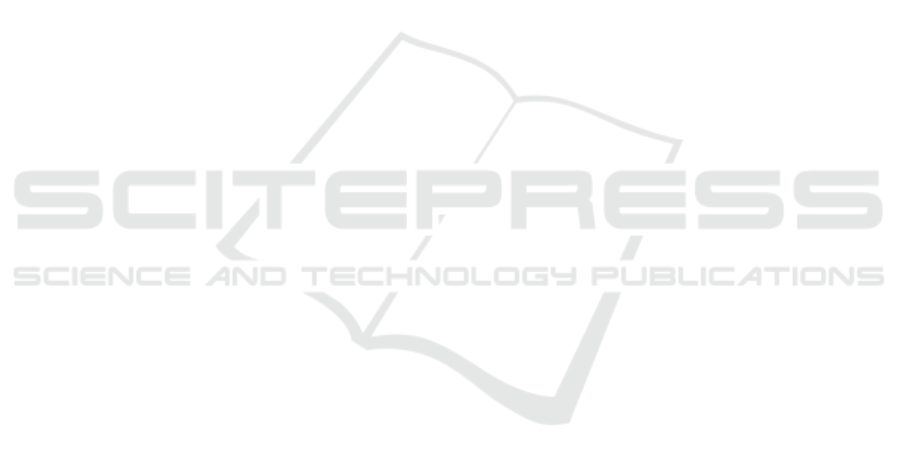
Several platforms have been developed to com-
pare agents’ strategic arguments, and there are strate-
gic argument competitions (Yuan et al., 2008). How-
ever, these do not include any perspectives on dishon-
est arguments.
Sakama formalized dishonesty using an argumen-
tation framework as a debate game (Sakama, 2012;
Sakama et al., 2015). Different from persuasion, they
judged the outcome of the dialogue by committed ar-
gumentation framework, thus each agent needs not es-
timate an opponent model. He also investigated some
properties of his model theoretically but did not for-
malize the detection of, or excuses for, deception.
6 CONCLUSIONS
We have presented the results of simulations of dis-
honest argumentation based on an opponent model.
This is the first attempt to present an evaluation of dis-
honest argumentation. The results show that the use
of dishonest arguments affects the chances of success-
fully persuading an opponent, or winning a debate
games. But we could not identify a relationship be-
tween the result of a dialogue and the argumentation
frameworks of agents.
As this is a preliminary report, only simple cases
are handled. In future, we should perform more ex-
periments on various types of argumentation frame-
works that include cyclic structures, and facilitate
more precise analysis. We will also investigate the
results under different semantics. since concepts re-
garding dishonesty depend on the semantics.
REFERENCES
Amgoud, L. and de Saint-Cyr, F. (2013). An axiomatic ap-
proach for persuasion dialogs. In ICTAI 2013, pages
618–625.
Amgoud, L., Maudet, N., and Parsons, S. (2000). Modeling
dialogues using argumentation. In ICMAS2000, pages
31–38.
Baroni, P., Caminada, M., and Giacomin, G. (2011). An
introduction to argumentation semantics. The Knowl-
edge Engineering Review, 26(4):365–410.
Bench-Capon, T. (2003). Persuasion in practice argument
using value-based argumentation frameworks. Jour-
nal of Logic and Computation, 13(3):429–448.
Black, E. and Hunter, A. (2015). Reasons and options for
updating an opponent model in persuasion dialogues.
In TAFA2015.
Dung, P. (1995). On the acceptability of arguments and
its fundamental role in nonmonotonic reasoning, logic
programming and n-person games. Artificial Intelli-
gence, 77(2):321–358.
Hadjinikolis, C., Siantos, Y., Modgil, S., Black, E., and
McBurney, P. (2013). Opponent modelling in persua-
sion dialogues. In IJCAI2013, pages 164–170.
Hunter, A. (2015). Modelling the persuadee in asymmet-
ric argumentation dialogues for persuasion. In IJ-
CAI2015, pages 3055–3061.
Parsons, S., Wooldridge, M., and Amgoud, L. (2003). On
the outcomes of formal inter-agent dialogues. In AA-
MAS2003, pages 616–623.
Prakken, H. (2006). Formal systems for persuasion
dialogue. The Knowledge Engineering Review,
21(2):163–188.
Prakken, H., Reed, C., and Walton, D. (2005). Dialogues
about the burden of proof. In ICAIL2005, pages 115–
124.
Rahwan, I., Lason, K., and Tohm’e, F. (2009). A character-
ization of strategy-proofness for grounded argumenta-
tion semantics. In IJCAI2009, pages 251–256.
Rahwan, I. and Simari, G. (2009). Argumentation in Artifi-
cial Intelligence. Springer.
Rienstra, T., Thimm, M., and Oren, N. (2013). Opponent
models with uncertainty for strategic argumentation.
In IJCAI2013, pages 332–338.
Sakama, C. (2012). Dishonest arguments in debate games.
In COMMA2012, pages 177–184.
Sakama, C., Caminada, M., and Herzig, A. (2015). A for-
mal account of dishonesty. The Logic Journal of the
IGPL, 23(2):259–294.
Takahashi, K. and Yokohama, S. (2017). On a formal
treatment of deception in argumentative dialogues. In
EUMAS-AT2016, Selected papers, pages 390–404.
Thimm, M. (2014). Strategic argumentation in multi-agent
systems. Kunstliche Intelligenz, 28(3):159–168.
Yokohama, S. and Takahashi, K. (2016). What should an
agent know not to fail in persuasion? In EUMAS-
AT2015, Selected papers, pages 219–233.
Yuan, T., Schulze, J., Devereux, J., and Reed, C. (2008).
Towards an arguing agents competition: Building on
argumento. In CMNA.
Evaluation of Dishonest Argumentation based on an Opponent Model: A Preliminary Report
275