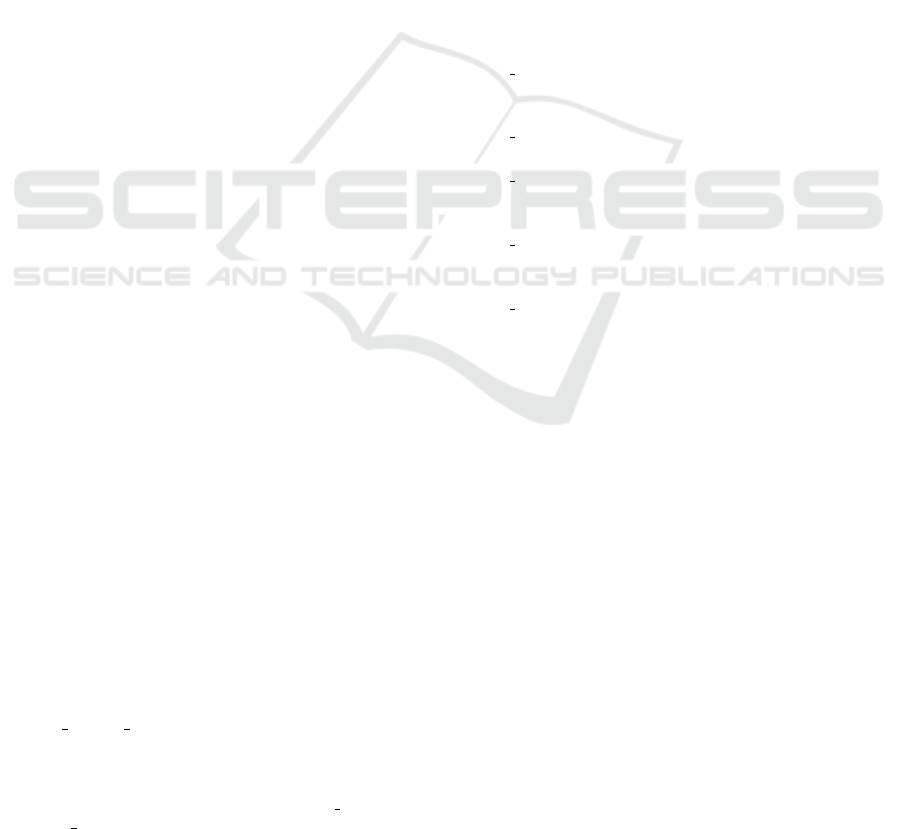
ters. Indeed, during the vote, the authorities will me-
asure a low bandwidth.
As far as bridge routers are concerned, a corrupted
machine in middle position could count the number
of network frames it is relaying when constructing a
circuit. It could then determine its own position and
hence compare the IP address of the machine right be-
fore its own position with those listed by the founda-
tion. It would enable to determine whether the input
router is a bridge or not. In order to prevent such an
attack, it is envisaged to always insert a guard router
between the bridge and the middle router. Thus, hid-
den nodes would be drowned within network users.
6 CONCLUSION
Our study tends to prove that the security of the Tor
network is not optimal. Indeed, since overall security
is based on the individual good practices of the router
owners, the maximum level that will be reached will
not exceed that of the weakest link. The latter can also
be set up voluntarily to identify particular users.
It is also important to note that local security (of no-
des and servers, protocol strength...) is not sufficient
as soon as we deal with a worldwide infrastructure.
Having a more global and higher point of view is also
important. In this respect, our study has showed the
Tor infrastructure too much relies actually on a redu-
ced number of nodes.
Our future work will mostly focus on two aspects.
First, we intend to optimize our multi threaded con-
sensus file processing algorithm. The aim is to be
able to process any new version of such file and ex-
tract top significant node subsets within less than one
minute. Second to go on working on the bridge mana-
gement and its evolution and to refine our extraction
algorithm.
REFERENCES
Alstott, J., Bullmore, E., and Plenz, D. (2014). Powerlaw:
A Python Package for Analysis of Heavy-Tailed Dis-
tributions. PLoS ONE, 9:e85777.
Clauset, A., Shalizi, C. R., and Newman, M. E. J. (2009).
Power-law distributions in empirical data. SIAM Rev.,
51(4):661–703.
Defense Science Board (2015). Study on 21st century
military operations in a complex electromagnetic en-
vironment. Office of the Under Secretary of Defense
for Acquisition, Technology, and Logistics. http://
www.acq.osd.mil/dsb/eports/2010s/DSB SS13–
EW Study.pdf.
Delong, M., Fatou, O., Filiol, E., Coddet, C., and Suhard, C.
(2018). Technical and osint analysis of the tor project.
In ICCWS’2018.
Filiol, E., Delong, M., and J., N. (2017). Results of the
tor routing protocol statistical and combinatorial ana-
lyses. http://cvo-lab.blogspot.fr/2017/09/preliminary-
results-on-tor-routing.html. Accessed: 2017-09-12.
Goldschlag, D. M., Reed, M. G., and Syverson, P. F. (1996).
Hiding Routing Information. In Anderson, R., edi-
tor, Proceedings of Information Hiding: First Inter-
national Workshop, pages 137–150. Springer-Verlag,
LNCS 1174.
Johnson, A., Wacek, C., Jansen, R., Sherr, M., and Syver-
son, P. (2013). Users get routed: Traffic correlation
on tor by realistic adversaries. In Proceedings of the
2013 ACM SIGSAC Conference on Computer &
Communications Security, CCS ’13, pages 337–348,
New York, NY, USA. ACM.
Syverson, P. F., Goldschlag, D. M., and Reed, M. G. (1997).
Anonymous connections and onion routing. In Pro-
ceedings of the 1997 IEEE Symposium on Security
and Privacy, SP ’97, pages 44–, Washington, DC,
USA. IEEE Computer Society.
TOR Foundation (2014a). Tor documentation. https://
www.torproject.org/docs/tor-manual.html.en. Acces-
sed: 2017-09-12.
TOR
Foundation (2014b). Tor project. https://gitweb.
torproject.org. Accessed: 2017-09-12.
TOR Foundation (2014c). The tor project. https://
www.torproject.org/docs/tor.git. Accessed: 2017-09-
12.
TOR Foundation (2014d). Tor specifications. https://
gitweb.torproject.org/torspec.git. Accessed: 2017-09-
12.
TOR Foundation (2015). Did the fbi pay a university
to attack tor users? https://blog.torproject.org/
did-fbi-pay-university-attack-tor-users. Accessed:
2017-09-12.
APPENDIX
Statistical Model for the TOR Routing
Protocol
The results obtained seem to indicate that the distri-
bution of TOR routes follows a power law distribu-
tion (general case including Pareto, Zipf, Mandelbrot
laws). We will limit ourselves to the discrete case
(however, when the number of data is large enough
—which is our case — it is possible to work with the
continuous version of this law (Clauset et al., 2009).
For more detailed information of these laws the reader
can refer to (Clauset et al., 2009; Alstott et al., 2014)
we also used for statistical analysis.