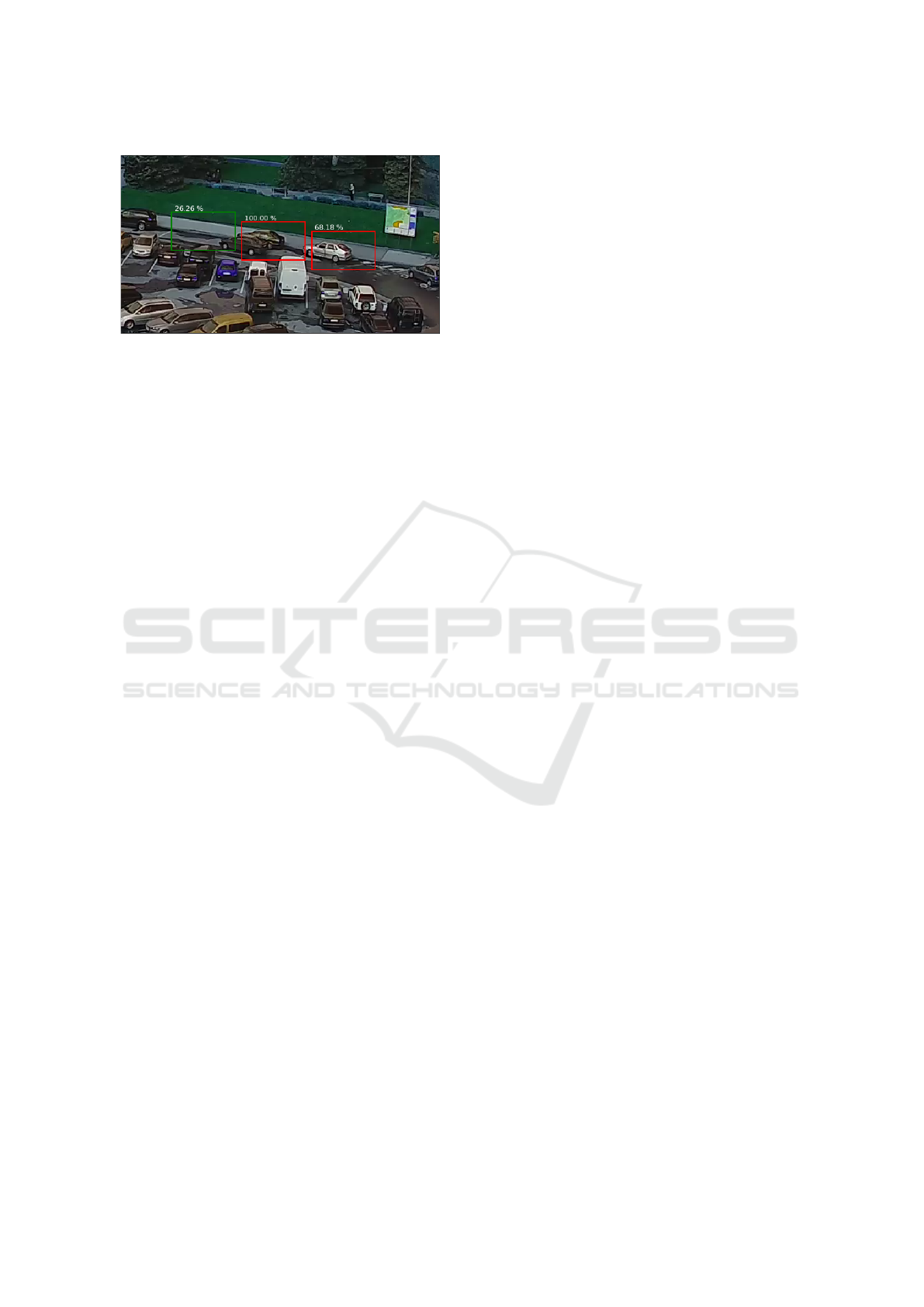
Figure 5: Parking lot status report.
be used for parking status detection with precision of
90% and more.
