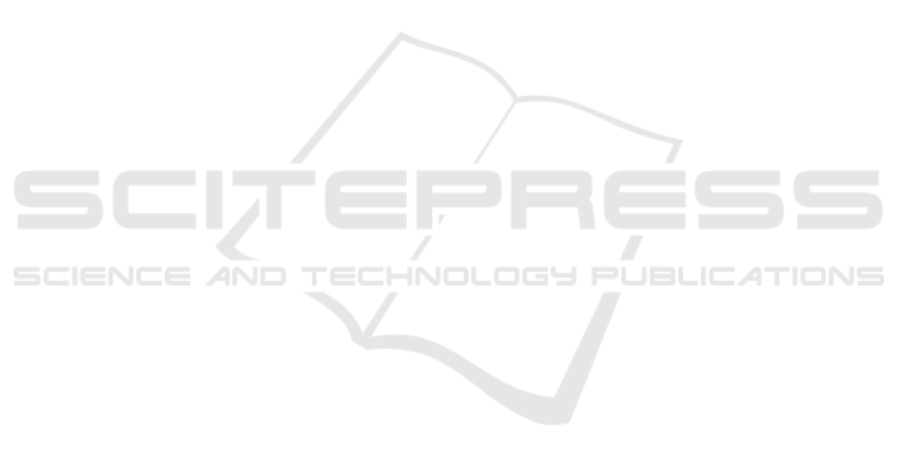
pricing of charging services for electric vehicles. Us-
ing the factored MDP demand-response pricing, we
aimed at the core objectives of electromobility: dis-
tribution of cost between the grid and EV owners,
signaling of power scarcity or abundance and incen-
tivization of behavior change of the EV drivers.
Experimentally, we have compared the demand-
response pricing strategy with the baseline of cur-
rently most commonly used time-of-use flat rate pric-
ing across a wide range of environmental parame-
ters, that is, the price elasticity of demand and volume
of demand for charging services. While the revenue
generated by the proposed demand-response pricing
method was higher than the flat rate pricing methods
only for specific values of the environmental param-
eters, our method performed better than any consid-
ered flat rate pricing in the achieved utilization of the
charging station and delivered energy across all con-
sidered scenarios. The improvement of our method
in the utilization of the charging station and delivered
energy over the flat rate pricing of comparable rev-
enue was up to 300%, depending on the price elastic-
ity and the demand.
As we mentioned in the paper, the most obvious
future work is to incorporate dependence of the con-
secutive time windows in the factored MDP model
and improve the demand model. Further, the model is
extendable to a game theoretic setting. Such approach
will, however, need substantial work to provide scal-
ability for practical use of the approach.
ACKNOWLEDGMENTS
This research was funded by the European Union
Horizon 2020 research and innovation programme
under the grant agreement N
◦
713864 and by the Grant
Agency of the Czech Technical University in Prague,
grant No. SGS16/235/OHK3/3T/13.
REFERENCES
Albadi, M. and El-Saadany, E. (2008). A summary of de-
mand response in electricity markets. Electric Power
Systems Research, 78(11):1989 – 1996.
Bellman, R. (1957). A markovian decision process. Journal
of Mathematics and Mechanics, 6:679–684.
Cao, Y., Tang, S., Li, C., Zhang, P., Tan, Y., Zhang, Z.,
and Li, J. (2012). An optimized EV charging model
considering TOU price and SOC curve. IEEE Trans.
Smart Grid, 3(1):388–393.
Chevaleyre, Y., Dunne, P. E., Endriss, U., Lang, J.,
Lema
ˆ
ıtre, M., Maudet, N., Padget, J. A., Phelps, S.,
Rodr
´
ıguez-Aguilar, J. A., and Sousa, P. (2006). Issues
in multiagent resource allocation. Informatica (Slove-
nia), 30(1):3–31.
Chiang, W.-C., Chen, J. C., and Xu, X. (2007). An overview
of research on revenue management: current issues
and future research. International Journal of Revenue
Management, 1(1):97–128.
Dyer, C., Epstein, M., and Culver, D. (2013). Station for
rapidly charging an electric vehicle battery. US Patent
8,350,526.
Li, R., Wu, Q., and Oren, S. S. (2014). Distribution lo-
cational marginal pricing for optimal electric vehicle
charging management. IEEE Transactions on Power
Systems, 29(1):203–211.
Lin Lawell, C.-Y. C. and Prince, L. (2013). Gasoline price
volatility and the elasticity of demand for gasoline.
Energy Economics, 38(C):111–117.
McGill, J. I. and van Ryzin, G. J. (1999). Revenue manage-
ment: Research overview and prospects. Transporta-
tion Science, 33(2):233–256.
McKinney, W. (2011). Pandas: a foundational python li-
brary for data analysis and statistics.
Puterman., M. L. (1994). Markov Decision Processes.
Rezaei, P., Frolik, J., and Hines, P. D. H. (2014). Packetized
plug-in electric vehicle charge management. IEEE
Transactions on Smart Grid, 5(2):642–650.
Subramanian, J., Jr., S. S., and Lautenbacher, C. J. (1999).
Airline yield management with overbooking, can-
cellations, and no-shows. Transportation Science,
33(2):147–167.
van der Walt, S., Colbert, S. C., and Varoquaux, G. (2011).
The numpy array: A structure for efficient numerical
computation. Computing in Science & Engineering,
13(2):22–30.
Versi, T. and Allington, M. (2016). Overview of the Electric
Vehicle market and the potential of charge points for
demand response. Technical report, ICF Consulting
Services.
ICAART 2018 - 10th International Conference on Agents and Artificial Intelligence
514