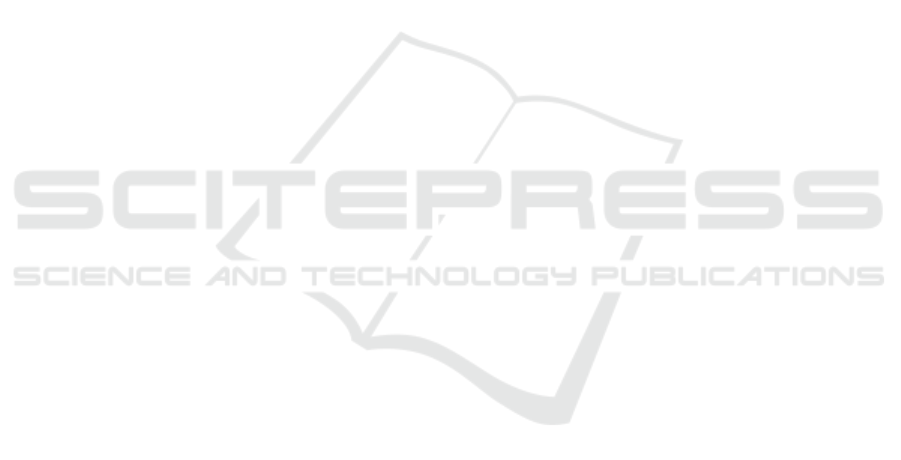
6 CONCLUSION
The dominant discrete theme of GGM obscures
the continuous convex properties of the multivariate
Gaussian distribution. Restricting inference to a par-
ticular graphical model obstructs accumulation of in-
formation describing the underlying distribution. For
Bayesian GGMs, uniform priors over the graphs re-
sults in extremely concentrated probability mass in
the natural parameters.
We support the use of GGMs for interpretation
and communication of approximate inference results
from multivariate Gaussian distributions. We strongly
discourage the use of GGMs directly for multivariate
Gaussian inference.
REFERENCES
Banerjee, O., Ghaoui, L. E., d’Aspremont, A., and Nat-
soulis, G. (2006). Convex optimization techniques for
fitting sparse gaussian graphical models. In Proceed-
ings of the 23rd international conference on Machine
learning, pages 89–96. ACM.
Bindel, D. (2009). Sherman-Morrison-Woodbury. Matrix
Computations (CS 6210), Cornell University lecture.
Bretthorst, G. (2012). The maximum entropy method of
moments and Bayesian probability theory. In 32nd
International Workshop on Bayesian Inference and
Maximum Entropy Methods in Science and Engineer-
ing, Carching, Germany, pages 3–15.
Celik, S., Logsdon, B., and Lee, S. (2014). Efficient di-
mensionality reduction for high-dimensional network
estimation. In Proceedings of the 31st International
Conference on Machine Learning (ICML-14), pages
1953–1961.
Dahl, J., Roychowdhury, V., and Vandenberghe, L. (2005).
Maximum likelihood estimation of Gaussian graphi-
cal models: numerical implementation and topology
selection. Technical report, Department of Electrical
Engineering, University of California, Los Angeles.
Dempster, A. (1969). Elements of continuous multivariate
analysis. Addison-Wesley.
Dempster, A. (1972). Covariance selection. Biometrics,
pages 157–175.
Dobra, A. and West, M. (2004). Bayesian covariance selec-
tion. Duke Statistics Discussion Papers, 23.
Fan, J., Feng, Y., and Wu, Y. (2009). Network exploration
via the adaptive lasso and scad penalties. The Annals
of Applied Statistics, 3(2):521.
Friedman, J., Hastie, T., and Tibshirani, R. (2008). Sparse
inverse covariance estimation with the graphical lasso.
Biostatistics, 9(3):432–441.
Gelman, A. (2004). Against parsimony. [Online; accessed
15-April-2015].
Gelman, A. (2011). David MacKay and Occam’s Razor.
[Online; accessed 15-April-2015].
Gelman, A. (2013). Flexibility is good. [Online; accessed
20-May-2015].
Giudici, P. and Green, P. (1999). Decomposable graph-
ical Gaussian model determination. Biometrika,
86(4):785–801.
Gonzalez, J. and Hong, S. (2008). Linear-time in-
verse covariance matrix estimation in Gaussian pro-
cesses. Technical report, Computer Science Depart-
ment, Carnegie Mellon University.
Good, I. J. (1963). Maximum entropy for hypothesis
formulation, especially for multidimensional contin-
gency tables. The Annals of Mathematical Statistics,
34(3):911–934.
Hoff, P. (2009). A first course in Bayesian statistical meth-
ods. Springer Science & Business Media.
Jalobeanu, A. and Guti
´
errez, J. (2007). Inverse covariance
simplification for efficient uncertainty management.
In 27th MaxEnt workshop, AIP Conference Proceed-
ings, Saratoga Springs, NY.
Jones, B., Carvalho, C., Dobra, A., Hans, C., Carter, C., and
West, M. (2005). Experiments in stochastic computa-
tion for high-dimensional graphical models. Statisti-
cal Science, 20(4):388–400.
Knuiman, M. (1978). Covariance selection. Advances in
Applied Probability, pages 123–130.
Lian, H. (2011). Shrinkage tuning parameter selection in
precision matrices estimation. Journal of Statistical
Planning and Inference, 141(8):2839–2848.
MacKay, D. (1992). Bayesian methods for adaptive models.
PhD thesis, California Institute of Technology.
Meinshausen, N. and B
¨
uhlmann, P. (2006). High-
dimensional graphs and variable selection with the
lasso. The Annals of Statistics, pages 1436–1462.
Moghaddam, B., Marlin, B., Khan, M., and Murphy, K.
(2009). Accelerating Bayesian structural inference
for non-decomposable Gaussian graphical models. In
NIPS.
Neal, R. (1996). Bayesian Learning for Neural Networks.
Springer.
Skilling, J. (2004). Nested sampling. Bayesian Inference
and Maximum Entropy Methods in Science and Engi-
neering, 735:395–405.
Tibshirani, R. (1996). Regression shrinkage and selection
via the lasso. Journal of the Royal Statistical Society.
Series B (Methodological), pages 267–288.
Wang, H., Reeson, C., and Carvalho, C. (2011). Dynamic
financial index models: Modeling conditional depen-
dencies via graphs. Bayesian Analysis, 6(4):639–664.
West, M. and Harrison, J. (1997). Bayesian forecasting and
dynamic models. Springer Verlag.
Whittaker, J. (1990). Graphical models in applied multi-
variate statistics. John Wiley & Sons Ltd.
Yuan, M. and Lin, Y. (2007). Model selection and esti-
mation in the Gaussian graphical model. Biometrika,
94(1):19–35.
Zhu, S. (1996). Statistical and computational theories
for image segmentation, texture modeling and object
recognition. PhD thesis, Harvard University.
The Wrong Tool for Inference - A Critical View of Gaussian Graphical Models
475