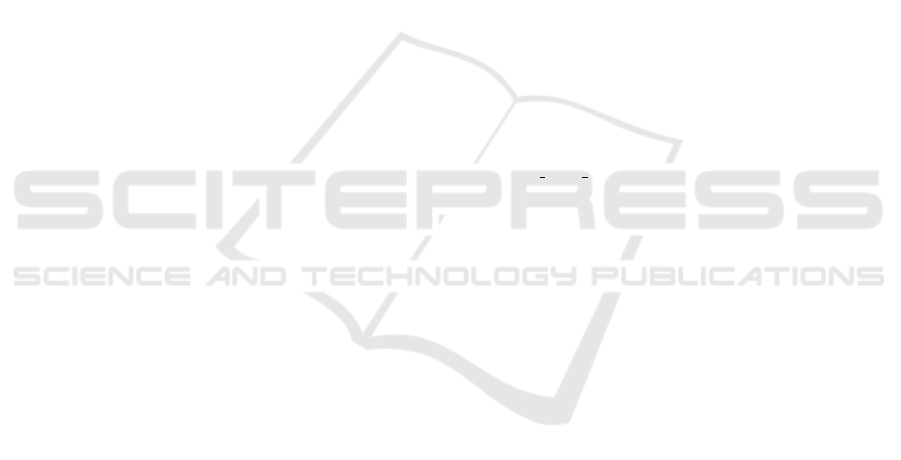
using CT: preliminary results and the effect of RMP-
7. Quantitative imaging in oncology, 1:1–5.
Fournier, L. S., Oudard, S., Thiam, R., Trinquart, L., Banu,
E., Medioni, J., Balvay, D., Chatellier, G., Frija, G.,
and Cuenod, C. A. (2010). Metastatic renal carci-
noma: Evaluation of antiangiogenic therapy with dy-
namic contrast-enhanced CT. Radiology, 256(2):511–
518.
Hachama, M., Desolneux, A., Cuenod, C. A., and Richard,
F. J. (2010). A classifying registration technique for
the estimation of enhancement curves of DCE-CT
scan sequences. Medical Image Analysis, 14(2):185
– 194.
Hill, D. L. G., Batchelor, P. G., Holden, M., and Hawkes,
D. J. (2001). Medical image registration. Physics in
Medicine and Biology, 46(3):R1.
Hou, Z., Wang, Y., Thng, C. H., Ng, Q.-S., Goh, V.,
and Koh, T. S. (2014). Automatic region-of-interest
segmentation and registration of dynamic contrast-
enhanced images of colorectal tumors. Physics in
Medicine and Biology, 59(23):7361.
Jia, Z., Huang, X., Chang, E. I. C., and Xu, Y. (2017).
Constrained deep weak supervision for histopathol-
ogy image segmentation. IEEE Transactions on Med-
ical Imaging, 36(11):2376–2388.
Kawulok, M., Kawulok, J., and Nalepa, J. (2014a).
Spatial-based skin detection using discriminative
skin-presence features. Pattern Recognition Letters,
41:3 – 13.
Kawulok, M., Kawulok, J., Nalepa, J., and Smołka, B.
(2014b). Self-adaptive algorithm for segmenting skin
regions. EURASIP Journal on Advances in Signal
Processing, 2014(1):170.
Liskowski, P. and Krawiec, K. (2016). Segmenting retinal
blood vessels with deep neural networks. IEEE Trans-
actions on Medical Imaging, 35(11):2369–2380.
Lorenzo, P. R., Nalepa, J., Kawulok, M., Ramos, L. S., and
Pastor, J. R. (2017). Particle swarm optimization for
hyper-parameter selection in deep neural networks. In
Proceedings of the Genetic and Evolutionary Compu-
tation Conference, GECCO ’17, pages 481–488, New
York, NY, USA. ACM.
