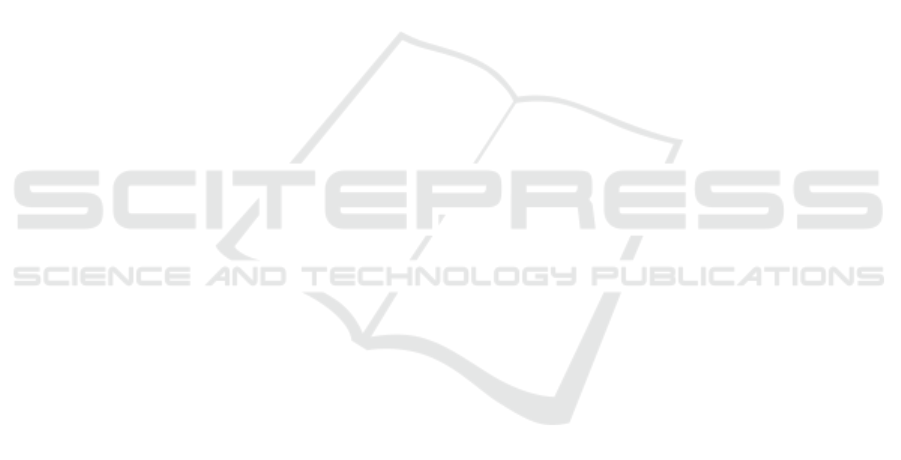
as a spatially distributed input signal into the implant
simulator. Edges and blobs are considered important
local features for object recognition and, thus, the net-
work seems to deduce appropriate features directly
from the global classification task. The results of the
classifier also indicate that the learned transformation
seems to be adequate for the task.
However, we do note that our approach has se-
vere limitations and restrictions regarding the techni-
cal side as well as in its expressiveness of the results.
Regarding the former, we made the implicit under-
lying assumption that the simulation of the percep-
tual image that is used in our virtual patient is cor-
rect and sufficient for further investigations. How-
ever, it is likely that the underlying processing of
pulse2percept, on which we base our estimation of the
PSF, will be subject to changes. Furthermore, we have
to stress, that the approximation of the signal process-
ing by using a PSF is restricting and does certainly not
capture all important steps in simulating a perceived
image. Moreover, the results presented indicate a sig-
nificant quality gain in an object classification task for
a virtual patient but, to this end, we cannot directly
implicate that this is also true for real patients.
4 CONCLUSION
We motivated the idea of bionic vision enhancement
w.r.t. subretinal implants in a virtual patient. We pro-
posed to model the signal processing from an input
image to a perceptual image as a neural network al-
lowing us to learn a suitable image transformation
for arbitrary differentiable objective functions. As a
proof of concept, we demonstrated the general ap-
plicability of our approach on an object classifica-
tion task, where a virtual patient is extended by an
artificial observing unit that decides on an object’s
class membership. Comparing our results to a base-
line model in which no image transformation is ap-
plied, the overall classification accuracy is increased
by 13.7% indicating great potential to enhance ob-
ject classification of visually impaired virtual patients.
Furthermore, since the virtual patient as well as the
image transformation are modelled in a neural net-
work, our approach is not limited to the visual task
of object classification but can be extended to other
objectives as well.
4.1 Limitations and Future Work
Our approach has limitations. First, since this is a pre-
liminary study on whether it is, in theory, possible to
enhance the perceived image of an impaired virtual
patient, we restricted ourselves to approximate the
processing of the perceived image by using a simple
PSF. This approximation certainly lacks details and is,
as already stated above, subject to a specific set of pa-
rameters regarding the implant and its actual position
inside the retina. However, every implant is likely to
be placed differently throughout the surgical implan-
tation and, thus, the perceived image will change w.r.t.
its placement. Moreover, there are different types and
stages of retinal diseases, so it is adequate so assume,
that the perceived image is likely to be different for
each treated patient.
Therefore, it is necessary to model the perceptual
simulation of pulse2percept and its underlying theo-
retical models (e.g., (Nanduri et al., 2012)) in greater
detail while maintaining differentiability for gradient
descent optimization as well as making them param-
eterizable for different kinds of implants, positions,
and so forth. Although, this task is very challenging,
the authors do believe, that this will promote further
efforts and advances in this research area.
Modelling the artificial observing unit as a classi-
fier refers to just one of many possible visual tasks.
Distance estimation or tracking of objects are exam-
ples of further tasks that may be of importance when
enhancing the input. Furthermore, we will research
possible dependencies of optimal input transforma-
tions for different recognition scenarios, such as the
ones listed above, to identify generally applicable po-
tential transformations for perception enhancement.
Finally, we cannot infer any direct proposition re-
garding the enhancement of real patient’s visual per-
ception. Therefore, extensive studies on real visu-
ally impaired and healthy subjects need to be done.
Specifically, w.r.t. this work it will be beneficial to
study, how healthy subjects perform on the object
classification task given the original and enhanced
perceptual images in order to see, whether the results
provided in this work are reasonable and usable for
real subjects.
REFERENCES
Beyeler, M., Boynton, G. M., Fine, I., and Rokem, A.
(2017). pulse2percept: A python-based simulation
framework for bionic vision. bioRxiv.
Busskamp, V., Duebel, J., Balya, D., Fradot, M., Viney,
T. J., Siegert, S., Groner, A. C., Cabuy, E., Forster,
V., Seeliger, M., Biel, M., Humphries, P., Paques,
M., Mohand-Said, S., Trono, D., Deisseroth, K.,
Sahel, J. A., Picaud, S., and Roska, B. (2010).
Genetic reactivation of cone photoreceptors restores
visual responses in retinitis pigmentosa. Science,
329(5990):413–417.
Perception Enhancement for Bionic Vision - Preliminary Study on Object Classification with Subretinal Implants
175