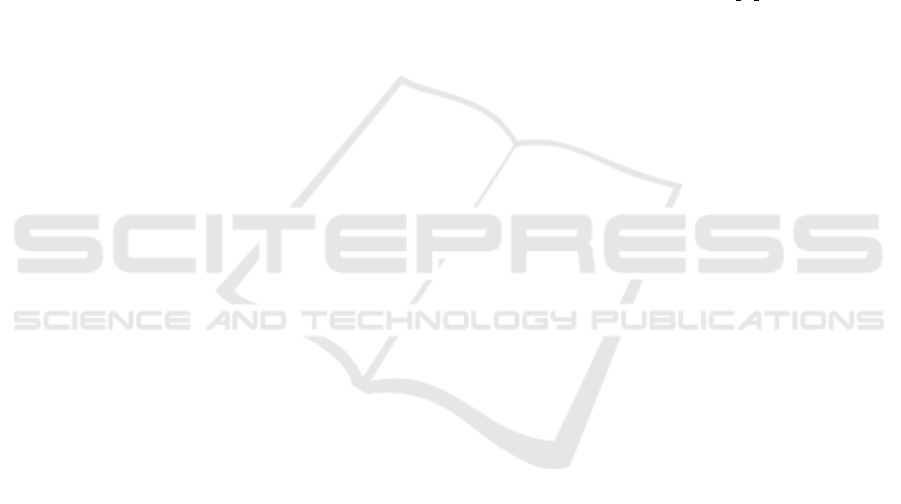
8 CONCLUSION AND
PERSPECTIVES
This manuscript introduces Crashzam, an innovative
way to detect car accidents with sound recorded by
smartphones or any other microphone-equipped smart
device installed in cars. The goal is to enhance dri-
vers’ safety with a low cost solution, capable to pro-
pose domain specific services like medical assistance
calling, first notification of loss (FNOL) or advice and
coordination during a minor event.
Sounds are analyzed by two models and the fi-
nal detection result reflects a combination of both of
them. The first model analyses the signal in time
and frequency, computing well-known features in the
sound recognition literature. The second one is based
on the analysis of spectrogram images and the disco-
very of patterns among a constellation of amplitude
peaks.
As no dataset of crash sounds recorded inside the
car cabin existed, we built one. Crash samples come
from both controlled experiments and real, genuine
conditions.
Detection results show a pretty accurate classifi-
cation between crash sounds and other sounds likely
to be reproduced in car. Also, combining models ana-
lyzing specific aspects of sound signals (time series,
frequency, spectrogram) helps the system to be more
accurate and reliable when the environment is noisy.
A wide range of perspectives are possible. We
proposed Crashzam in the context of driving safety
and connected car, but the same concept could be app-
lied to other domains such as the connected home and
the connected health. For instance, Minut, a Scan-
dinavian startup, is specialized on sound-based home
surveillance (Minut, 2017). Their devices are able to
detect alarm or glass breaking. Remaining in a the te-
lematics context, the detection of repetitive car horn
sounds gives an insight on the drivers’ driving style.
As regards the system design, the tendency is to
embed models into devices. For instance, one of
the most advertised novelty in the 2017 Google I/O
was the possibility to embed TensorFlow models in-
side smartphones (Android and iOS) (Google I/O ’17,
2017). In the safe driving context, having an auto-
nomous system that gets rid of server calling would
mean a full time detection availability.
Deep learning has gained more and more estima-
tion on image and audio classification tasks. Spectro-
gram image processing may be tested with Convolu-
tional Neural Networks to discover patterns. Nevert-
heless, in some working domains like insurance, it is
essential to know how to explain the results.
ACKNOWLEDGEMENTS
The authors would like to thank AXA Winterthur for
inviting the AXA Data Innovation Lab to participate
to the Spring 2016 crash test campaign and AXA Sin-
gapore for helping with the data crawling and clea-
ning.
REFERENCES
Aloul, F. A., Zualkernan, I. A., Abu-Salma, R., Al-Ali, H.,
and Al-Merri, M. (2015). ibump: Smartphone appli-
cation to detect car accidents. Computers & Electrical
Engineering, 43:66–75.
Car Crash Time (2017). Youtube channel. https://www.
youtube.com/channel/UCwuZi C yFtHsfCicthqygw.
Accessed: 2017-05-03.
Carletti, V., Foggia, P., Percannella, G., Saggese, A., Stris-
ciuglio, N., and Vento, M. (2013). Audio surveillance
using a bag of aural words classifier. 2013 10th IEEE
International Conference on Advanced Video and Sig-
nal Based Surveillance, AVSS 2013, (December):81–
86.
Clavel, C. and Ehrette, T. (2008). Fear-type emotion re-
cognition and abnormal events detection for an audio-
based surveillance system. WIT Transactions on In-
formation and Communication Technologies, 39:471–
479.
Crocco, M., Cristani, M., Trucco, A., and Murino, V.
(2016). Audio Surveillance: a Systematic Review.
ACM Computing Surveys, 48(4):1–44.
Dixon, S. (2006). Onset Detection Revisited. In Proc. of the
Int. Conf. on Digital Audio Effects (DAFx-06), pages
133–137, Montreal, Quebec, Canada.
Evanco, W. M. (1996). The impact of rapid incident de-
tection on freeway accident fatalities. Technical re-
port.
Foggia, P., Petkov, N., Saggese, A., Strisciuglio, N., and
Vento, M. (2016a). Audio surveillance of roads: A
system for detecting anomalous sounds. IEEE Tran-
sactions on Intelligent Transportation Systems.
Foggia, P., Petkov, N., Saggese, A., Strisciuglio, N., and
Vento, M. (2016b). Audio surveillance of roads:
A system for detecting anomalous sounds. IEEE
Transactions on Intelligent Transportation Systems,
17(1):279–288.
FreeSound (2017). On-line free sound samples.
www.freesound.org. Accessed: 2017-05-03.
Ganchev, T., Fakotakis, N., and Kokkinakis, G. (2005).
Comparative evaluation of various mfcc implementa-
tions on the speaker verification task. In in Proc. of
the SPECOM-2005, pages 191–194.
Giannakopoulos, T., Pikrakis, A., and Theodoridis, S.
(2007). A multi-class audio classification method with
respect to violent content in movies using bayesian
networks. In IEEE 9th Workshop on Multimedia Sig-
nal Processing, MMSP 2007, Chania, Crete, Greece,
October 1-3, 2007, pages 90–93.
VEHITS 2018 - 4th International Conference on Vehicle Technology and Intelligent Transport Systems
34