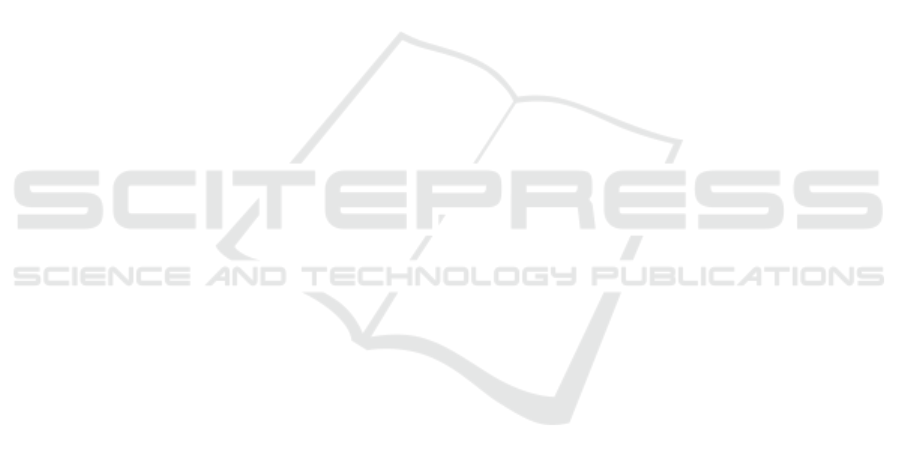
to have a high prestige in the network since the views
and ideas expressed by prestigious learners are
considered more important than other members. On
the other hand, the out-degree centrality is used to
measure learners’ influence in a network, which can
be measured by the number of posts of a learner
delivering to others, indicating that the learner’s
activity degree in engaging in a forum. As shown in
Table 3, there are significant positive correlations
among learners’ learning outcomes, prestige and
influence. In other words, the learners with high
academic performance tend to have a high prestige or
influence. The influential learners typically could
receive more replies. Additionally, the result also
shows that the learners participating in interactions
averagely gain the overall score of 87.81, and there is
a quite low average deviation among the learners’
scores (standard deviation is 2.3). As for those
learners who never participated in the forum (a total
of 5), their overall scores rank relatively backward
such as 26, 27, 73, 74 and 77, respectively, the
average score of which is less than learners who
participated in the forum.
5 CONCLUSIONS
This study utilizes the social network analysis to
investigate the evolution trends of network structure
of learners within a course forum in a university’
online learning platform, as well as further analyze
the correlation between individual position features
and learning outcomes in the forum. We could draw
the following conclusions:
Social network structure of learners would
dynamically vary as the progresses of course. In the
first three months of the course, the network density,
number of participants, number of posts and network
centrality all show gradual upgrading trends. That is,
the interactions among learners tend to be
increasingly frequent while links among them
become closer. However, in the last month, when the
course approaches the end of the semester, both the
numbers of participants and posts decrease, as well as
the sociogram also becomes relatively sparse.
Within interactions of the course forum, the
positions of learners in the network are partially
correlated to learning outcomes. Social network
centrality metrics have significantly positive
correlations with learning outcomes. The learners
with higher prestige or influence in social network
could typically gain higher learning outcomes. And
the factor that is most correlated to learning outcome
is degree centrality, followed by betweenness
centrality, the last one is closeness centrality. Finally,
learner’s prestige and influence in a forum are
significantly positively correlated to their learning
outcomes. This also indicates that the high-achieving
learners generally have the high prestige and
influence.
Therefore, if designed appropriately, discussion
activities may not only enhance the interactions
among learners, but also facilitate collaborative
inquiry learning and knowledge construction among
learners. To improve the activity levels of learners
among interactions, teachers may design some high-
quality interactive activities like inquiry-based
discussions, questions and answers, literature reviews
and knowledge brainstorms. Also, these activities
should be designed to be appropriate for knowledge
skills and interests of learners as well as have a certain
difficulty to drive learners to actively conduct
collaborative inquiries and discussions.
ACKNOWLEDGEMENTS
This work was supported by the Research Funds from
National Natural Science Foundation of China (Grant
No. 61702207), MOE (Ministry of Education in
China) Project of Humanities and Social Sciences
(Grant No. 16YJC880052), China Scholarship
Council (Grant No. 201706775022), National Social
Science Fund Project of China (Grant No.
14BGL131), Ministry of Education-China Mobile
(Grant No. MCM20160401).
REFERENCES
Aviv, R., Erlich, Z., Ravid, G., & Geva, A. (2003). Network
analysis of knowledge construction in asynchronous
learning networks. Journal of Asynchronous Learning
Network, 7(3): 1–23.
Baker-Eveleth, L. J. (2003). An online third place:
Emerging communities of practice. Ph.D. Dissertation.
United States: Washington State University.
Cela, K. L., Sicilia, M. Á., & Sánchez, S. (2015).
Comparison of collaboration and performance in
groups of learners assembled randomly or based on
learners’ topic preferences. Educational Technology &
Society, 18(4): 287–298.
Dowell, N. M., Graesser, A. C., Hennis, T. A., et al. (2015).
Modeling Learners’ Social Centrality and Performance
through Language and Discourse. In Proceedings of the
8th International Conference on Educational Data
Mining, pages 250-257. ERIC.
Jo, I. H., Kang, S., & Yoon, M. (2014). Effects of
Communication Competence and Social Network
CSEDU 2018 - 10th International Conference on Computer Supported Education
20