Results can be considered as good taking into ac-
count camera position, parking lot configuration and
drivers’ parking habits. Figure 5 shows an example
of parking status report. Frame colors indicate either
parking lot is free (green) or occupied (red). Percent-
ages show probability of lot occupancy P(car) calcu-
lated by model.
4 CONCLUSIONS
Results show that classification precision is not signif-
icantly dependent on number of image samples used
for model training (about 300 samples is enough for
desired precision).
Disadvantage of video based parking monitoring
approach is that if car is not parked directly at consid-
ered lot, than system will not detect car correctly. In
case parking lots are differently oriented to camera,
than several models have to be trained for each park-
ing lot orientation. In future it is planned to extend
this solution for whole parking monitoring.
Significant advantage of video based parking
monitoring is that existing infrastructure can be used:
already installed surveillance, security or other cam-
eras can be used for image acquisition.
For better results pixel spatial location aware mod-
els should be used (e.g. convolutional neural net-
works, histogram of oriented gradients, etc).
ACKNOWLEDGMENTS
Scientific research, publication and presentation are
supported by the ERANet-LAC Project Enabling re-
silient urban transportation systems in smart cities
(RETRACT, ELAC2015/T10-0761).
REFERENCES
Al-Kharusi, H. and Al-Bahadly, I. (2014). Intelligent
parking management system based on image process-
ing. World Journal of Engineering and Technology,
2(02):55.
Albino, V., Berardi, U., and Dangelico, R. M. (2015). Smart
cities: Definitions, dimensions, performance, and ini-
tiatives. Journal of Urban Technology, 22(1):3–21.
Arnott, R. and Inci, E. (2006). An integrated model of
downtown parking and traffic congestion. Journal of
Urban Economics, 60(3):418–442.
Bradski, G. (2000). The OpenCV Library. Dr. Dobb’s Jour-
nal of Software Tools.
Idris, M., Leng, Y., Tamil, E., Noor, N., and Razak, Z.
(2009). park system: a review of smart parking sys-
tem and its technology. Information Technology Jour-
nal, 8(2):101–113.
Kuran, M. S¸., Viana, A. C., Iannone, L., Kofman, D., Mer-
moud, G., and Vasseur, J. P. (2015). A smart parking
lot management system for scheduling the recharg-
ing of electric vehicles. IEEE Transactions on Smart
Grid, 6(6):2942–2953.
Lan, K.-C. and Shih, W.-Y. (2014). An intelligent driver lo-
cation system for smart parking. Expert Systems with
Applications, 41(5):2443–2456.
Pham, T. N., Tsai, M.-F., Nguyen, D. B., Dow, C.-R., and
Deng, D.-J. (2015). A cloud-based smart-parking sys-
tem based on internet-of-things technologies. IEEE
Access, 3:1581–1591.
Polycarpou, E., Lambrinos, L., and Protopapadakis, E.
(2013). Smart parking solutions for urban areas. In
World of Wireless, Mobile and Multimedia Networks
(WoWMoM), 2013 IEEE 14th International Sympo-
sium and Workshops on a, pages 1–6. IEEE.
Sevillano, X., M
`
armol, E., and Fernandez-Arguedas, V.
(2014). Towards smart traffic management systems:
Vacant on-street parking spot detection based on video
analytics. In Information Fusion (FUSION), 2014
17th International Conference on, pages 1–8. IEEE.
Shaheen, S. (2005). Smart parking management field test:
A bay area rapid transit (bart) district parking demon-
stration. Institute of Transportation Studies.
Shoup, D. C. (2006). Cruising for parking. Transport Pol-
icy, 13(6):479–486.
Zacepins, A., Komasilovs, V., Kviesis, A., Gatins, A., Sku-
dra, M., and Pierhurovics, A. (2017). Implementation
of smart parking system in jelgava city in latvia. In
Application of Information and Communication Tech-
nologies (AICT), 2016 IEEE 10th International Con-
ference on, pages (in–print). IEEE.
Implementation of Smart Parking Solution by Image Analysis
669