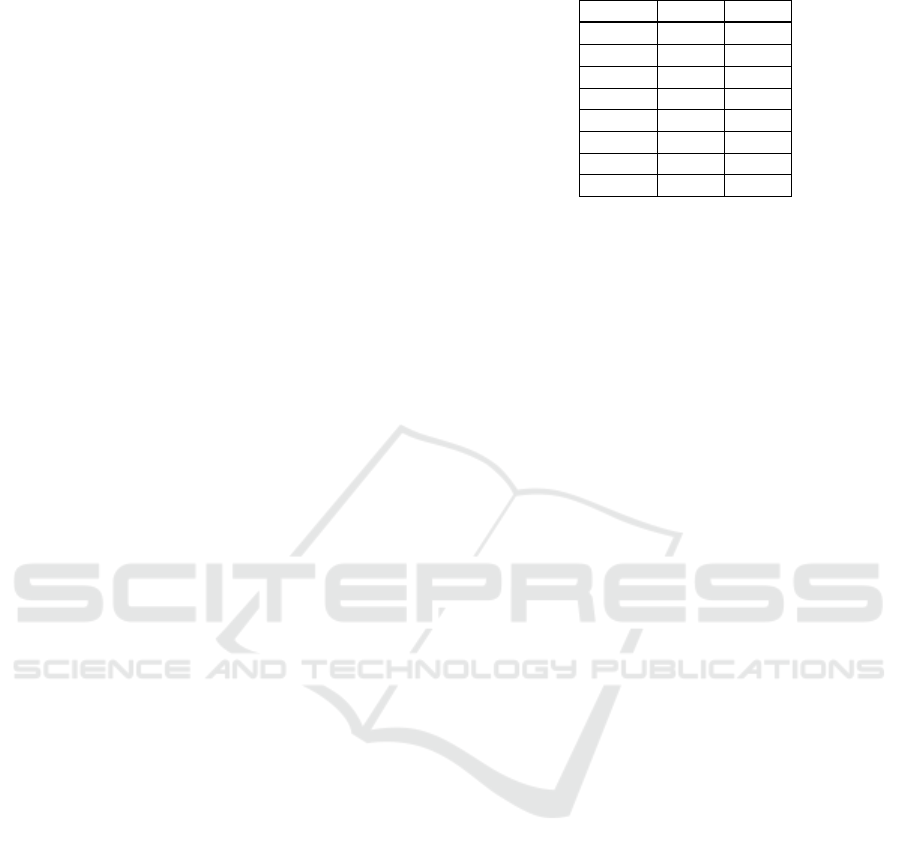
considered provider suggests the location changes of
operated centers.
The suggested model together with a suitable IP-
solver represent such a tool, which can enable the
negotiation under knowledge of consequences both
rules and provider’s behavior. As the considered
rules are quantified by the values of F, H, w and D,
the provider can find, what is the optimal profit
under the values and thus, he can conclude whether
the changes pay off.
As concerns the system administrator, the tool,
which models the provider’s behavior, enables to
investigate the provider’s profit under given values
F and H. Starting with some default values, e.g. the
transportation performance and the worst distance
between a user and the nearest center obtained for
the original center deployment, the administrator can
repeat the solving algorithm with step by step
decreased values and he can suggest such values,
which improve service accessibility for users and
also let the considered provider increase the profit.
Another issue of the tool for the administrator is
represented by a possibility to test effectiveness of
the auxiliary and formal rules w and D from the
point of users’ benefit.
5 COMPUTATIONAL STUDY
To study presented approach to reengineering of the
emergency service system, we performed series of
numerical experiments, in which the optimization
software FICO Xpress 8.0 (64-bit, release 2016) was
used and the experiments were run on a PC equipped
with the Intel® Core™ i7 5500U processor with the
parameters: 2.4 GHz and 16 GB RAM.
Used benchmarks were derived from real
emergency health care system, which was originally
implemented in eight regions of Slovak Republic.
For each self-governing region, i.e. Bratislava (BA),
Banská Bystrica (BB), Košice (KE), Nitra (NR),
Prešov (PO), Trenčín (TN), Trnava (TT) and Žilina
(ZA), all cities and villages with corresponding
number b
j
of inhabitants were taken into account.
The coefficients b
j
were rounded to hundreds. The
set of communities represents both the set J of users’
locations and the set I of possible center locations as
well. The cardinalities of these sets are reported in
Table 1, where the associated column is denoted by
|I|. The total number of located centers is given in
the column denoted as TNC. The network distance
from a user to the nearest located center was taken
as the user´s disutility.
Table 1: Size of used benchmarks.
An individual experiment was organized so that
the current deployment of service centers for each
self-governing region was studied first. The obtained
results are summarized in Table 2. The total
transportation performance was computed as a sum
of weighted distances between system users and the
nearest located service centers. The weights were set
to the number of users sharing the same location.
The values of the total transportation performance
are reported in column denoted by “Total TP”. For
each self-governing region, ten different instances
were generated randomly. These instances differ in
the list of located service centers operated by the
considered provider. The average percentage ratio of
the provider’s centers to all centers is reported in the
column denoted by “Prov. [%]”. The right part of
Table 2 denoted by “Max TP decrease” contains the
results of analysis aimed at computing the maximal
possible decrease of the total transportation
performance, which can be achieved by relocating
some of the provider’s service centers. To determine
these values, the model (1)-(15) was simplified. The
objective function value (1) was replaced by
minimization of the left part of the constraint (8),
whereas constraint (8) was completely excluded
from the model. The constraints containing variables
z
ij
{0, 1} for i
I
R
I
F
and j
J were not taken into
account, because they were not needed. Other
constraints stayed unchanged. The value of
parameter w was set to the cardinality of the
provider’s service center list. It means that all
centers operated by the considered provider could
change their location. The value of D was set to 15
according to the rule applied in the emergency
health care system of the Slovak Republic (Kvet and
Janáček, 2016). The value of H was set to the
maximal value of distance between a user and the
nearest located service center in the current design.
By solving the adjusted model, we obtained the
minimal value of transportation performance, which
can be obtained by reengineering. The average
computational time in seconds necessary for
problem solving is denoted by “Time [s]”.The last
ICORES 2018 - 7th International Conference on Operations Research and Enterprise Systems
228