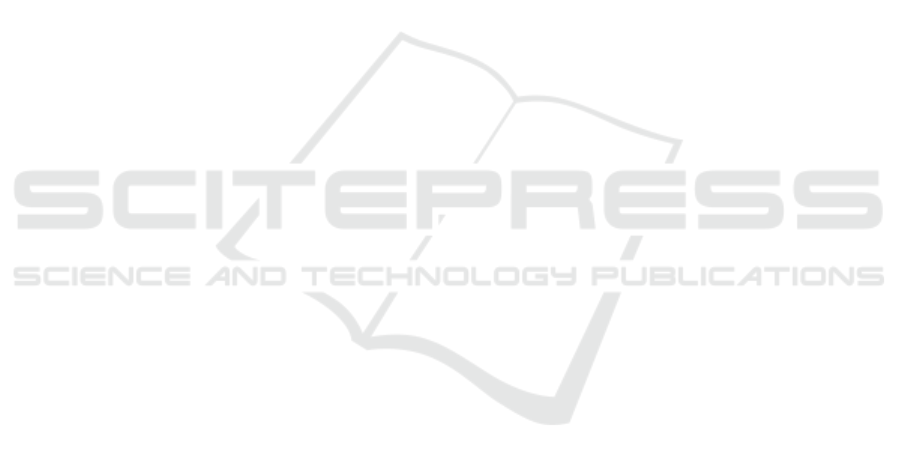
ACKNOWLEDGMENTS
Scientific research, publication and presentation are
supported by the ERANet-LAC Project ”Enabling re-
silient urban transportation systems in smart cities”
(RETRACT, ELAC2015/T10-0761).
REFERENCES
Ahmed, S. H., Bouk, S. H., Yaqub, M. A., Kim, D., Song,
H., and Lloret, J. (2016). Codie: Controlled data
and interest evaluation in vehicular named data net-
works. IEEE Transactions on Vehicular Technology,
65(6):3954–3963.
Anthopoulos, L. G. and Reddick, C. G. (2016). Understan-
ding electronic government research and smart city: A
framework and empirical evidence. Information Po-
lity, 21(1):99–117.
Bacon, J., Bejan, A., Beresford, A., Evans, D., Gibbens, R.,
and Moody, K. (2011). Using real-time road traffic
data to evaluate congestion. Dependable and Historic
Computing, pages 93–117.
Barcellos, P., Bouvi
´
e, C., Escouto, F. L., and Scharcanski, J.
(2015). A novel video based system for detecting and
counting vehicles at user-defined virtual loops. Expert
Systems with Applications, 42(4):1845–1856.
Bradski, G. (2000). The OpenCV Library. Dr. Dobb’s Jour-
nal of Software Tools.
Carli, R., Dotoli, M., Epicoco, N., Angelico, B., and Vinci-
ullo, A. (2015). Automated evaluation of urban traf-
fic congestion using bus as a probe. In Automation
Science and Engineering (CASE), 2015 IEEE Inter-
national Conference on, pages 967–972. IEEE.
Iwasaki, Y. and Itoyama, H. (2007). Real-time vehicle de-
tection using information of shadows underneath vehi-
cles. In Advances in Computer, Information, and Sys-
tems Sciences, and Engineering, pages 94–98. Sprin-
ger.
KaewTraKulPong, P. and Bowden, R. (2002). An impro-
ved adaptive background mixture model for real-time
tracking with shadow detection. Video-based surveil-
lance systems, 1:135–144.
Lim, K. H., Ang, L., Seng, K. P., and Chin, S. W. (2009).
Lane-vehicle detection and tracking. In Proceedings
of the international multiconference of engineers and
computer scientists 2009 Vol II IMECS 2009, Hong
Kong.
Mahizhnan, A. (1999). Smart cities: the singapore case.
Cities, 16(1):13–18.
Mandellos, N. A., Keramitsoglou, I., and Kiranoudis, C. T.
(2011). A background subtraction algorithm for de-
tecting and tracking vehicles. Expert Systems with Ap-
plications, 38(3):1619–1631.
Rad, R. and Jamzad, M. (2005). Real time classification
and tracking of multiple vehicles in highways. Pattern
Recognition Letters, 26(10):1597–1607.
Tian, B., Yao, Q., Gu, Y., Wang, K., and Li, Y. (2011).
Video processing techniques for traffic flow monito-
ring: A survey. In Intelligent Transportation Systems
(ITSC), 2011 14th International IEEE Conference on,
pages 1103–1108. IEEE.
Wan, J., Liu, J., Shao, Z., Vasilakos, A. V., Imran, M., and
Zhou, K. (2016). Mobile crowd sensing for traffic pre-
diction in internet of vehicles. Sensors, 16(1):88.
Wang, S., Zhang, X., Cao, J., He, L., Stenneth, L., Yu, P. S.,
Li, Z., and Huang, Z. (2017). Computing urban traf-
fic congestions by incorporating sparse gps probe data
and social media data. ACM Transactions on Informa-
tion Systems (TOIS), 35(4):40.
Wu, W., QiSen, Z., and Mingjun, W. (2001). A method
of vehicle classification using models and neural net-
works. In Vehicular Technology Conference, 2001.
VTC 2001 Spring. IEEE VTS 53rd, volume 4, pages
3022–3026. IEEE.
Zhu, Z., Yang, B., Xu, G., and Shi, D. (1996). A real-time
vision system for automatic traffic monitoring based
on 2d spatio-temporal images. In Applications of
Computer Vision, 1996. WACV’96., Proceedings 3rd
IEEE Workshop on, pages 162–167. IEEE.
Zivkovic, Z. (2004). Improved adaptive gaussian mixture
model for background subtraction. In Pattern Recog-
nition, 2004. ICPR 2004. Proceedings of the 17th In-
ternational Conference on, volume 2, pages 28–31.
IEEE.
Traffic Monitoring System Development in Jelgava City, Latvia
665