Martin, S., Johnson, C., Brophy, M., Palma, D. A., Bar-
ron, J. L., Beauchemin, S. S., Louie, A. V., Yu,
E., Yaremko, B., Ahmad, B., Rodrigues, G. B., and
Gaede, S. (2015). Impact of target volume segmen-
tation accuracy and variability on treatment planning
for 4d-ct-based non-small cell lung cancer radiother-
apy. Acta Oncologica, 54(3):322–332.
Miles, K. A. (2002). Functional computed tomography in
oncology. European Journal of Cancer, 38(16):2079
– 2084.
Miles, K. A., Hayball, M. P., and Dixon, A. K. (1993).
Functional images of hepatic perfusion obtained with
dynamic CT. Radiology, 188(2):405–411.
Miles, K. A., Lee, T.-Y., Goh, V., Klotz, E., Cuenod, C.,
Bisdas, S., Groves, A. M., Hayball, M. P., Alonzi,
R., and Brunner, T. (2012). Current status and guide-
lines for the assessment of tumour vascular support
with dynamic contrast-enhanced computed tomogra-
phy. European Radiology, 22(7):1430–1441.
Nalepa, J. and Błocho, M. (2015). Co-operation in the par-
allel memetic algorithm. International Journal of Par-
allel Programming, 43(5):812–839.
Nalepa, J. and Blocho, M. (2016). Adaptive memetic al-
gorithm for minimizing distance in the vehicle rout-
ing problem with time windows. Soft Computing,
20(6):2309–2327.
Nalepa, J. and Kawulok, M. (2014). A memetic algorithm
to select training data for support vector machines. In
Proc. of the 2014 Annual Conference on Genetic and
Evolutionary Computation, GECCO ’14, pages 573–
580, New York, USA. ACM.
Nalepa, J. and Kawulok, M. (2016). Adaptive memetic
algorithm enhanced with data geometry analysis to
select training data for SVMs. Neurocomputing,
185:113 – 132.
Ng, Q.-S., Goh, V., Carnell, D., Meer, K., Padhani, A. R.,
Saunders, M. I., and Hoskin, P. J. (2007). Tumor
antivascular effects of radiotherapy combined with
combretastatin A4 phosphate in human non-small-cell
lung cancer. International Journal of Radiation On-
cology, 67(5):1375 – 1380.
O’Connor, J. P. B., Tofts, P. S., Miles, K. A., Parkes,
L. M., Thompson, G., and Jackson, A. (2011). Dy-
namic contrast-enhanced imaging techniques: Ct and
mri. The British Journal of Radiology, 84(spe-
cial issue 2):S112–S120. PMID: 22433822.
Oliveira, F. P. and Tavares, J. M. R. (2014). Medical image
registration: a review. Computer Methods in Biome-
chanics and Biomedical Engineering, 17(2):73–93.
PMID: 22435355.
Smistad, E., Falch, T. L., Bozorgi, M., Elster, A. C., and
Lindseth, F. (2015). Medical image segmentation on
GPUs a comprehensive review. Medical Image Anal-
ysis, 20(1):1 – 18.
Vitulano, S., Ruberto, C. D., and Nappi, M. (1997). Differ-
ent methods to segment biomedical images. Pattern
Recognition Letters, 18(11):1125 – 1131.
Walczak, M., Burda, I., Nalepa, J., and Kawulok, M.
(2017). Segmenting lungs from whole-body ct
scans. In Kozielski, S., Mrozek, D., Kasprowski,
P., Małysiak-Mrozek, B., and Kostrzewa, D., edi-
tors, Beyond Databases, Architectures and Structures.
Towards Efficient Solutions for Data Analysis and
Knowledge Representation: 13th International Con-
ference, BDAS 2017, Ustro
´
n, Poland, May 30 - June 2,
2017, Proceedings, pages 403–414, Cham. Springer
International Publishing.
Wei, J. and Li, G. (2014). Automated lung segmenta-
tion and image quality assessment for clinical 3-d/4-d-
computed tomography. IEEE Journal of Translational
Engineering in Health and Medicine, 2:1–10.
Extracting Biomarkers from Dynamic Images - Approaches and Challenges
525