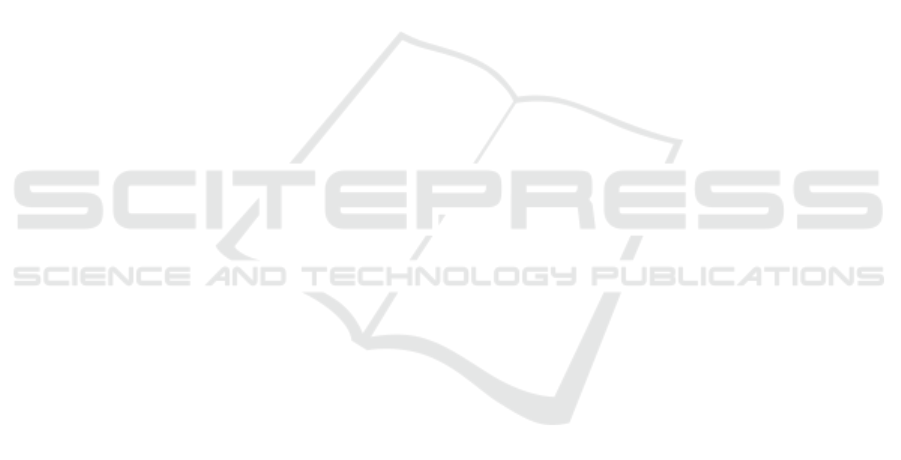
all cases. In our future research, we aim to further
increase the HCC automatic diagnosis accuracy by
employing the multi-resolution versions of the
CTMCM matrices and of the corresponding
Haralick features. We will also consider larger
datasets in order to improve the validation procedure
and deep learning methods in order to increase the
classification performance. We take into account the
possibility of using other types of ultrasound images,
as well, such as contrast enhanced ultrasound images
(CEUS), respectively elastographic images.
REFERENCES
American liver foundation, 2015. Online: http://www.
liverfoundation.org/abouttheliver/info/
Sherman, M. 2005. Approaches to the Diagnosis of
Hepatocellular Carcinoma. In Current
Gastroenterology Reports, vol. 7, no. 1, pp.11-18.
Sujana, H., Swarnamani, S., 1996. Application of
Artificial Neural Networks for the classification of
liver lesions by texture parameters. In Ultrasound in
Medicine & Biology, vol. 22, no. 9, pp.1177 –1181.
Yoshida, H., Casalino, D., 2003. Wavelet packet based
texture analysis for diferentiation between benign and
malignant liver tumors in ultrasound images. In
Physics in Medicine & Biology, vol. 48, pp. 3735-
3753.
Madabhushi, A., 2005. Automated Detection of Prostatic
Adenocarcinoma from High-Resolution Ex Vivo MRI.
In IEEE Transactions on Medical Imaging, pp.1611-
1626.
Chikui, T., 2005. Sonographic texture characterization of
salivary gland tumors by fractal analysis, In
Ultrasound in Medicine & Biology, vol. 31, no. 10,
pp.1297- 1304.
Duda, D., Kretowski, M., 2013. Computer-Aided
Diagnosis of Liver Tumors Based on Multi-Image
Texture Analysis of Contrast-Enhanced CT. Selection
of the Most Appropriate Texture Features. In Studies
in Logic, Grammar and Rhetoric, vol. 35, no. 1, pp.
49–70.
Khuzir, A., Besar, R., 2009. Identification of masses in
digital mammogram using gray level co-occurrence
matrices. In Biomedical Imaging Intervention Journal,
vol. 5, no. 3, pp. e17-e30.
Masood, K., 2006. Co-occurrence and morphological
analysis for colon tissue biopsy classification. In
Proceedings of the 4th International Workshop on
Information Technology, 2006, Pakistan, pp. 211-216.
Meyer-Base, A., 2009. Pattern recognition for medical
imaging, Elsevier, San Diego, California.
Mitrea, D., Mitrea, P., Nedevcshi, S., Lupsor, M.,
Socaciu, M, Golea, A., Hagiu, C., Ciobanu, L., 2012.
Abdominal tumor characterization and recognition
using superior order co-occurrence matrices, based on
ultrasound images. In Computational and
Mathematical Methods in Medicine, 17 pages.
Mitrea, D., Nedevcshi, S., Socaciu, M., Badea, R., 2012.
The Role of the Superior Order GLCM in the
Characterization and Recognition of the Liver Tumors
from Ultrasound Images. In Radioengineering, vol.
21, no. 1, pp. 79-85.
Mitrea, D., Nedevschi, S., Badea, R., 2014. The Role of
the Textural Microstructure Co-occurrence Matrices
in the Classification of the Abdominal Tumors. The
10th IEEE ICCP 2014 Conference, Technical
University of Cluj-Napoca , pp. 187-190.
Mitrea, D., Nedevschi, S., Abrudean, M., Badea, R., 2015.
Colorectal cancer recognition from ultrasound images,
using complex textural microstructure co-occurrence
matrices, based on Laws’ features, In proccedings of
the 38
th
IEEE International Conference on
Telecommunications and Signal Processing, 2015
(TSP 2015), Praga, pp. 458-462.
Mitrea, D., Nedevschi, S, Badea, R., 2016. The Role of the
Complex Extended Textural Microstructure Co-
occurrence Matrix in the Unsupervised Detection of
the HCC Evolution Phases, based on Ultrasound
Images. In Proceedings of International Conference on
Pattern Recognition Applications and Methods
(ICPRAM 2016), Roma, pp. 698-705.
Davis, L.S., Clearman, M., Aggarwal, J., 1979. A
Comparative Texture Classification Study Based on
Generalized Cooccurence Matrix. Proceedings of the
18-th IEEE Conference on Decision and Control, pp.
71-78.
Sujatha, B., Kumar, V., Harini, P., 2012. A new logical
compact LBP Co-Occurrence Matrix for Texture
Analysis. In International Journal of Scientific &
Engineering Research, vol. 3, no. 2, pp. 1-5, 2012.
Sujatha, B., Sekhar Reddi, C., 2013. Texture classification
using texton co-occurrence matrix derived from
texture orientation. In International J. Soft Computing
and Eng, vol. 2, no. 6, pp. 18-23.
Laws, K.I., 1980. Rapid texture identification. In SPIE
Vol. 238 Image Processing for Missile Guidance,
pp.76-380.
Nanni, L., Brahnam, S., Ghidoni, S., Menegatti, E.,
Barrier, T., 2013. “Different Approaches for
Extracting Information from the Co-Occurrence
Matrix”. In PloS One Journal, Vol.8, No. 12, 2013, pp.
1-9.
Kirsch, R., 1971. Computer determination of the
constituent structure of biological images. In
Computers and Biomedical Research, Vol.4, No.3, pp.
315–328.
Hall, M, 2003. Benchmarking attribute selection
techniques for discrete class data mining. In IEEE
Trans On Knowledge and Data Eng., vol. 15, no. 3,
pp. 1-16.
Weka3, Data Mining Software in Java, 2017. Online:
http://www.cs.waikato.ac.nz/ml/weka.
Duda, R., 2003 Pattern Classification, Wiley Interscience,
Hoboken, New Jersey.
Automatic Recognition of the Hepatocellular Carcinoma from Ultrasound Images using Complex Textural Microstructure Co-Occurrence
Matrices (CTMCM)
189