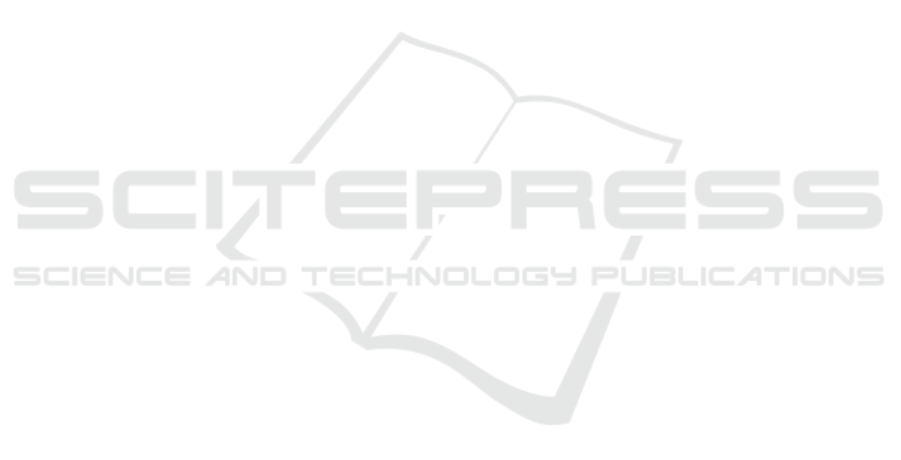
studies in all validation methods, so also the enhanced
features after applying the NCA. For arousal, feature
fusion did not work as good as for valence. How-
ever, applying the NCA enhanced them to work better
but not at all dimensionality. Results presented here
were the best results with the lowest dimensional-
ity. Overall, feature fusion of normalized HRV-based
and cvxEDA-based features together with feature en-
hancement using the NCA offered new baselines for
both valence and arousal in three validation methods.
Our results in arousal were only slightly above the
best ones from the previous studies based on LOO and
LOSO validation, and was similar to the one based on
10-fold cross validation. Using other feature extrac-
tion method is recommended to enhance the perfor-
mance in all validation methods and employing more
sophisticated classifier other than the simple kNN are
left for future works.
ACKNOWLEDGEMENTS
This research was supported by the Finnish Cultural
Foundation, Northern Ostrobothnia Regional Fund
2017.
REFERENCES
Ferdinando, H. and Alasaarela, E. (2017). Emotion recog-
nition using cvxEDA-based features. In Proceed-
ing of the 3rd International Conference on Electrical
Systems, Technology and Information (ICESTI) 2017.
MATEC Web of Conference.
Ferdinando, H., Sepp
¨
anen, T., and Alasaarela, E. (2016).
Comparing features from ECG pattern and HRV anal-
ysis for emotion recognition system. In 2016 IEEE
Conference on Computational Intelligence in Bioin-
formatics and Computational Biology, CIBCB 2016,
Chiang Mai, Thailand, October 5-7, 2016, pages 1–6.
Ferdinando, H., Sepp
¨
anen, T., and Alasaarela, E. (2017a).
Emotion recognition using neighborhood components
analysis and ecg/hrv-based features. In M. De Mar-
sico, G. S. di Baja, A. F., editor, Lecture Note on Com-
puter Science: Pattern Recognition Applications and
Methods. Springer Berlin Heidelberg, Berlin, Heidel-
berg. Accepted.
Ferdinando, H., Sepp
¨
anen, T., and Alasaarela, E. (2017b).
Enhancing emotion recognition from ECG signals us-
ing supervised dimensionality reduction. In Pro-
ceedings of the 6th International Conference on Pat-
tern Recognition Applications and Methods, ICPRAM
2017, Porto, Portugal, February 24-26, 2017., pages
112–118.
Goldberger, J., Hinton, G., Roweis, S., and Salakhutdinov,
R. (2005). Neighbourhood components analysis. In
Saul, L. K., Weiss, Y., and Bottou, L., editors, Ad-
vances in Neural Information Processing Systems 17,
pages 513–520. MIT Press.
Greco, A., Valenza, G., Lanata, A., Scilingo, E. P., and
Citi, L. (2016). cvxeda: A convex optimization ap-
proach to electrodermal activity processing. IEEE
Trans. Biomed. Engineering, 63(4):797–804.
Shu, Y. and Wang, S. (2017). Emotion recognition through
integrating eeg and peripheral signals. In Proceeding
of the IEEE International Conference on Acoustics,
Speech and Signal Processing (ICASSP). IEEE.
Soleymani, M., Lichtenauer, J., Pun, T., and Pantic, M.
(2012). A multimodal database for affect recognition
and implicit tagging. IEEE Trans. Affective Comput-
ing, 3(1):42–55.
Task Force of the European Society of Cardiology the
North American Society of Pacing Electrophysiology
(1996). Heart rate variability standards of measure-
ment, physiological interpretation, and clinical use.
Circulation, 93(5):1043–1065. HRV autonomic risk
factors.
Wiem, M. B. H. and Lachiri, Z. (2017). Emotion classi-
fication in arousal valence model using mahnob-hci
database. International Journal of Advanced Com-
puter Science and Applications, 8(3).
Zhu, Y., Wang, S., and Ji, Q. (2014). Emotion recogni-
tion from users’ eeg signals with the help of stimulus
videos. In Proceeding of IEEE International Confer-
ence on Multimedia and Expo (ICME). IEEE.
Enhancement of Emotion Recogniton using Feature Fusion and the Neighborhood Components Analysis
469